Generalizable Clinical Note Section Identification with Large Language Models
medrxiv(2024)
摘要
Objective Clinical note section identification helps locate relevant information and could be beneficial for downstream tasks such as named entity recognition. But the traditional supervised methods suffer from transferability issues. This study proposes a new framework for using large language models for section identification to overcome the limitations.
Materials and methods We framed section identification as question-answering and provided the section definitions in free-text. We evaluated multiple LLMs off-the-shelf without any training. We also fine- tune our LLMs to investigate how the size and the specificity of the fine-tuning dataset impacts model performance.
Results GPT4 achieved the highest F1 score of 0.77. The best open-source model (Tulu2-70b) achieved 0.64 and is on par with GPT3.5 (ChatGPT). GPT4 is also found to obtain F1 scores greater than 0.9 for 9 out of the 27 (33%) section types and greater than 0.8 for 15 out of 27 (56%) section types. For our fine-tuned models, we found they plateaued with an increasing size of the general domain dataset. We also found that adding a reasonable amount of section identification examples is beneficial.
Discussion These results indicate that GPT4 is nearly production-ready for section identification, and seemingly contains both knowledge of note structure and the ability to follow complex instructions, and the best current open-source LLM is catching up.
Conclusion Our study shows that LLMs are promising for generalizable clinical note section identification. They have the potential to be further improved by adding section identification examples to the fine-tuning dataset.
### Competing Interest Statement
The authors have declared no competing interest.
### Funding Statement
This work was supported by the National Institutes of Health under Award Number R01LM012973, R01MH126977, R01HL151604, and R01LM013486. The content is solely the responsibility of the authors and does not necessarily represent the official views of the National Institutes of Health.
### Author Declarations
I confirm all relevant ethical guidelines have been followed, and any necessary IRB and/or ethics committee approvals have been obtained.
Yes
The details of the IRB/oversight body that provided approval or exemption for the research described are given below:
The discharge dataset notes are available at , and the section annotations can be found at [https://github.com/2533245542/SectionChunker\_i2b2\_2010_data][1]. The progress dataset is available at . The ORCA dataset is available at .
I confirm that all necessary patient/participant consent has been obtained and the appropriate institutional forms have been archived, and that any patient/participant/sample identifiers included were not known to anyone (e.g., hospital staff, patients or participants themselves) outside the research group so cannot be used to identify individuals.
Yes
I understand that all clinical trials and any other prospective interventional studies must be registered with an ICMJE-approved registry, such as ClinicalTrials.gov. I confirm that any such study reported in the manuscript has been registered and the trial registration ID is provided (note: if posting a prospective study registered retrospectively, please provide a statement in the trial ID field explaining why the study was not registered in advance).
Yes
I have followed all appropriate research reporting guidelines, such as any relevant EQUATOR Network research reporting checklist(s) and other pertinent material, if applicable.
Yes
The discharge dataset notes are available at , and the section annotations can be found at [https://github.com/2533245542/SectionChunker\_i2b2\_2010_data][1]. The progress dataset is available at . The ORCA dataset is available at .
[1]: https://github.com/2533245542/SectionChunker_i2b2_2010_data
更多查看译文
AI 理解论文
溯源树
样例
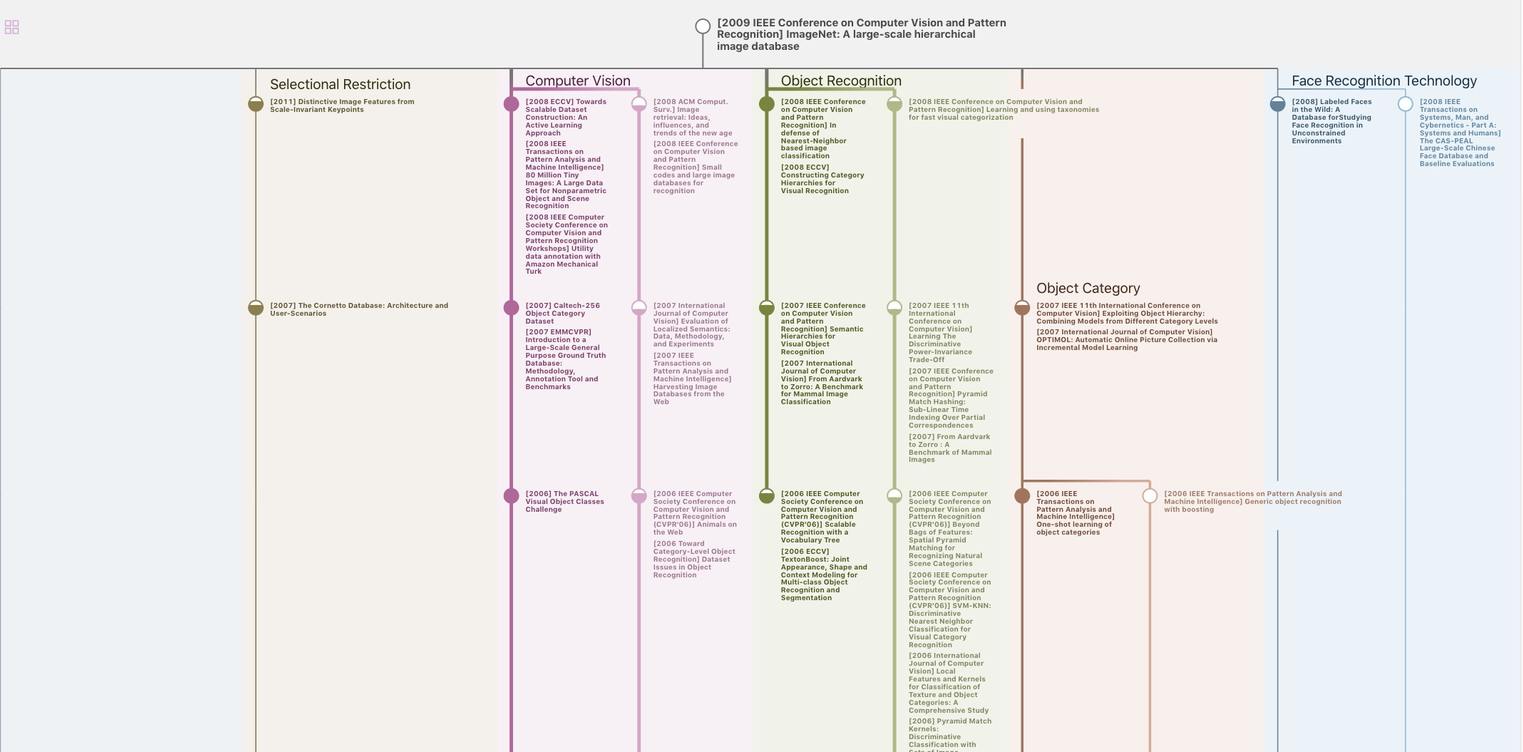
生成溯源树,研究论文发展脉络
Chat Paper
正在生成论文摘要