Enhancing sine cosine algorithm based on social learning and elite opposition-based learning
Computing(2024)
摘要
In recent years, Sine Cosine Algorithm (SCA) is a kind of meta-heuristic optimization algorithm with simple structure, simple parameters and trigonometric function principle. It has been proved that it has good competitiveness among the existing optimization algorithms. However, the single mechanism of SCA leads to its insufficient utilization of the information of the whole population, insufficient ability to jump out of local optima and poor performance at solving complex objective function. Therefore, this paper introduces social learning strategy (SL) and elite opposition-based learning (EOBL) strategy to improve SCA, and proposes novel algorithm: enhancing Sine Cosine Algorithm based on elite opposition-based learning and social learning (ESLSCA). Social learning strategy takes full advantage of information from the entire population. The elite opposition-based learning strategy provides a possibility for the algorithm to jump out of local optima and increases the diversity of the population. To demonstrate the performance of ESLSCA, this paper uses 22 well-known benchmark test functions and CEC2019 test function set to evaluate ESLSCA. The comparisons show that the proposed ESLSCA has better performance than the standard SCA and it is very competitive among other excellent optimization algorithms.
更多查看译文
关键词
Global optimization,Sine cosine algorithm,Meta-heuristic algorithm,Social learning,Elite opposition-based learning,65K10
AI 理解论文
溯源树
样例
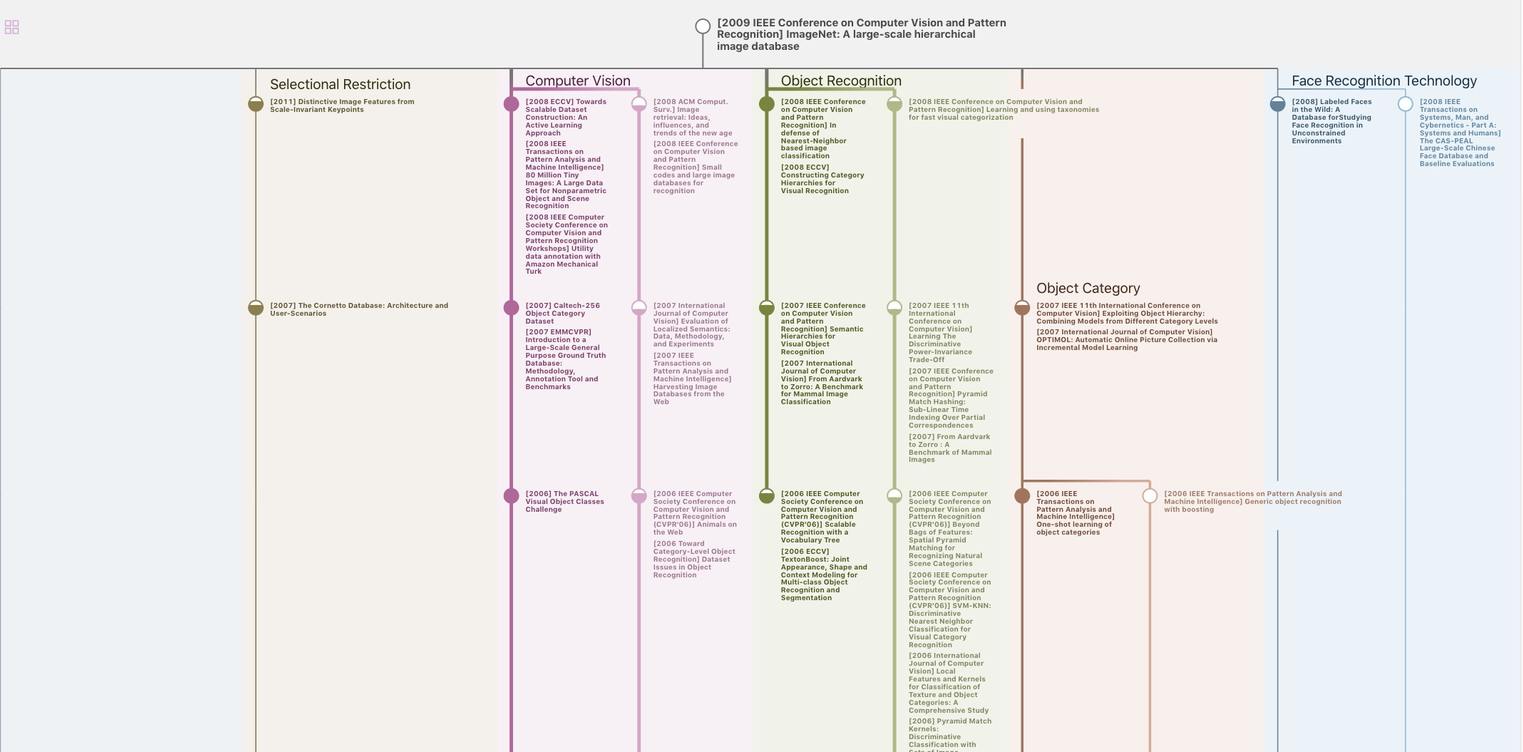
生成溯源树,研究论文发展脉络
Chat Paper
正在生成论文摘要