Design and Evaluation of Low Voltage Neural Network-Based State Estimators in Scenarios With Minimal Measurement Infrastructure
IEEE ACCESS(2024)
摘要
Learning-based state estimators can represent a cost-effective opportunity for distribution system operators to perform grid monitoring and control in low-voltage grids where the measuring infrastructure is minimal, if not absent. This study lays the foundation for designing and evaluating neural network-based state estimators for low-voltage radial distribution grids. A simulation-based methodology is proposed for generating synthetic training data-sets relying only on minimal grid data. Additionally, a novel framework for performance analysis of low voltage learning-based state estimators is considered, which relies on a bi-dimensional evaluation of the absolute error and the parallel observation of relative metrics. The applicability and potential of these estimators have been tested and validated through various low-voltage radial case studies, showing promising results especially for large distribution grids. Finally, a propagation error study has been conducted to observe how these estimators handle errors in input measurements.
更多查看译文
关键词
Distribution networks,State estimation,grid monitoring,neural networks,observability,state estimation
AI 理解论文
溯源树
样例
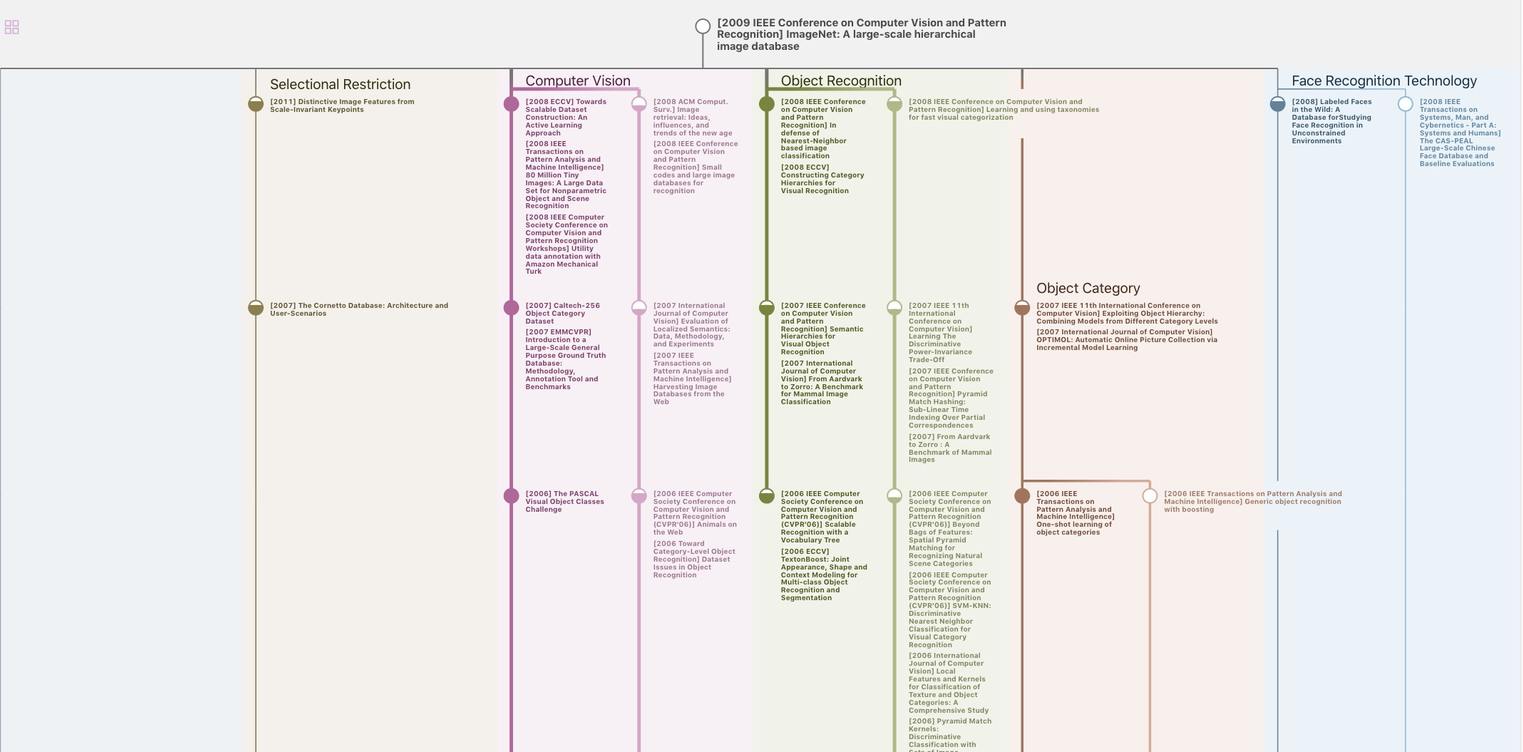
生成溯源树,研究论文发展脉络
Chat Paper
正在生成论文摘要