Double-Branch Multi-Scale Contextual Network: A Model for Multi-Scale Street Tree Segmentation in High-Resolution Remote Sensing Images
SENSORS(2024)
摘要
Street trees are of great importance to urban green spaces. Quick and accurate segmentation of street trees from high-resolution remote sensing images is of great significance in urban green space management. However, traditional segmentation methods can easily miss some targets because of the different sizes of street trees. To solve this problem, we propose the Double-Branch Multi-Scale Contextual Network (DB-MSC Net), which has two branches and a Multi-Scale Contextual (MSC) block in the encoder. The MSC block combines parallel dilated convolutional layers and transformer blocks to enhance the network's multi-scale feature extraction ability. A channel attention mechanism (CAM) is added to the decoder to assign weights to features from RGB images and the normalized difference vegetation index (NDVI). We proposed a benchmark dataset to test the improvement of our network. Experimental research showed that the DB-MSC Net demonstrated good performance compared with typical methods like Unet, HRnet, SETR and recent methods. The overall accuracy (OA) was improved by at least 0.16% and the mean intersection over union was improved by at least 1.13%. The model's segmentation accuracy meets the requirements of urban green space management.
更多查看译文
关键词
street trees,segmentation,db-msc net,deep learning,neural network,remote sensing images
AI 理解论文
溯源树
样例
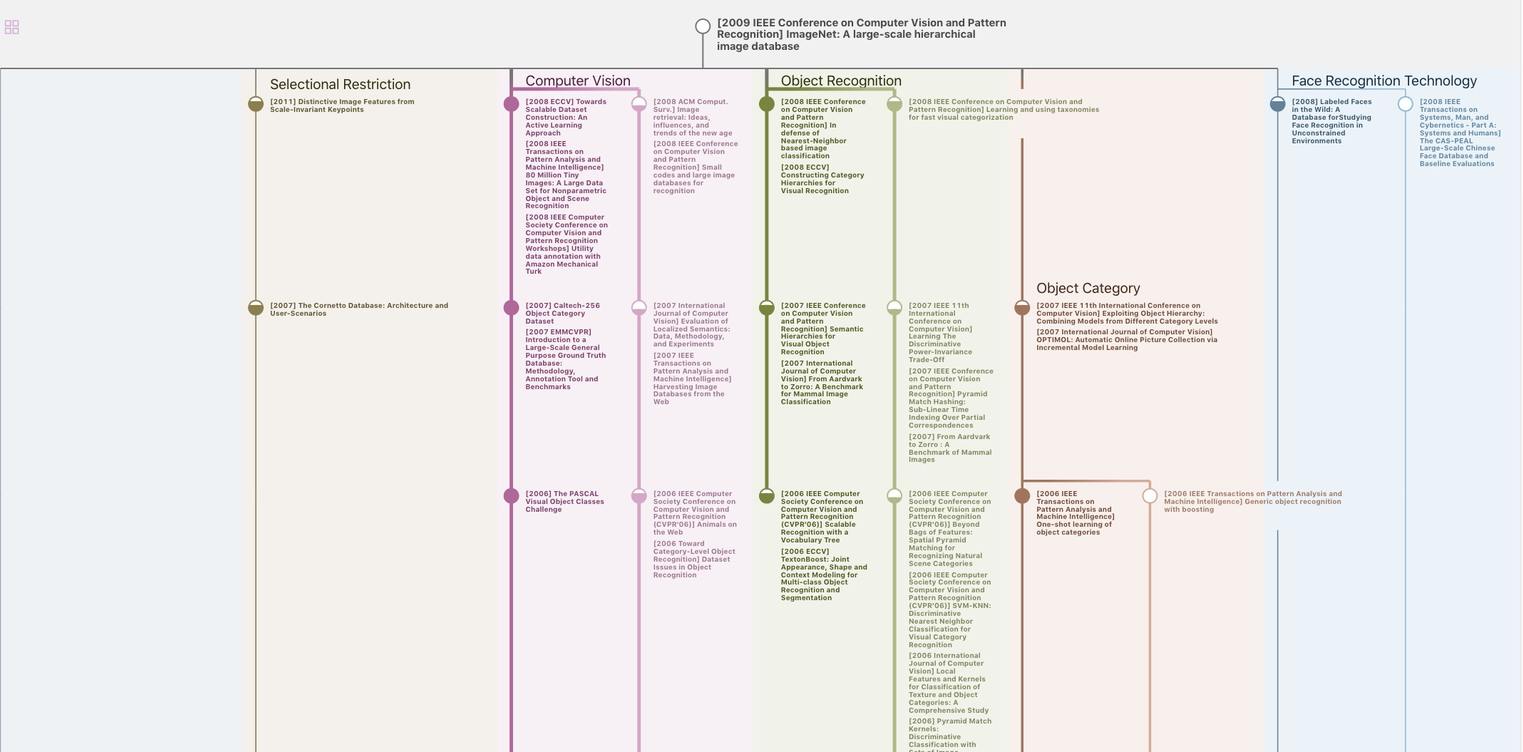
生成溯源树,研究论文发展脉络
Chat Paper
正在生成论文摘要