Learn to Bet: Using Reinforcement Learning to Improve Vehicle Bids in Auction-Based Smart Intersections
SENSORS(2024)
摘要
With the advent of IoT, cities will soon be populated by autonomous vehicles and managed by intelligent systems capable of actively interacting with city infrastructures and vehicles. In this work, we propose a model based on reinforcement learning that teaches to autonomous connected vehicles how to save resources while navigating in such an environment. In particular, we focus on budget savings in the context of auction-based intersection management systems. We trained several models with Deep Q-learning by varying traffic conditions to find the most performance-effective variant in terms of the trade-off between saved currency and trip times. Afterward, we compared the performance of our model with previously proposed and random strategies, even under adverse traffic conditions. Our model appears to be robust and manages to save a considerable amount of currency without significantly increasing the waiting time in traffic. For example, the learner bidder saves at least 20% of its budget with heavy traffic conditions and up to 74% in lighter traffic with respect to a standard bidder, and around three times the saving of a random bidder. The results and discussion suggest practical adoption of the proposal in a foreseen future real-life scenario.
更多查看译文
关键词
deep reinforcement learning,smart city,intersection management,auctions,connected vehicles,autonomous vehicles
AI 理解论文
溯源树
样例
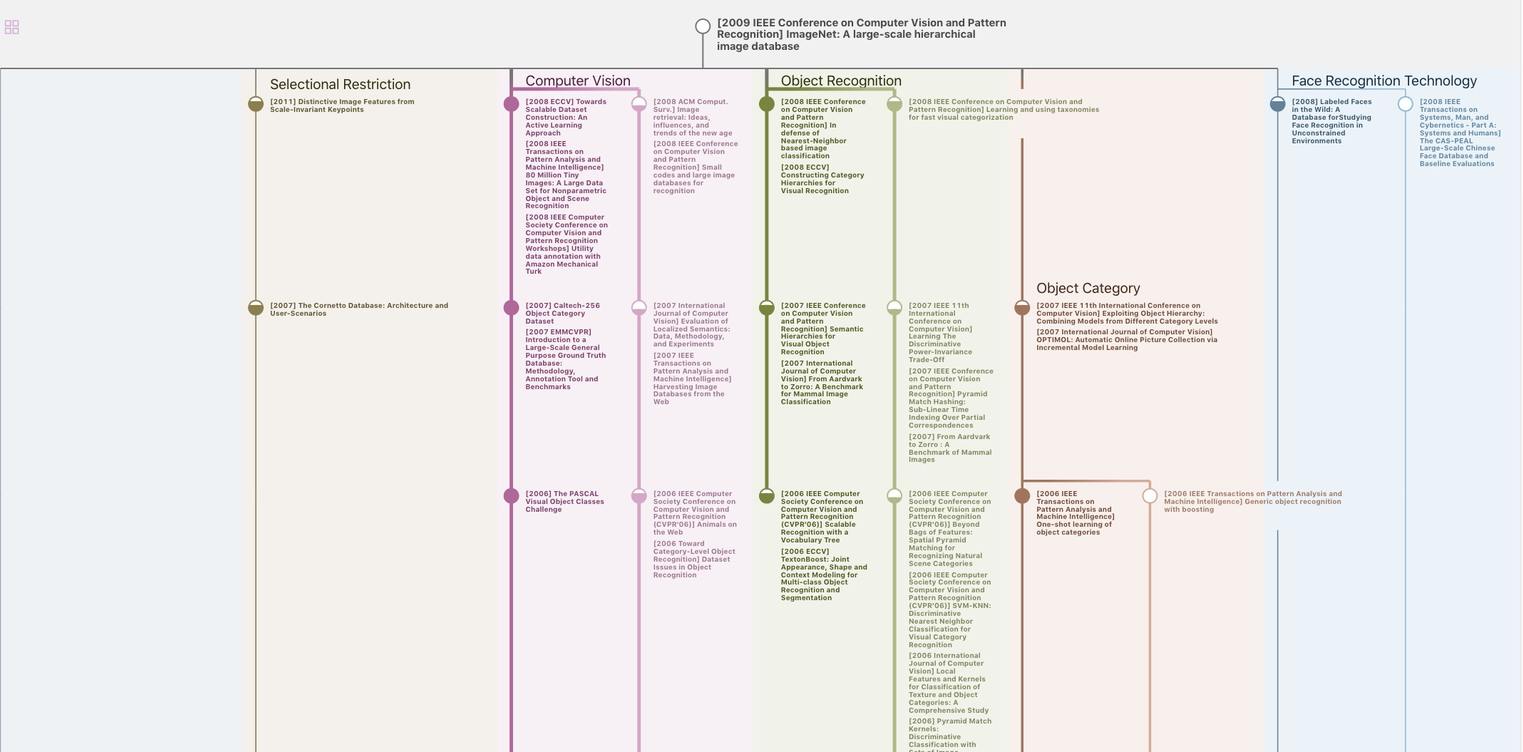
生成溯源树,研究论文发展脉络
Chat Paper
正在生成论文摘要