BSPA: Exploring Black-box Stealthy Prompt Attacks against Image Generators
CoRR(2024)
摘要
Extremely large image generators offer significant transformative potential
across diverse sectors. It allows users to design specific prompts to generate
realistic images through some black-box APIs. However, some studies reveal that
image generators are notably susceptible to attacks and generate Not Suitable
For Work (NSFW) contents by manually designed toxin texts, especially
imperceptible to human observers. We urgently need a multitude of universal and
transferable prompts to improve the safety of image generators, especially
black-box-released APIs. Nevertheless, they are constrained by labor-intensive
design processes and heavily reliant on the quality of the given instructions.
To achieve this, we introduce a black-box stealthy prompt attack (BSPA) that
adopts a retriever to simulate attacks from API users. It can effectively
harness filter scores to tune the retrieval space of sensitive words for
matching the input prompts, thereby crafting stealthy prompts tailored for
image generators. Significantly, this approach is model-agnostic and requires
no internal access to the model's features, ensuring its applicability to a
wide range of image generators. Building on BSPA, we have constructed an
automated prompt tool and a comprehensive prompt attack dataset (NSFWeval).
Extensive experiments demonstrate that BSPA effectively explores the security
vulnerabilities in a variety of state-of-the-art available black-box models,
including Stable Diffusion XL, Midjourney, and DALL-E 2/3. Furthermore, we
develop a resilient text filter and offer targeted recommendations to ensure
the security of image generators against prompt attacks in the future.
更多查看译文
AI 理解论文
溯源树
样例
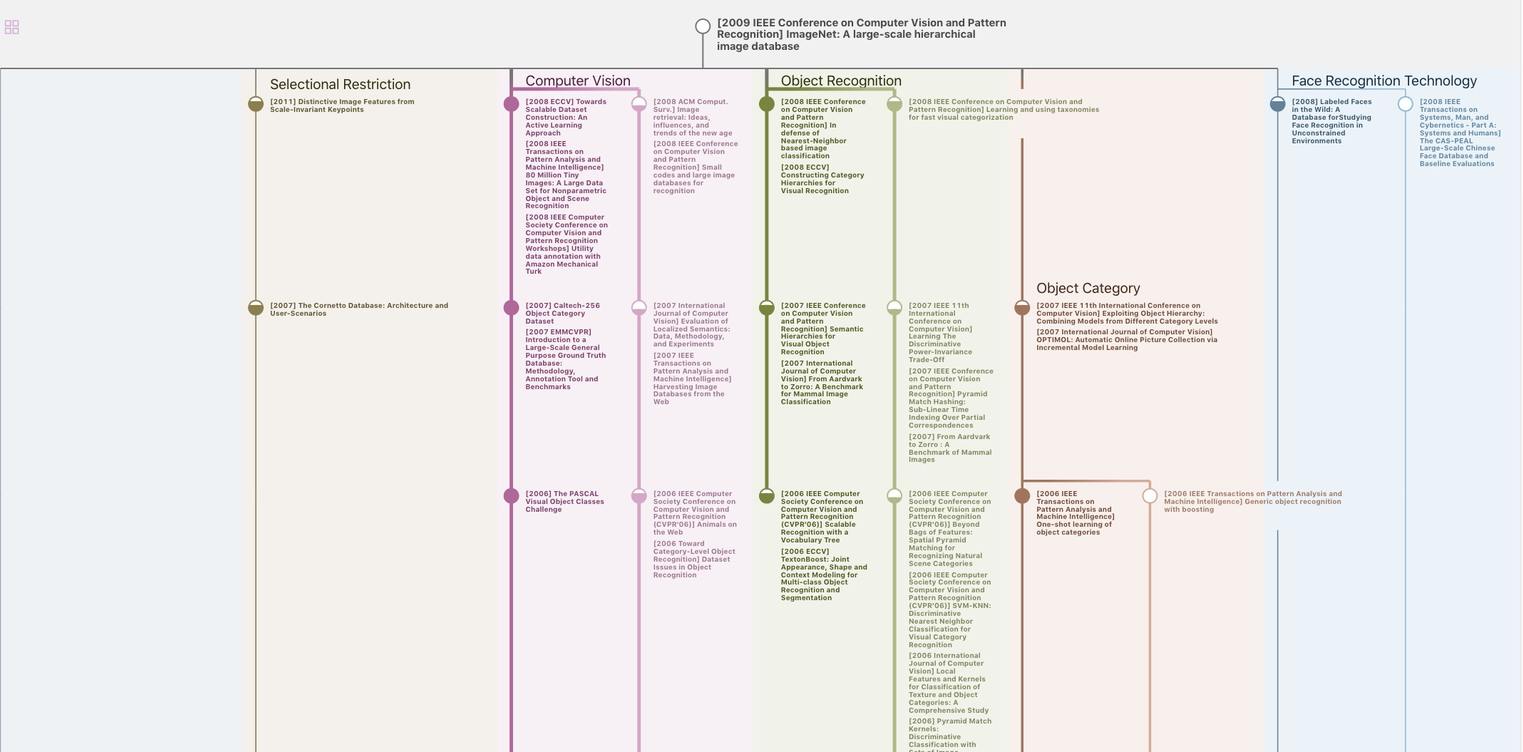
生成溯源树,研究论文发展脉络
Chat Paper
正在生成论文摘要