Estimating Above-Ground Biomass of the Regional Forest Landscape of Northern Western Ghats Using Machine Learning Algorithms and Multi-sensor Remote Sensing Data
Journal of the Indian Society of Remote Sensing(2024)
摘要
Estimating above-ground biomass (AGB) using machine learning (ML) algorithms and multi-sensor satellite data is a promising approach for monitoring and managing forest resources. This research integrated synthetic aperture radar (SAR) and multispectral imagery alongside in-field observations to accurately estimate above-ground biomass (AGB) in the Purna regional landscape of northern Western Ghats, India. The satellite data employed in the study included dual-polarization (VV + VH) imagery from Sentinel-1 and multi-spectral bands from Sentinel-2, processed and analysed using advanced ML algorithms. The ML algorithms, namely Random Forest (RF), Extreme Gradient Boosting (XGB), and Boosted Regression Trees (BRT), were strategically applied across different model scenarios to determine their effectiveness in AGB prediction. The XGB model displayed the highest accuracy with an R2 value of 0.61 and the lowest RMSE of 37.85 t/ha. The spatial distribution of AGB was successfully mapped, showing varied biomass concentrations throughout the study area. The study’s findings demonstrate the potential of integrating SAR and multispectral data for enhanced AGB estimation and suggest that ML models, specifically algorithms like RF, XGB, and BRT can address the complex relationships between AGB and satellite-derived variables more effectively than traditional methods.
更多查看译文
关键词
Biomass,Remote sensing,Synthetic aperture radar,Multispectral,Machine learning
AI 理解论文
溯源树
样例
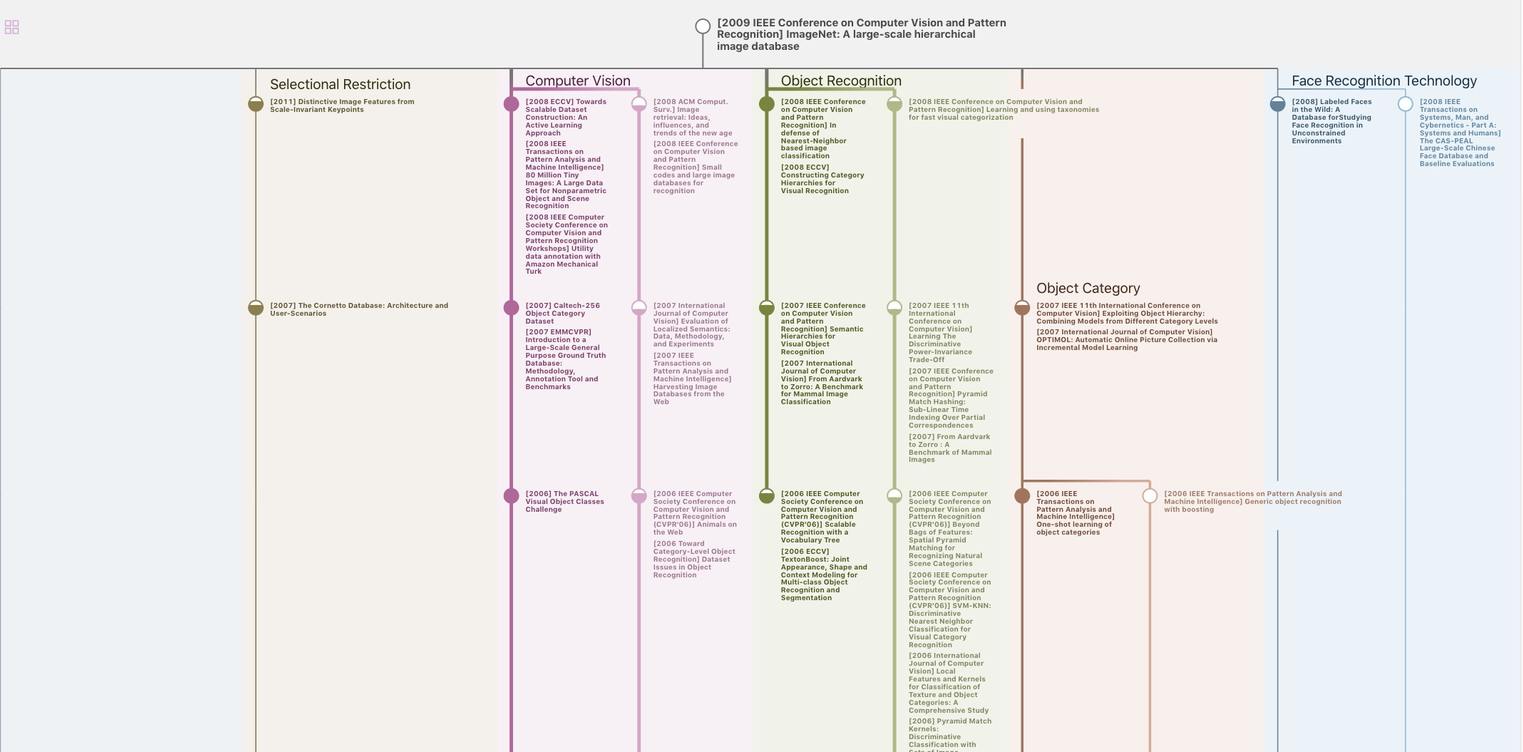
生成溯源树,研究论文发展脉络
Chat Paper
正在生成论文摘要