Fluid Viscosity and Density Determination With Machine Learning-Enhanced Coriolis Mass Flow Sensors
2024 IEEE 37th International Conference on Micro Electro Mechanical Systems (MEMS)(2024)
摘要
We report on statistical machine learning methods applied to a Coriolis-based sensor chip to accurately estimate liquid density and dynamic viscosity for trained liquids. This estimation is challenging with conventional methods due to non-ideal sensor effects. The chip (1.2 cm
2
) has been exposed to different combinations of temperatures, flows, and pressures for three different liquids: ethanol, water and isopropanol. The statistical machine learning methods have been applied to the raw sampled signals of the sensing structures. The results have been obtained for different temperatures and shown to be less dependent on the liquid state (i.e., pressure and flow). The best-performing method was the Gaussian Process Regression (GPR) method, which results in a mean absolute percentage error of < 0.01 % and < 1 % for density and viscosity respectively, which is a factor of > 4 better compared to conventional methods.
更多查看译文
关键词
Microfluidics,Coriolis,mass flow sensors,machine learning,regression,viscosity,density,estimation
AI 理解论文
溯源树
样例
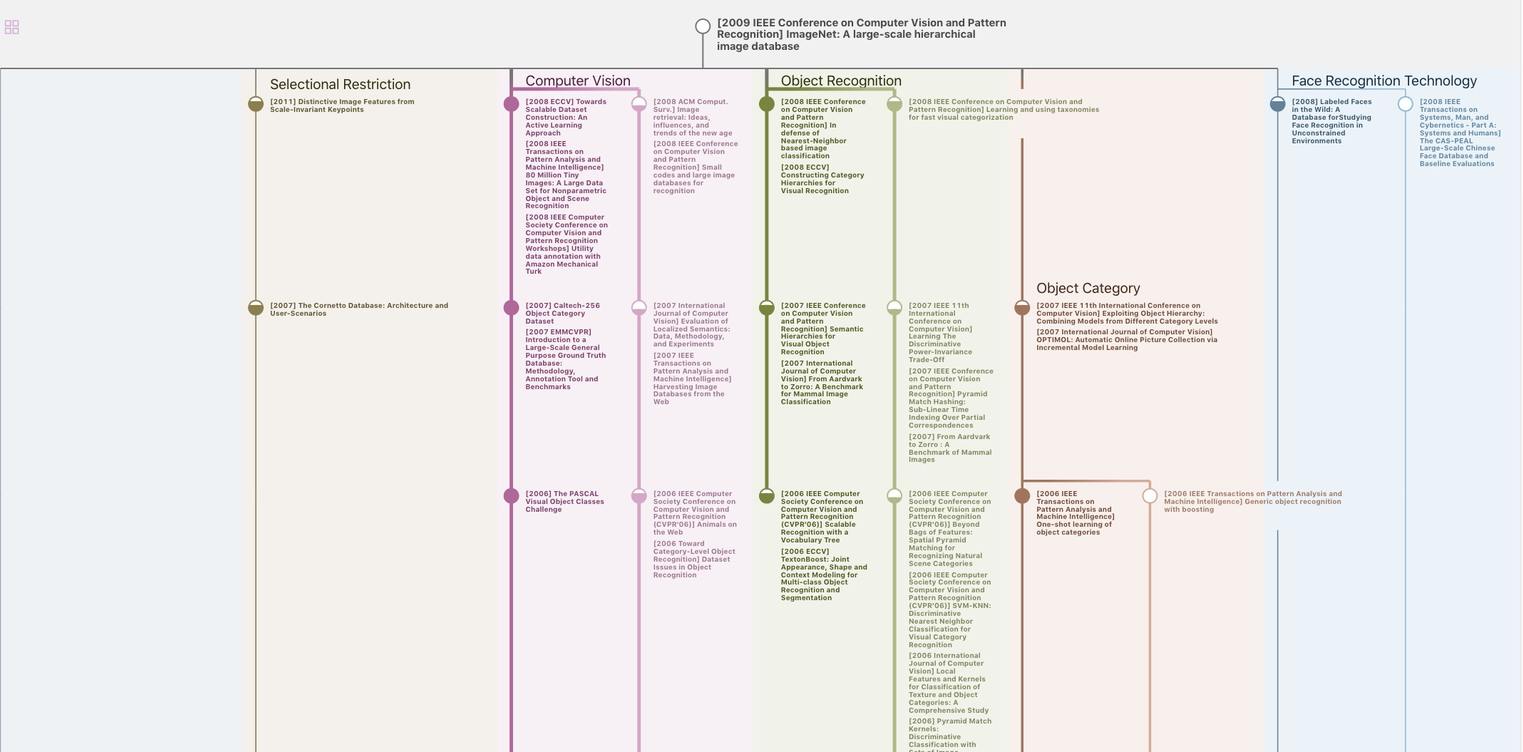
生成溯源树,研究论文发展脉络
Chat Paper
正在生成论文摘要