Development of a differential treatment selection model for depression on consolidated and transformed clinical trial datasets
medrxiv(2024)
摘要
Objective Major depressive disorder (MDD) is the leading cause of disability worldwide, yet treatment selection still proceeds via “trial and error”. Given the varied presentation of MDD and heterogeneity of treatment response, the use of machine learning to understand complex, non-linear relationships in data may be key for treatment personalization. Well-organized, structured data from clinical trials with standardized outcome measures is useful for training machine learning models; however, combining data across trials poses numerous challenges. There is also persistent concern that machine learning models can propagate harmful biases.
Methods We have created a methodology for organizing and preprocessing depression clinical trial data such that transformed variables harmonized across disparate datasets can be used as input for feature selection. Using bayesian optimization, we identified an optimal multi-layer dense neural network which used data from 21 clinical and sociodemographic features as input in order to perform differential treatment benefit prediction.
Results With this combined dataset of 5032 individuals and 6 drugs, we created a differential treatment benefit prediction model. Our model generalized well to the held-out test set and produced similar accuracy metrics in the test and validation set with an AUC of 0.7 when predicting binary remission. To address the potential for bias propagation, we used a bias testing performance metric to evaluate the model for harmful biases related to ethnicity, age, or sex.
Conclusion We present a full pipeline from data preprocessing to model validation that was employed to create the first differential treatment benefit prediction model for MDD containing 6 treatment options.
### Competing Interest Statement
KP, JM, DB, CA, RF, CP, JFT, JW, CR, GG are current or former shareholders, option holders, employees and/or officers of Aifred Health. GT has no competing interests.
### Funding Statement
This work was supported by a grant from ERA-Permed Vision 2020 supporting IMADAPT.
### Author Declarations
I confirm all relevant ethical guidelines have been followed, and any necessary IRB and/or ethics committee approvals have been obtained.
Yes
The details of the IRB/oversight body that provided approval or exemption for the research described are given below:
The ethics committee/IRB of the Douglas Mental Health University Institute gave ethical approval for this work. A data sharing agreement was signed with GSK/Eli Lilly to obtain access to de-identified data.
I confirm that all necessary patient/participant consent has been obtained and the appropriate institutional forms have been archived, and that any patient/participant/sample identifiers included were not known to anyone (e.g., hospital staff, patients or participants themselves) outside the research group so cannot be used to identify individuals.
Yes
I understand that all clinical trials and any other prospective interventional studies must be registered with an ICMJE-approved registry, such as ClinicalTrials.gov. I confirm that any such study reported in the manuscript has been registered and the trial registration ID is provided (note: if posting a prospective study registered retrospectively, please provide a statement in the trial ID field explaining why the study was not registered in advance).
Yes
I have followed all appropriate research reporting guidelines, such as any relevant EQUATOR Network research reporting checklist(s) and other pertinent material, if applicable.
