The Rise of Hypothesis-Driven Artificial Intelligence in Oncology
CANCERS(2024)
摘要
Simple Summary This review introduces a new class of Artificial Intelligence (AI) algorithms called hypothesis-driven AI. We elaborate on how this new class of AI methods differs from conventional AI using published AI algorithms and illustrate the power of hypothesis-driven AI in making new discoveries in cancer research. Finally, we outline the ingredients needed to overcome limitations and expand hypothesis-driven AI in the near future.Abstract Cancer is a complex disease involving the deregulation of intricate cellular systems beyond genetic aberrations and, as such, requires sophisticated computational approaches and high-dimensional data for optimal interpretation. While conventional artificial intelligence (AI) models excel in many prediction tasks, they often lack interpretability and are blind to the scientific hypotheses generated by researchers to enable cancer discoveries. Here we propose that hypothesis-driven AI, a new emerging class of AI algorithm, is an innovative approach to uncovering the complex etiology of cancer from big omics data. This review exemplifies how hypothesis-driven AI is different from conventional AI by citing its application in various areas of oncology including tumor classification, patient stratification, cancer gene discovery, drug response prediction, and tumor spatial organization. Our aim is to stress the feasibility of incorporating domain knowledge and scientific hypotheses to craft the design of new AI algorithms. We showcase the power of hypothesis-driven AI in making novel cancer discoveries that can be overlooked by conventional AI methods. Since hypothesis-driven AI is still in its infancy, open questions such as how to better incorporate new knowledge and biological perspectives to ameliorate bias and improve interpretability in the design of AI algorithms still need to be addressed. In conclusion, hypothesis-driven AI holds great promise in the discovery of new mechanistic and functional insights that explain the complexity of cancer etiology and potentially chart a new roadmap to improve treatment regimens for individual patients.
更多查看译文
关键词
Artificial Intelligence,machine learning,domain knowledge,system biology,oncology
AI 理解论文
溯源树
样例
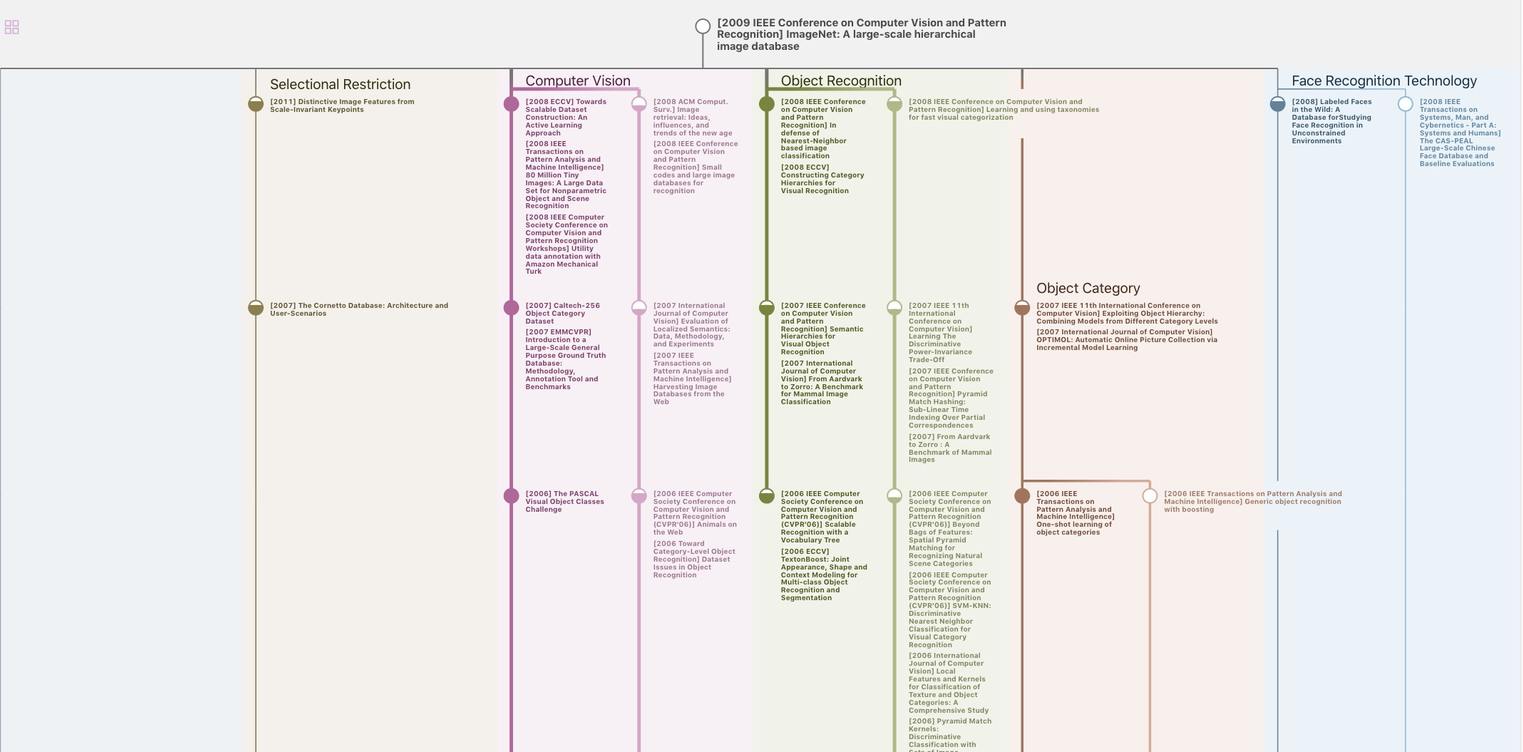
生成溯源树,研究论文发展脉络
Chat Paper
正在生成论文摘要