Machine-Learning-Based Classification Model to Address Diagnostic Challenges in Transbronchial Lung Biopsy.
Cancers(2024)
摘要
BACKGROUND:When obtaining specimens from pulmonary nodules in TBLB, distinguishing between benign samples and mis-sampling from a tumor presents a challenge. Our objective is to develop a machine-learning-based classifier for TBLB specimens.
METHODS:Three pathologists assessed six pathological findings, including interface bronchitis/bronchiolitis (IB/B), plasma cell infiltration (PLC), eosinophil infiltration (Eo), lymphoid aggregation (Ly), fibroelastosis (FE), and organizing pneumonia (OP), as potential histologic markers to distinguish between benign and malignant conditions. A total of 251 TBLB cases with defined benign and malignant outcomes based on clinical follow-up were collected and a gradient-boosted decision-tree-based machine learning model (XGBoost) was trained and tested on randomly split training and test sets.
RESULTS:Five pathological changes showed independent, mild-to-moderate associations (AUC ranging from 0.58 to 0.75) with benign conditions, with IB/B being the strongest predictor. On the other hand, FE emerged to be the sole indicator of malignant conditions with a mild association (AUC = 0.66). Our model was trained on 200 cases and tested on 51 cases, achieving an AUC of 0.78 for the binary classification of benign vs. malignant on the test set.
CONCLUSION:The machine-learning model developed has the potential to distinguish between benign and malignant conditions in TBLB samples excluding the presence or absence of tumor cells, thereby improving diagnostic accuracy and reducing the burden of repeated sampling procedures for patients.
更多查看译文
关键词
transbronchial lung biopsy (TBLB),non-diagnostic samples,Delphi method,interface bronchitis/bronchiolitis (IB/B),decision-tree based classifiers
AI 理解论文
溯源树
样例
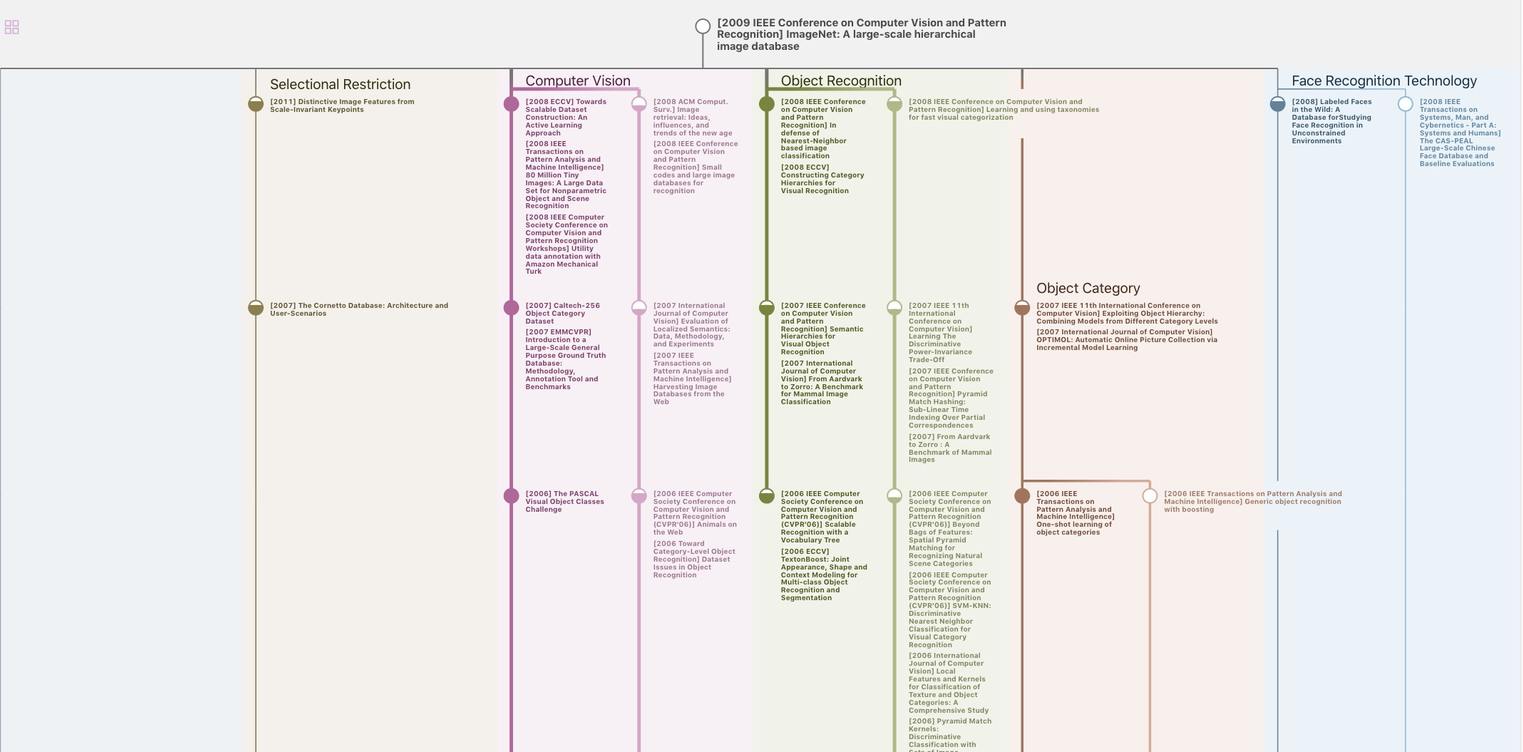
生成溯源树,研究论文发展脉络
Chat Paper
正在生成论文摘要