Yes
All GlaxoSmithKline and Eli Lilly data was obtained via the Clinical Study Data Request (CSDR) platform - we do not own any of the clinical trial data. Studies with the following IDs were utilized in this paper: gsk\_29060\_128, gsk\_29060\_115, US-HMCR, gsk\_ak130939, gsk-WXL101497, gsk\_29060\_874, lilly\_F1J-MC-HMCQ, lily\_F1J-AA-HMCV, gsk\_29060\_810, gsk\_AK1113351, lilly\_FJ1-MC-HMAYa, lilly\_FJ1-MC-HMAYb, lilly\_FJ1-MC-HMATA, lilly\_FJ1-MC-HMATb, lilly\_FIJ-MC-HMAQb, lilly\_F1J-MC-HMBV, lilly_FJ1-MC-HMAQa. All information about the model, including model weights and scaling factors can be found at the following github link: [https://github.com/Aifred-Health/pharma\_research\_model][1]
[1]: https://github.com/Aifred-Health/pharma_research_model
更多查看译文
AI 理解论文
溯源树
样例
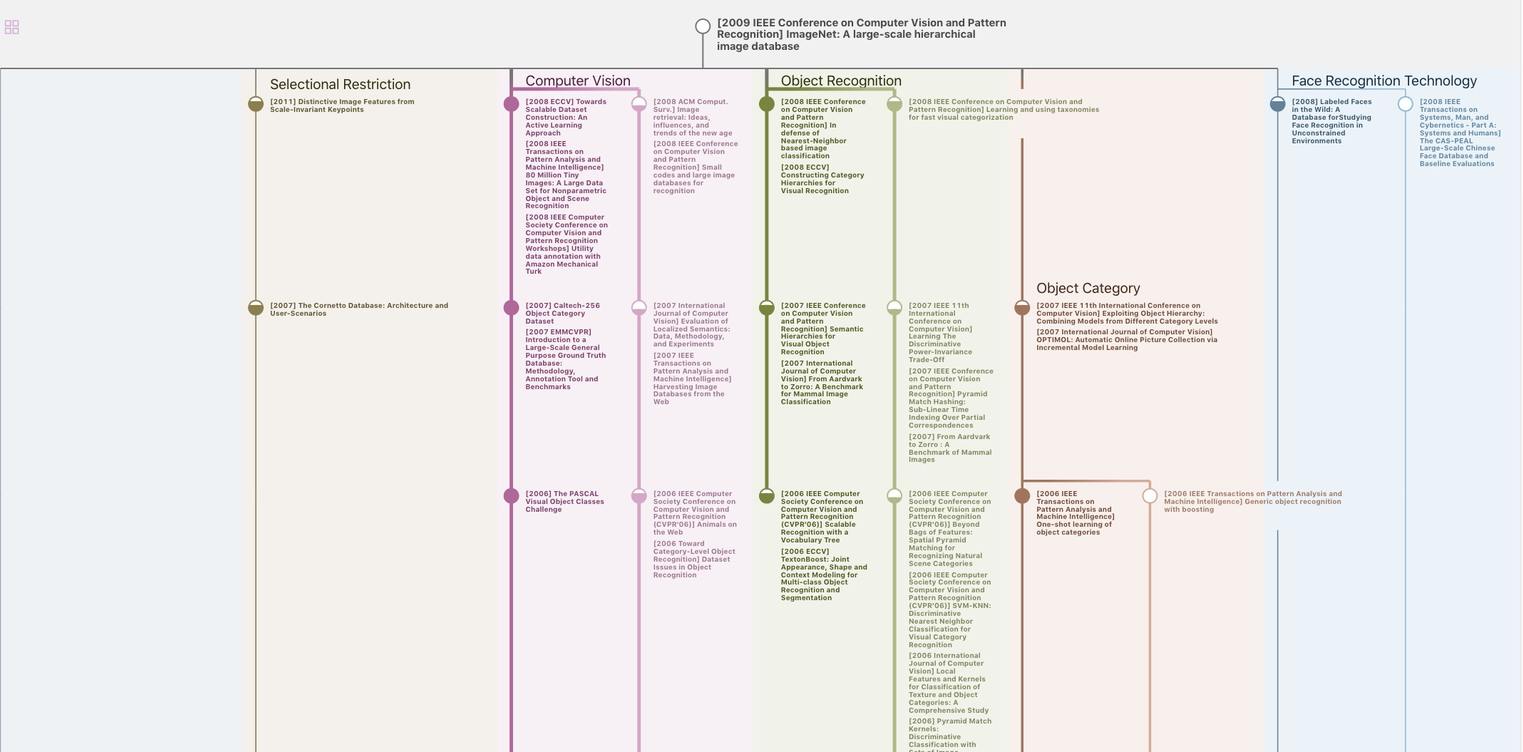
生成溯源树,研究论文发展脉络
Chat Paper
正在生成论文摘要