Confident Naturalness Explanation (CNE): A Framework to Explain and Assess Patterns Forming Naturalness
IEEE Geoscience and Remote Sensing Letters(2024)
摘要
Protected natural areas characterized by minimal modern human footprint are often challenging to assess. Machine-learning (ML) models, particularly explainable methods, offer promise in understanding and mapping the naturalness of these environments through the analysis of satellite imagery. However, current approaches encounter challenges in delivering valid and objective explanations and quantifying the contribution of specific patterns to naturalness. These challenges persist due to the reliance on hand-crafted weights assigned to contributing patterns, which can introduce subjectivity and limit the model’s ability to capture relationships within the data. We propose the confident naturalness explanation (CNE) framework to address these issues, integrating explainable ML and uncertainty quantification. This framework introduces a new quantitative metric to describe the confident contribution of patterns to the concept of naturalness. Additionally, it generates segmentation masks that depict the uncertainty levels in each pixel, highlighting areas where the model lacks knowledge. To showcase the framework’s effectiveness, we apply it to a study site in Fennoscandia, utilizing two open-source satellite datasets. In our proposed metric scale, moors and heathlands register high values of 1 and 0.81, respectively, indicating pronounced naturalness. In contrast, water bodies score lower on the scale, with a metric value of 0.18, placing them at the lower end.
更多查看译文
关键词
Explainable machine learning (ML),naturalness index,pattern recognition,remote sensing,uncertainty quantification
AI 理解论文
溯源树
样例
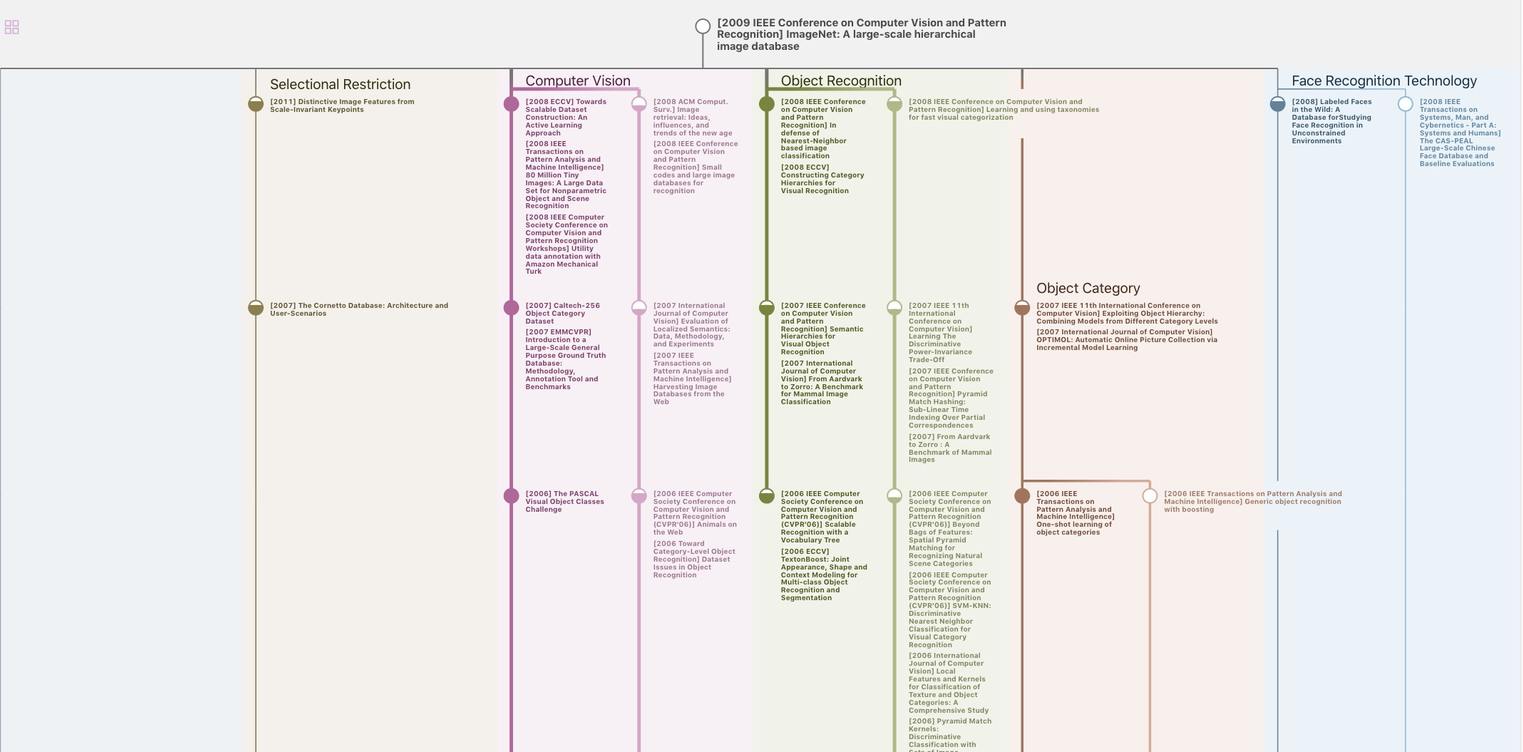
生成溯源树,研究论文发展脉络
Chat Paper
正在生成论文摘要