Distance-weighted Sinkhorn loss for Alzheimer’s disease classification
iScience(2024)
摘要
Traditional loss functions such as cross-entropy loss often quantify the penalty for each mis-classified training sample without adequately considering its distance from the ground truth class distribution in the feature space. Intuitively, the larger this distance is, the higher the penalty should be. With this observation, we propose a penalty called distance-weighted Sinkhorn (DWS) loss. For each mis-classified training sample (with predicted label A and true label B), its contribution to the DWS loss positively correlates to the distance the training sample needs to travel to reach the ground truth distribution of all the A samples. We apply the DWS framework with a neural network to classify different stages of Alzheimer’s disease. Our empirical results demonstrate that the DWS framework outperforms the traditional neural network loss functions and is comparable or better to traditional machine learning methods, highlighting its potential in biomedical informatics and data science.
更多查看译文
关键词
Medical informatics,Biocomputational method,Classification of bioinformatical subject,Neural networks,Machine learning
AI 理解论文
溯源树
样例
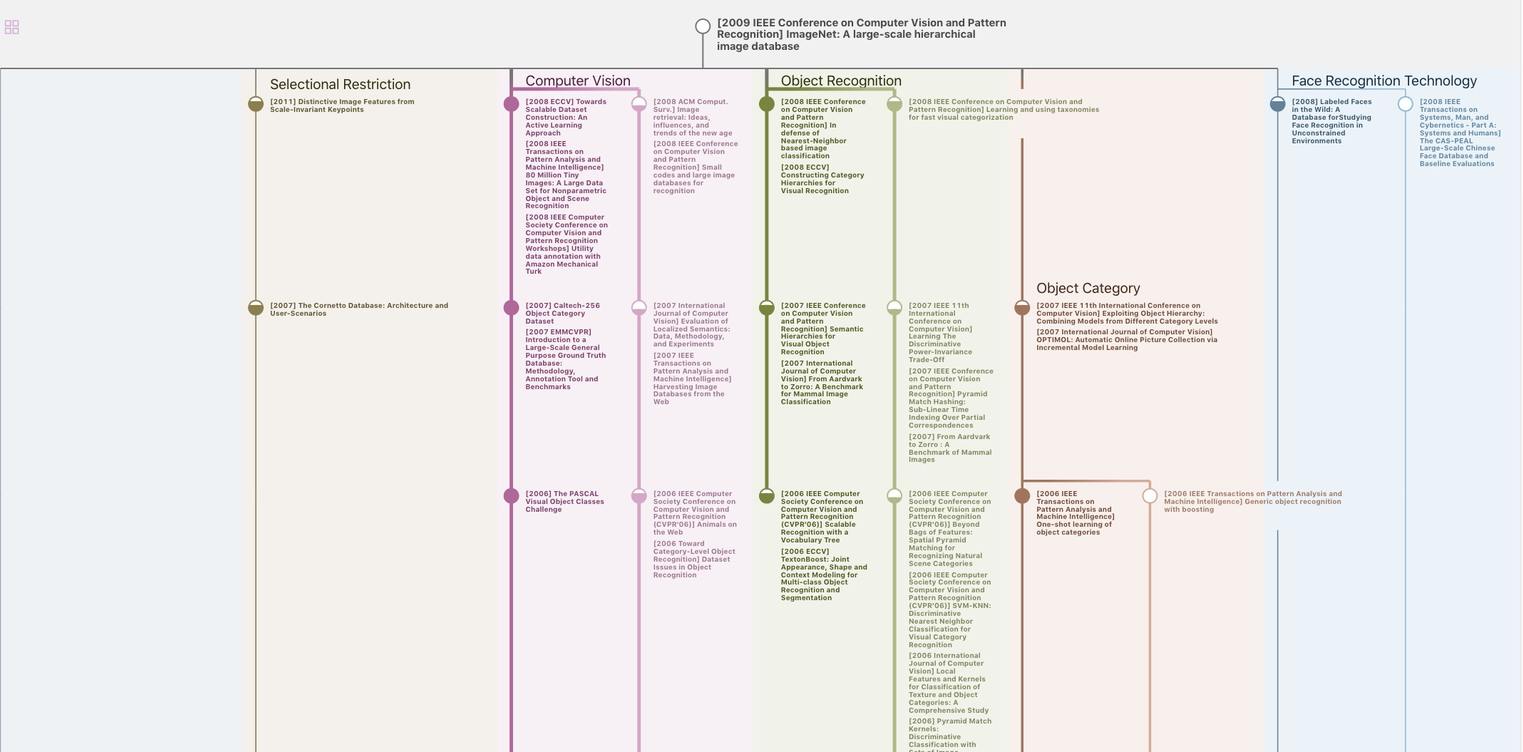
生成溯源树,研究论文发展脉络
Chat Paper
正在生成论文摘要