HGNN-QSSA: Heterogeneous Graph Neural Networks With Quantitative Sampling and Structure-Aware Attention
IEEE ACCESS(2024)
摘要
Heterogeneous information networks provide abundant structural and semantic information. Two main strategies for leveraging this data include meta-path-based and meta-path-free methods. The effectiveness of the former heavily depends on the quality of manually defined meta-paths, which may lead to the instability of the model. However, the existing meta-path-free methods lack of neighbor screening during aggregating, and there is also an overemphasis on attribute information. To address these issues, we propose the Heterogeneous Graph Neural Network model by incorporating Quantitative Sampling and Structure-aware Attention. We introduce a Quantitative Sampling Module that calculates the similarity between neighbors of the target nodes and target nodes, enabling us to select the top k nodes with the strongest relevance to the target node based on this measure, and incorporate a Structure-aware Attention Module during the aggregation of neighbor information. This module combines both structural and attribute information to aggregate the neighbor information effectively. By implementing these improvements, our proposed model exhibits superior performance compared to several state-of-the-art methods on two real-world datasets.
更多查看译文
关键词
Graph neural networks,Social networking (online),Correlation,Prediction algorithms,Task analysis,Stacking,Semantics,Information systems,Heterogeneous networks,Detection algorithms,Heterogeneous information network,community detection,quantitative sampling,structure-aware attention
AI 理解论文
溯源树
样例
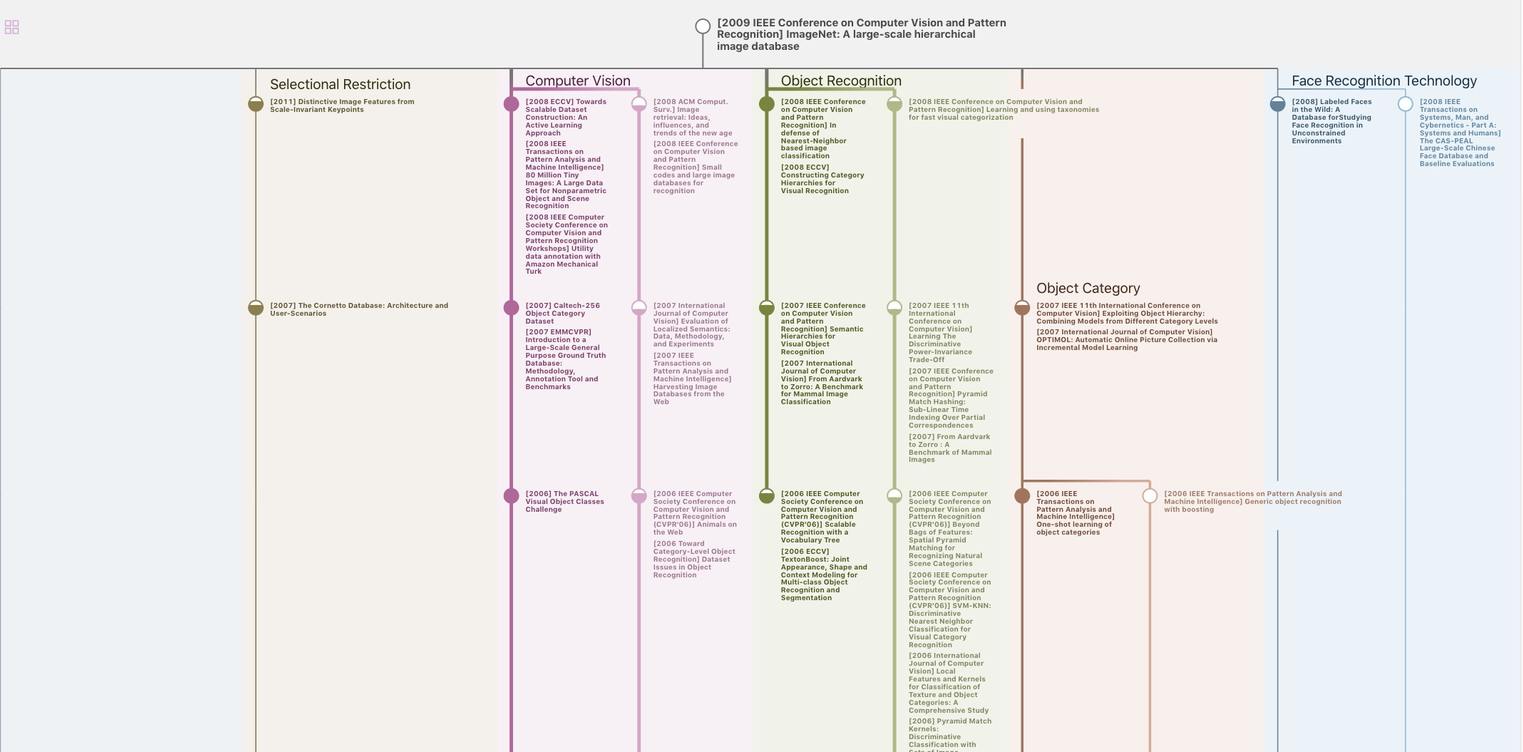
生成溯源树,研究论文发展脉络
Chat Paper
正在生成论文摘要