Robust Optical and SAR Image Matching Using Attention-Enhanced Structural Features
IEEE TRANSACTIONS ON GEOSCIENCE AND REMOTE SENSING(2024)
摘要
Due to the complementary nature of optical and synthetic aperture radar (SAR) images, their alignment is of increasing interest. However, due to the significant radiometric differences between them, precise matching becomes a very challenging problem. Although current advanced structural features and deep learning-based methods have proposed feasible solutions, there is still much potential for improvement. In this article, we propose a hybrid matching method using attention-enhanced structural features (namely AESF), which combines the advantages of both handcrafted-based and learning-based methods to improve the accuracy of optical and SAR image matching. It mainly consists of two modules: a novel effective multibranch global attention (MBGA) module and a joint multicropping image matching loss function (MCTM) module. The MBGA module is designed to focus on shared information in structural feature descriptors of heterogeneous images across space and channel dimensions, significantly improving the expressive capacity of the classical structural features and generating more refined and robust image features. The MCTM module is constructed to fully exploit the association between global and local information of the input image, which can optimize the triple loss discriminator to discriminate between positive and negative samples. To validate the effectiveness of the proposed method, it is compared with five state-of-the-art matching methods by using various optical and SAR datasets. The experimental results show that the matching accuracy at the 1-pixel threshold is improved by about 1.8%-8.7% compared with the most advanced deep learning method (OSMNet) and 6.5%-23% compared with the handcrafted description method channel feature of orientated gradient (CFOG).
更多查看译文
关键词
Attention mechanism,image matching,optical and synthetic aperture radar (SAR) images,structural features
AI 理解论文
溯源树
样例
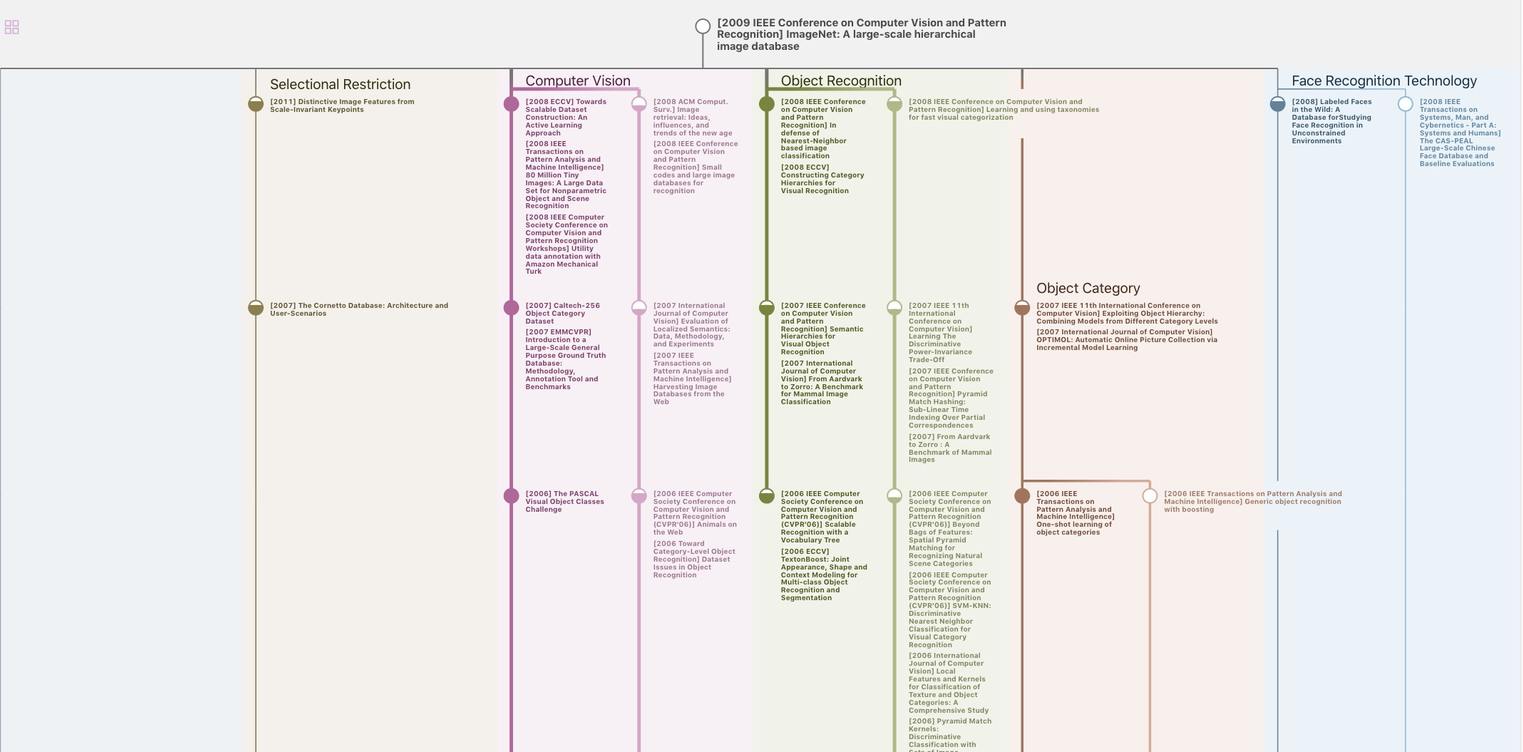
生成溯源树,研究论文发展脉络
Chat Paper
正在生成论文摘要