Pathological Priors Inspired Network for Vertebral Osteophytes Recognition.
IEEE transactions on medical imaging(2024)
摘要
Automatic vertebral osteophyte recognition in Digital Radiography is of great importance for the early prediction of degenerative disease but is still a challenge because of the tiny size and high inter-class similarity between normal and osteophyte vertebrae. Meanwhile, common sampling strategies applied in Convolution Neural Network could cause detailed context loss. All of these could lead to an incorrect positioning predicament. In this paper, based on important pathological priors, we define a set of potential lesions of each vertebra and propose a novel Pathological Priors Inspired Network (PPIN) to achieve accurate osteophyte recognition. PPIN comprises a backbone feature extractor integrating with a Wavelet Transform Sampling module for high-frequency detailed context extraction, a detection branch for locating all potential lesions and a classification branch for producing final osteophyte recognition. The Anatomical Map-guided Filter between two branches helps the network focus on the specific anatomical regions via the generated heatmaps of potential lesions in the detection branch to address the incorrect positioning problem. To reduce the inter-class similarity, a Bilateral Augmentation Module based on the graph relationship is proposed to imitate the clinical diagnosis process and to extract discriminative contextual information between adjacent vertebrae in the classification branch. Experiments on the two osteophytes-specific datasets collected from the public VinDr-Spine database show that the proposed PPIN achieves the best recognition performance among multitask frameworks and shows strong generalization. The results on a private dataset demonstrate the potential in clinical application. The Class Activation Maps also show the powerful localization capability of PPIN. The source codes are available in https://github.com/Phalo/PPIN.
更多查看译文
关键词
Multitask learning,Pathological prior,Graph convolution network,Wavelet transform
AI 理解论文
溯源树
样例
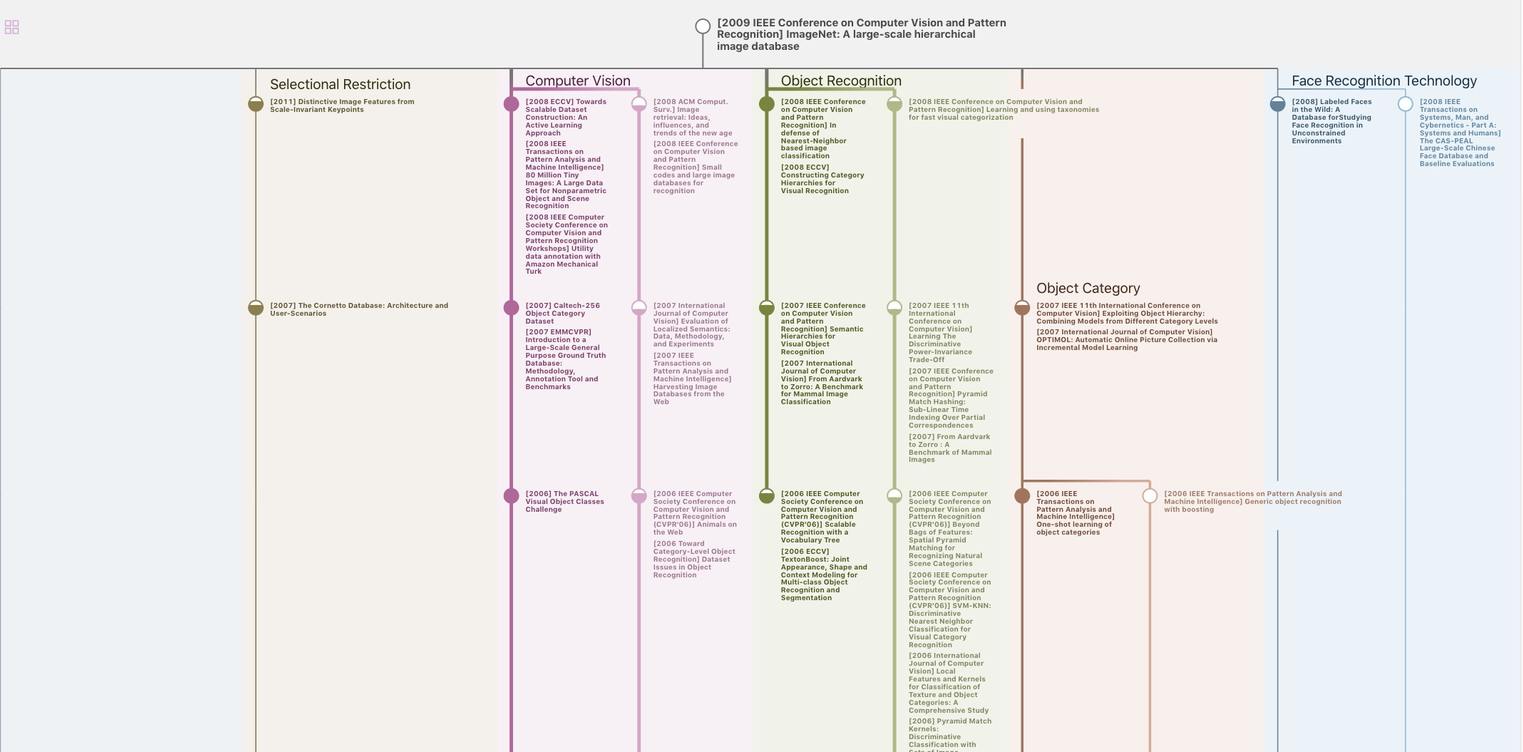
生成溯源树,研究论文发展脉络
Chat Paper
正在生成论文摘要