AST3DRNet: Attention-Based Spatio-Temporal 3D Residual Neural Networks for Traffic Congestion Prediction
SENSORS(2024)
摘要
Traffic congestion prediction has become an indispensable component of an intelligent transport system. However, one limitation of the existing methods is that they treat the effects of spatio-temporal correlations on traffic prediction as invariable during modeling spatio-temporal features, which results in inadequate modeling. In this paper, we propose an attention-based spatio-temporal 3D residual neural network, named AST3DRNet, to directly forecast the congestion levels of road networks in a city. AST3DRNet combines a 3D residual network and a self-attention mechanism together to efficiently model the spatial and temporal information of traffic congestion data. Specifically, by stacking 3D residual units and 3D convolution, we proposed a 3D convolution module that can simultaneously capture various spatio-temporal correlations. Furthermore, a novel spatio-temporal attention module is proposed to explicitly model the different contributions of spatio-temporal correlations in both spatial and temporal dimensions through the self-attention mechanism. Extensive experiments are conducted on a real-world traffic congestion dataset in Kunming, and the results demonstrate that AST3DRNet outperforms the baselines in short-term (5/10/15 min) traffic congestion predictions with an average accuracy improvement of 59.05%, 64.69%, and 48.22%, respectively.
更多查看译文
关键词
traffic congestion prediction,3D convolution,3D residual unit,self-attention mechanism,spatio-temporal attention
AI 理解论文
溯源树
样例
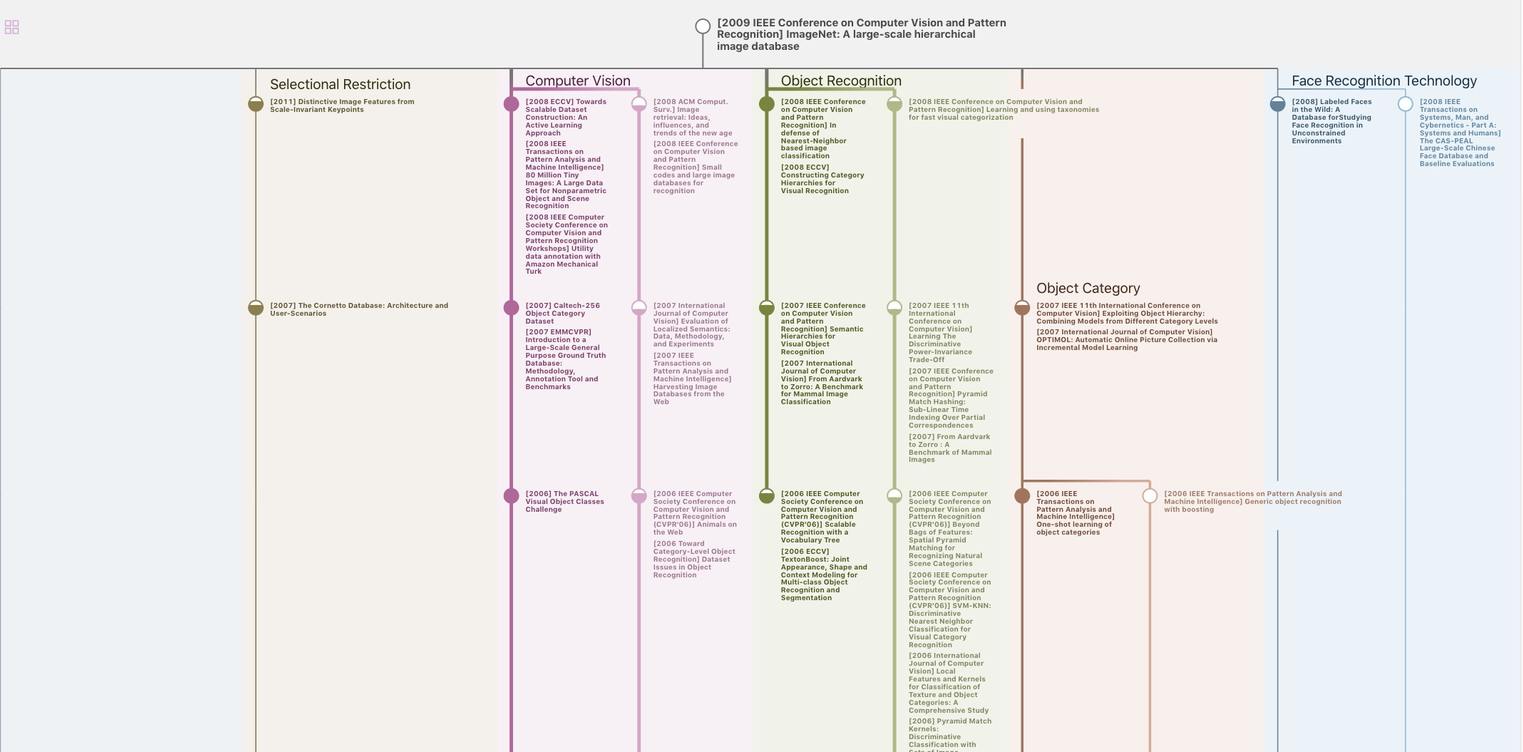
生成溯源树,研究论文发展脉络
Chat Paper
正在生成论文摘要