Distributed Generalized Nash Equilibria Computation of Noncooperative Games Via Novel Primal-Dual Splitting Algorithms
IEEE TRANSACTIONS ON SIGNAL AND INFORMATION PROCESSING OVER NETWORKS(2024)
摘要
This article investigates the generalized Nash equilibria (GNE) seeking problem for noncooperative games, where all players dedicate to selfishly minimizing their own cost functions subject to local constraints and coupled constraints. To tackle the considered problem, we initially form an explicit local equilibrium condition for its variational formulation. By employing proximal splitting operators, a novel distributed primal-dual splitting algorithm with full-decision information (Dist_PDS_FuDeIn) is designed, eliminating the need for global step-sizes. Furthermore, to address scenarios where players lack access to all other players' decisions, a local estimation is introduced to approximate the decision information of other players, and a fully distributed primal-dual splitting algorithm with partial-decision information (Dist_PDS_PaDeIn) is then proposed. Both algorithms enable the derivation of new distributed forward-backward-like extensions. Theoretically, a new analytical approach for convergence is presented, demonstrating that the proposed algorithms converge to the variational GNE of games, and their convergence rates are also proven, provided that uncoordinated step-sizes are positive and less than explicit upper bounds. Moreover, the approach not only generalizes the forward-backward splitting technique but also improves convergence rates of several well-known algorithms. Finally, the advantages of Dist_PDS_FuDeIn and Dist_PDS_PaDeIn are illustrated through comparative simulations.
更多查看译文
关键词
Games,Convergence,Costs,Information processing,Heuristic algorithms,Standards,Lyapunov methods,Distributed algorithm,generalized Nash equilibria,game theory,operator splitting
AI 理解论文
溯源树
样例
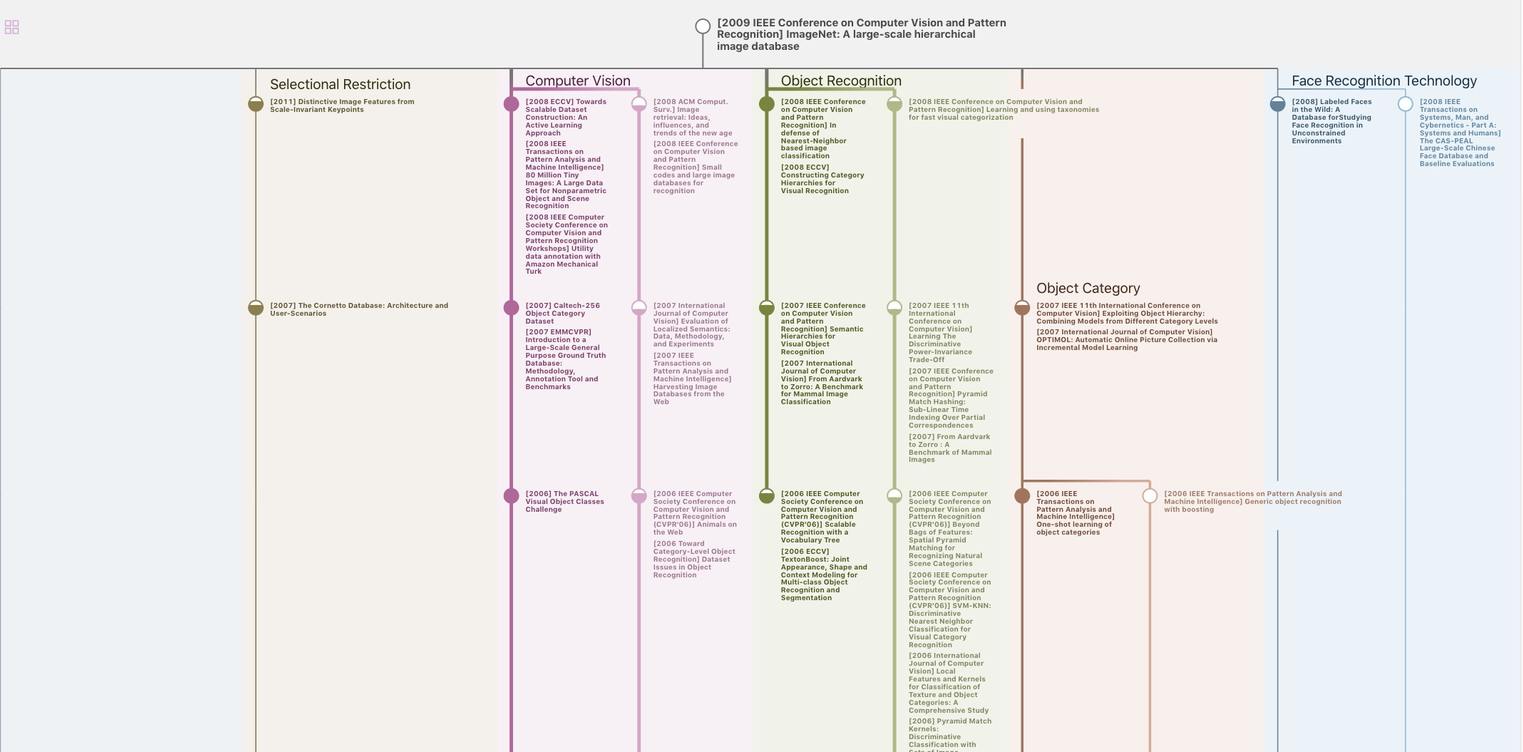
生成溯源树,研究论文发展脉络
Chat Paper
正在生成论文摘要