Machine learning regression algorithms to predict emissions from steam boilers
Heliyon(2024)
摘要
Currently, the modeling of complex chemical-physical processes is drastically influencing industrial development. Therefore, the analysis and study of the combustion process of the boilers using machine learning (ML) techniques are vital to increase the efficiency with which this equipment operates and reduce the pollution load they contribute to the environment. This work aims to predict the emissions of CO, CO2, NOx, and the temperature of the exhaust gases of industrial boilers from real data. Different ML algorithms for regression analysis are discussed. The following are input variables: ambient temperature, working pressure, steam production, and the type of fuel used in around 20 industrial boilers. Each boiler's emission data was collected using a TESTO 350 Combustion Gas Analyzer. The modeling, with a machine learning approach using the Gradient Boosting Regression algorithm, showed better performance in the predictions made on the test data, outperforming all other models studied. It was achieved with predicted values showing a mean absolute error of 0.51 and a coefficient of determination of 99.80%. Different regression models (DNN, MLR, RFR, GBR) were compared to select the most optimal. Compared to models based on Linear Regression, the DNN model has better prediction performance. The proposed model provides a new method to predict CO2, CO, NOx emissions, and exhaust gas outlet temperature.
更多查看译文
关键词
Linear regression,Python,Artificial intelligence,Combustion
AI 理解论文
溯源树
样例
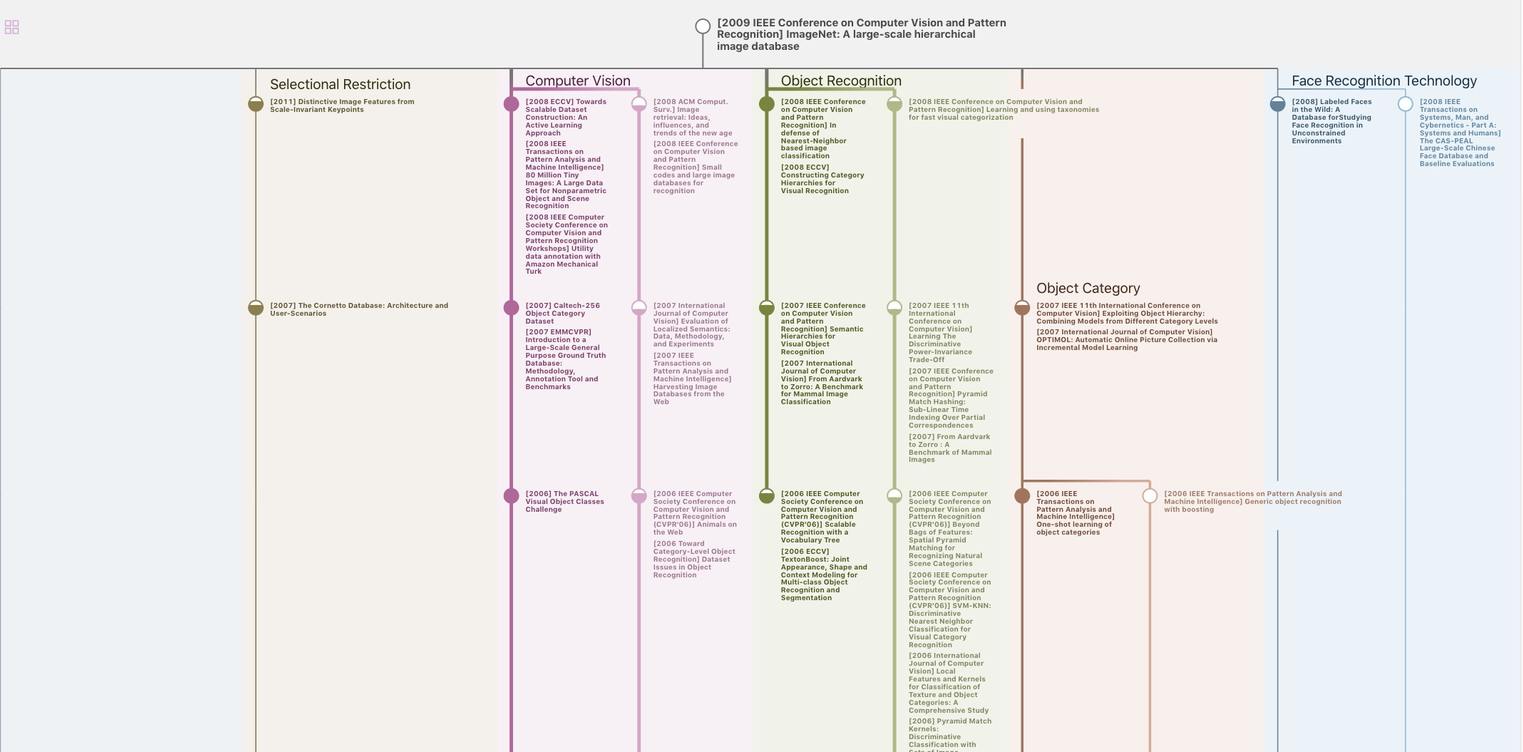
生成溯源树,研究论文发展脉络
Chat Paper
正在生成论文摘要