Spectral-Spatial Dual Graph Unfolding Network for Multispectral and Hyperspectral Image Fusion.
IEEE Trans. Geosci. Remote. Sens.(2024)
摘要
Recently, deep neural network (DNN)-based methods have achieved good results in terms of the fusion of low-spatial-resolution hyperspectral (LR HS) and high-spatial-resolution multispectral (HR MS) images. However, the spectral band correlation (SBC) and the spatial nonlocal similarity (SNS) in hyperspectral (HS) images are not sufficiently exploited by them. To model the two priors efficiently, we propose a spectral–spatial dual graph unfolding network (SDGU-Net), which is derived from the optimization of graph regularized restoration models. Specifically, we introduce spectral and spatial graphs to regularize the reconstruction of the desired high-spatial-resolution HS (HR HS) image. To explore the SBC and SNS priors of HS images in feature space and utilize the powerful learning ability of DNNs simultaneously, the iterative optimization of the spectral and spatial graph regularized models is unfolded as a network, which is composed of spectral and spatial graph unfolding modules. The two kinds of modules are designed according to the solutions of the spectral and spatial graph regularized models. In these modules, we employ graph convolution networks (GCNs) to capture the SBC and SNS in the fused image. Then, the learned features are integrated by the corresponding feature fusion modules and fed into the feature condense module to generate the HR HS image. We conduct extensive experiments on three benchmark datasets, and the results demonstrate the effectiveness of our proposed SDGU-Net.
更多查看译文
关键词
Graph unfolding network,hyperspectral (HS) image,image fusion,multispectral (MS) image,remote sensing
AI 理解论文
溯源树
样例
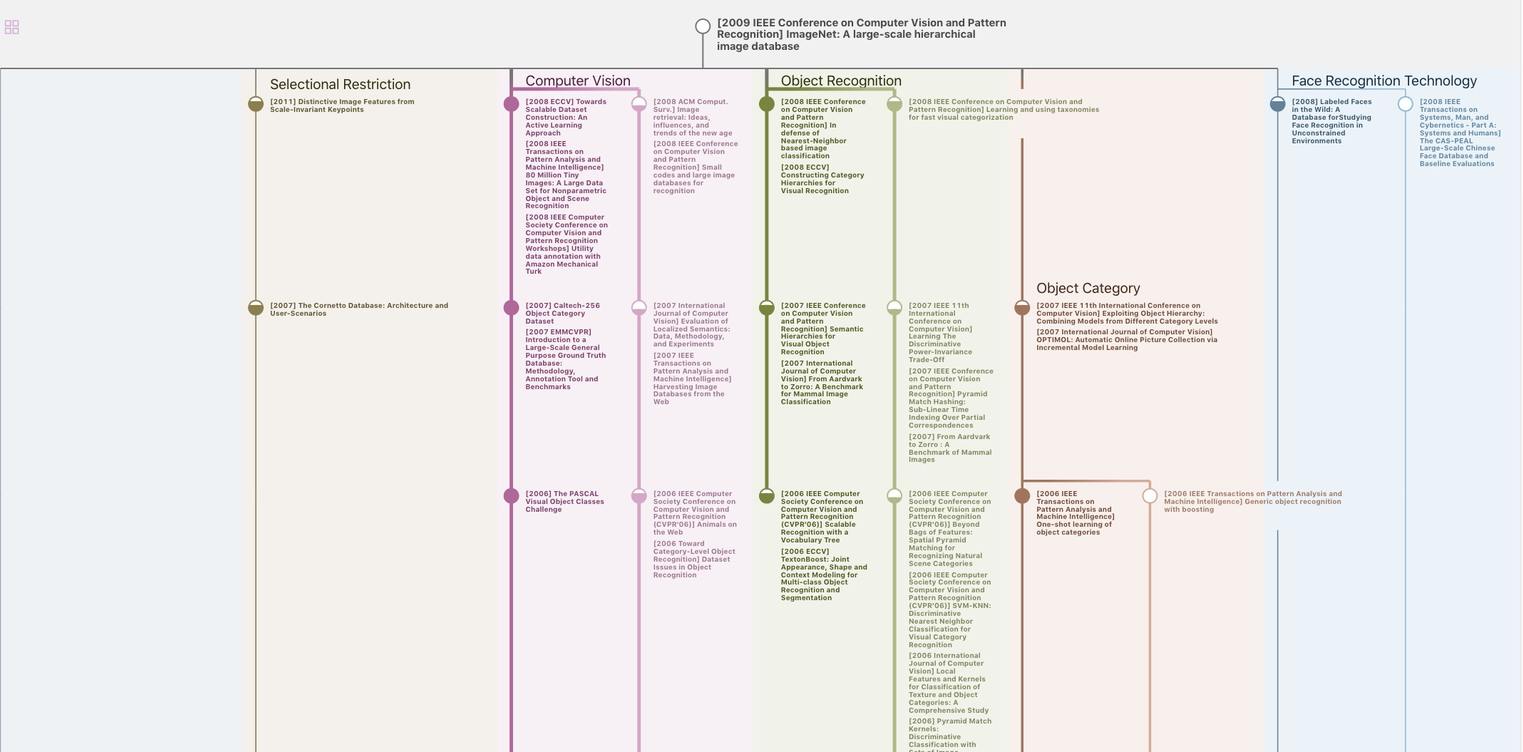
生成溯源树,研究论文发展脉络
Chat Paper
正在生成论文摘要