Automatic Identification System-Based Prediction of Tanker and Cargo Estimated Time of Arrival in Narrow Waterways
JOURNAL OF MARINE SCIENCE AND ENGINEERING(2024)
摘要
In maritime logistics, accurately predicting the Estimated Time of Arrival (ETA) of vessels is pivotal for optimizing port operations and the global supply chain. This study proposes a machine learning method for predicting ETA, drawing on historical Automatic Identification System (AIS) data spanning 2018 to 2020. The proposed framework includes a preprocessing module for extracting, transforming, and applying feature engineering to raw AIS data, alongside a modeling module that employs an XGBoost model to accurately estimate vessel travel times. The framework's efficacy was validated using AIS data from the Port of Houston, and the results indicate that the model can estimate travel times with a Mean Absolute Percentage Error (MAPE) of just 5%. Moreover, the model retains consistent accuracy in a simplified form, pointing towards the potential for reduced complexity and increased generalizability in maritime ETA predictions.
更多查看译文
关键词
travel time,ETA prediction,AIS data,XGBoost
AI 理解论文
溯源树
样例
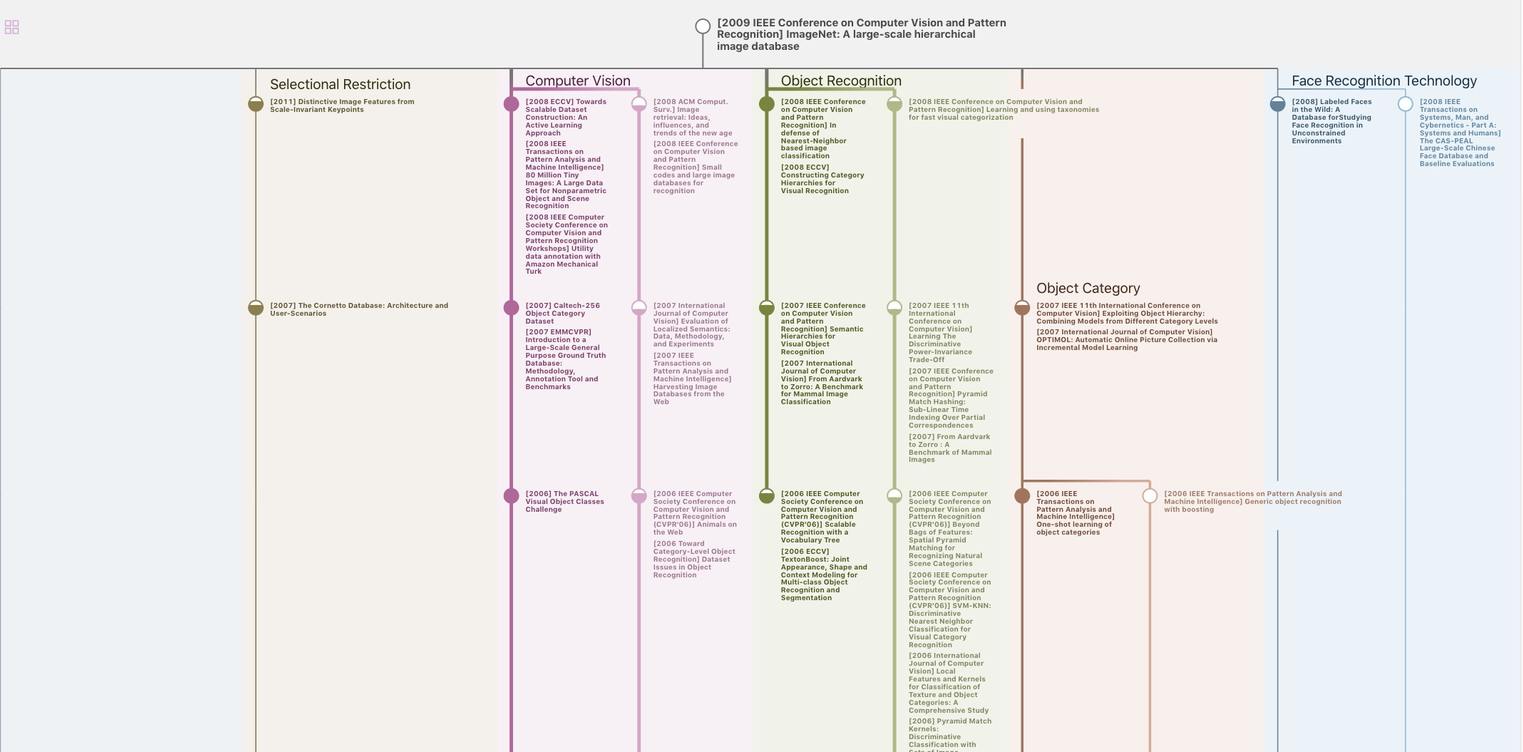
生成溯源树,研究论文发展脉络
Chat Paper
正在生成论文摘要