Lightweight Vehicle Detection Based on Improved YOLOv5s
SENSORS(2024)
摘要
A vehicle detection algorithm is an indispensable component of intelligent traffic management and control systems, influencing the efficiency and functionality of the system. In this paper, we propose a lightweight improvement method for the YOLOv5 algorithm based on integrated perceptual attention, with few parameters and high detection accuracy. First, we propose a lightweight module IPA with a Transformer encoder based on integrated perceptual attention, which leads to a reduction in the number of parameters while capturing global dependencies for richer contextual information. Second, we propose a lightweight and efficient multiscale spatial channel reconstruction (MSCCR) module that does not increase parameter and computational complexity and facilitates representative feature learning. Finally, we incorporate the IPA module and the MSCCR module into the YOLOv5s backbone network to reduce model parameters and improve accuracy. The test results show that, compared with the original model, the model parameters decrease by about 9%, the average accuracy (mAP@50) increases by 3.1%, and the FLOPS does not increase.
更多查看译文
关键词
artificial intelligence,deep learning,object detection,vehicle detection,lightweight
AI 理解论文
溯源树
样例
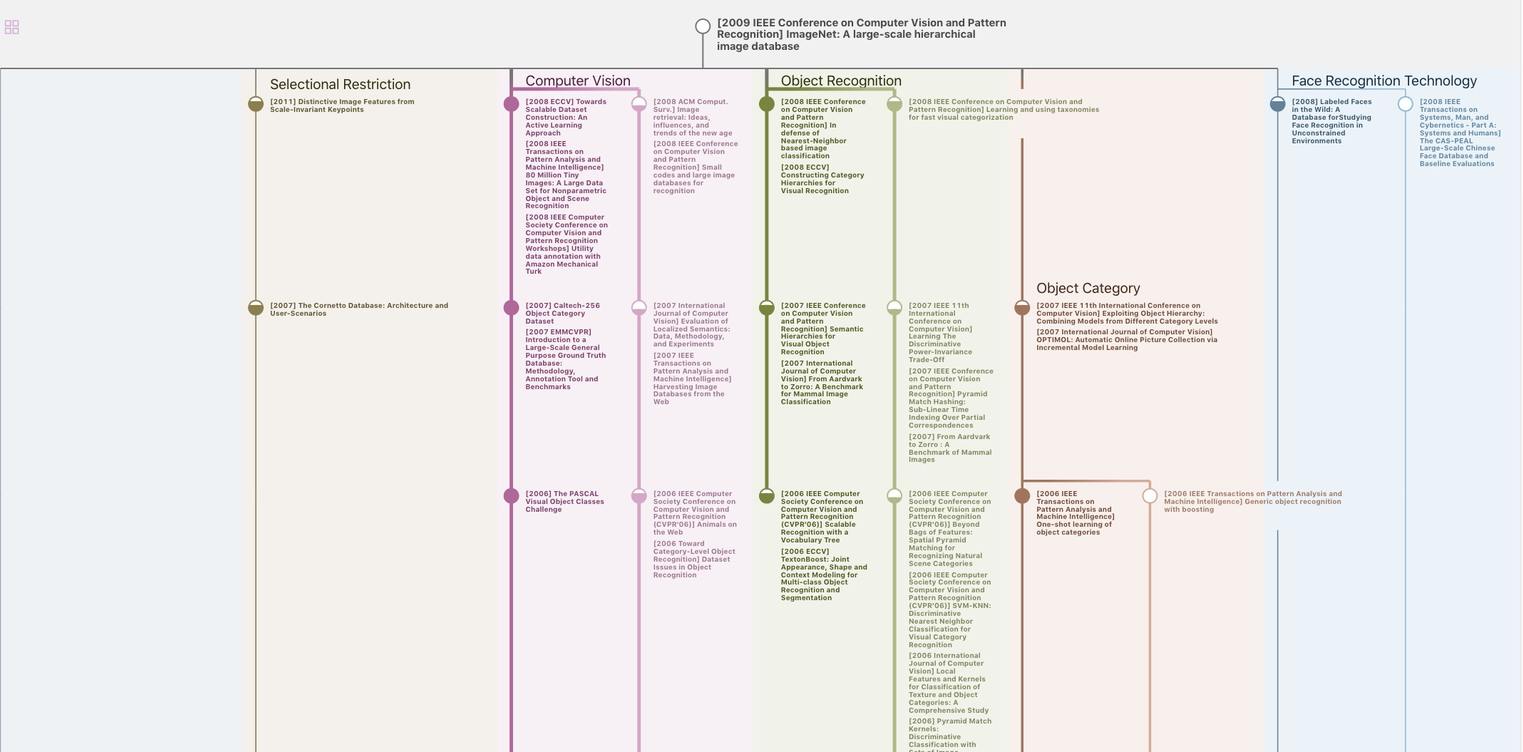
生成溯源树,研究论文发展脉络
Chat Paper
正在生成论文摘要