Respiratory Motion Prediction with Empirical Mode Decomposition-Based Random Vector Functional Link
MATHEMATICS(2024)
摘要
The precise prediction of tumor motion for radiotherapy has proven challenging due to the non-stationary nature of respiration-induced motion, frequently accompanied by unpredictable irregularities. Despite the availability of numerous prediction methods for respiratory motion prediction, the prediction errors they generate often suffer from large prediction horizons, intra-trace variabilities, and irregularities. To overcome these challenges, we have employed a hybrid method, which combines empirical mode decomposition (EMD) and random vector functional link (RVFL), referred to as EMD-RVFL. In the initial stage, EMD is used to decompose respiratory motion into interpretable intrinsic mode functions (IMFs) and residue. Subsequently, the RVFL network is trained for each obtained IMF and residue. Finally, the prediction results of all the IMFs and residue are summed up to obtain the final predicted output. We validated this proposed method on the benchmark datasets of 304 respiratory motion traces obtained from 31 patients for various prediction lengths, which are equivalent to the latencies of radiotherapy systems. In direct comparison with existing prediction techniques, our hybrid architecture consistently delivers a robust and highly accurate prediction performance. This proof-of-concept study indicates that the proposed approach is feasible and has the potential to improve the accuracy and effectiveness of radiotherapy treatment.
更多查看译文
关键词
radiotherapy,respiratory motion,prediction,empirical mode decomposition (EMD),random vector functional link (RVFL)
AI 理解论文
溯源树
样例
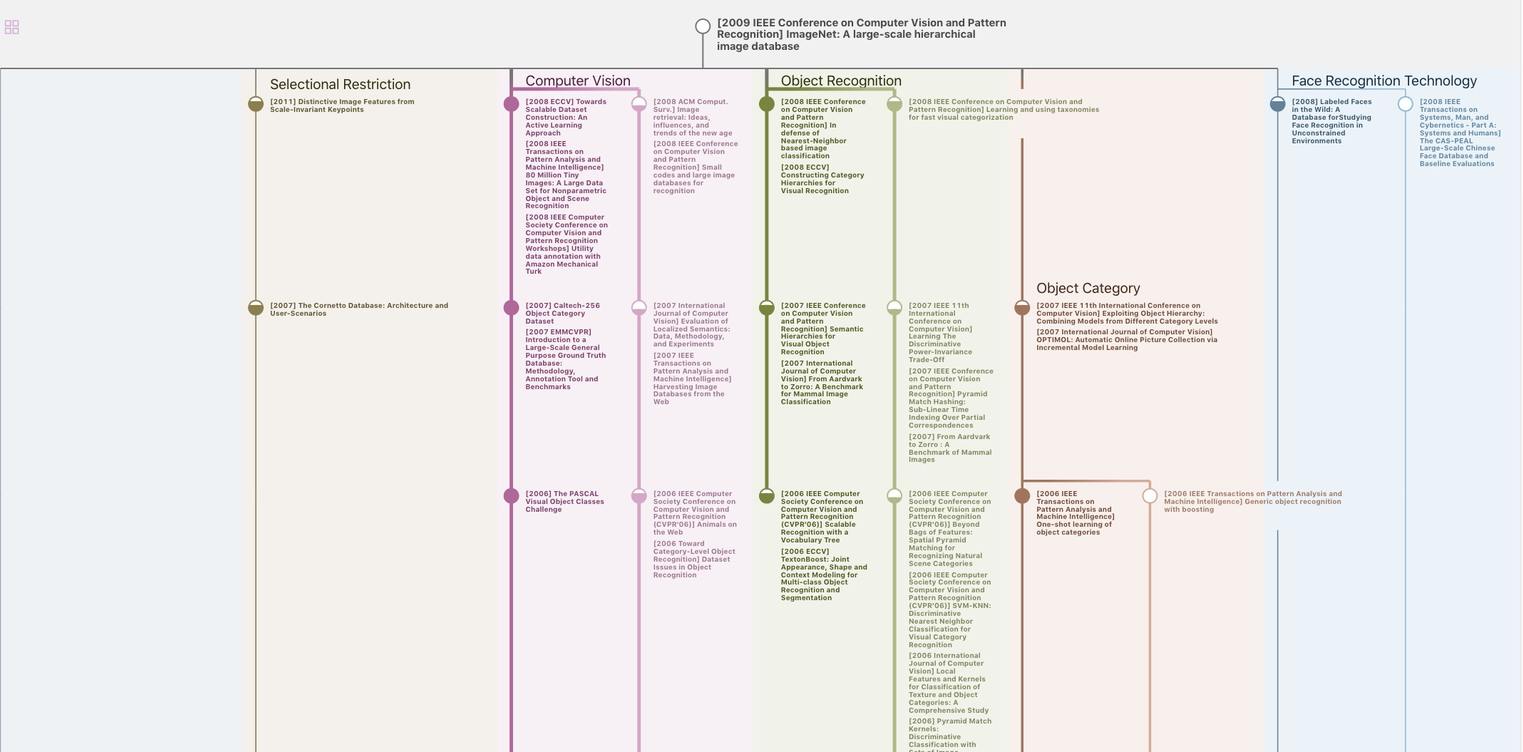
生成溯源树,研究论文发展脉络
Chat Paper
正在生成论文摘要