Predictions of Boron Phase Stability Using an Efficient Bayesian Machine Learning Interatomic Potential
JOURNAL OF PHYSICAL CHEMISTRY LETTERS(2024)
摘要
Thermodynamic phase stability of three elemental boron allotropes, i.e., alpha-B, beta-B, and gamma-B, was investigated using a Bayesian interatomic potential trained via a sparse Gaussian process (SGP). SGP potentials trained with data sets from on-the-fly active learning achieve quantum mechanical level accuracy when employed in molecular dynamics (MD) simulations to predict wide-ranging thermodynamic, structural, and vibrational properties. The simulated phase diagram (500-1400 K and 0-16 GPa) agrees with experimental measurements. The SGP-based MD simulations also successfully predicted that the B13 defect is critical in stabilizing beta-B below 700 K. At higher temperatures, the entropy becomes the dominant factor, making beta-B the more stable phase over alpha-B. This letter demonstrates that SGP potentials based on a training set consisting of defect-free-only systems could make correct predictions of defect-related phenomena in solid-state crystals, paving the path to investigate crystal phase stability and transitions.
更多查看译文
AI 理解论文
溯源树
样例
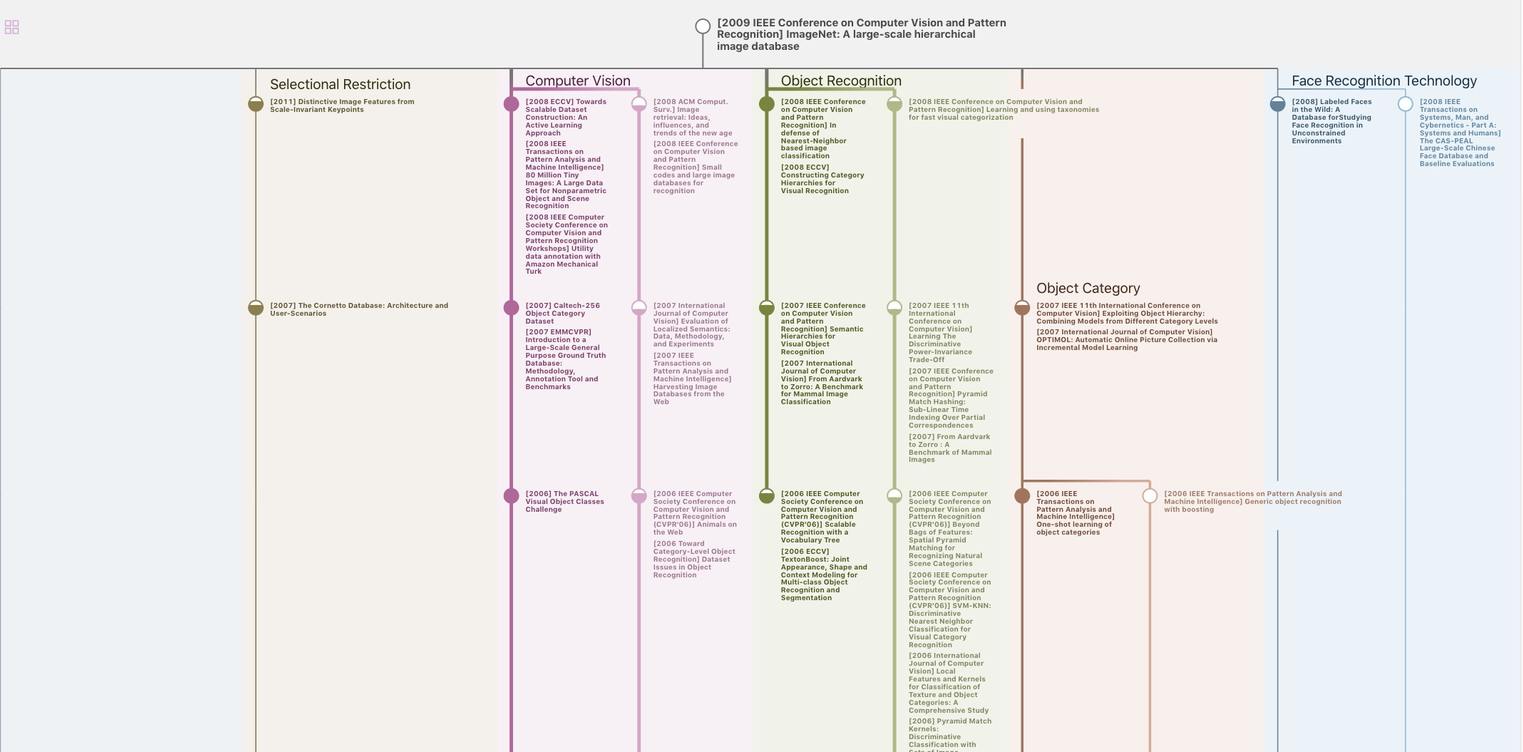
生成溯源树,研究论文发展脉络
Chat Paper
正在生成论文摘要