DVR Using Randomized Self-Structuring Fuzzy and Recurrent Probabilistic Fuzzy Neural-Based Controller
Journal of The Institution of Engineers (India): Series B(2024)
摘要
This article proposes a machine learning-based dynamic voltage restorer (DVR) control strategy for addressing the conventional design procedure of fuzzy logic that needs human expertise to decide membership functions. A randomized evolving Takagi–Sugeno (ReTSK) machine learning approach is proposed for the estimation of fundamental weight components from the polluted grid for enhanced DVR compensating capability. A recurrent probabilistic fuzzy neural network (RPFNN) control is employed for encountering the manual parameters tuning approach of a proportional-integral controller that depends on the optimized coefficients during severe voltage disturbances. The outlined approaches demonstrate robust performance by improving the DC- and AC-link voltage regulation with parametric variations and disturbances. The recommended RPFNN control provides a better response during the transitory state in terms of performance indicators like rise time (0.15 s), settle time (0.08 s), overshoot (3.3
更多查看译文
关键词
Compensating voltages,DC-link,Kernel Fuzzy C-means,Unbalanced,Rat Swarm Optimizer,THD,Sag
AI 理解论文
溯源树
样例
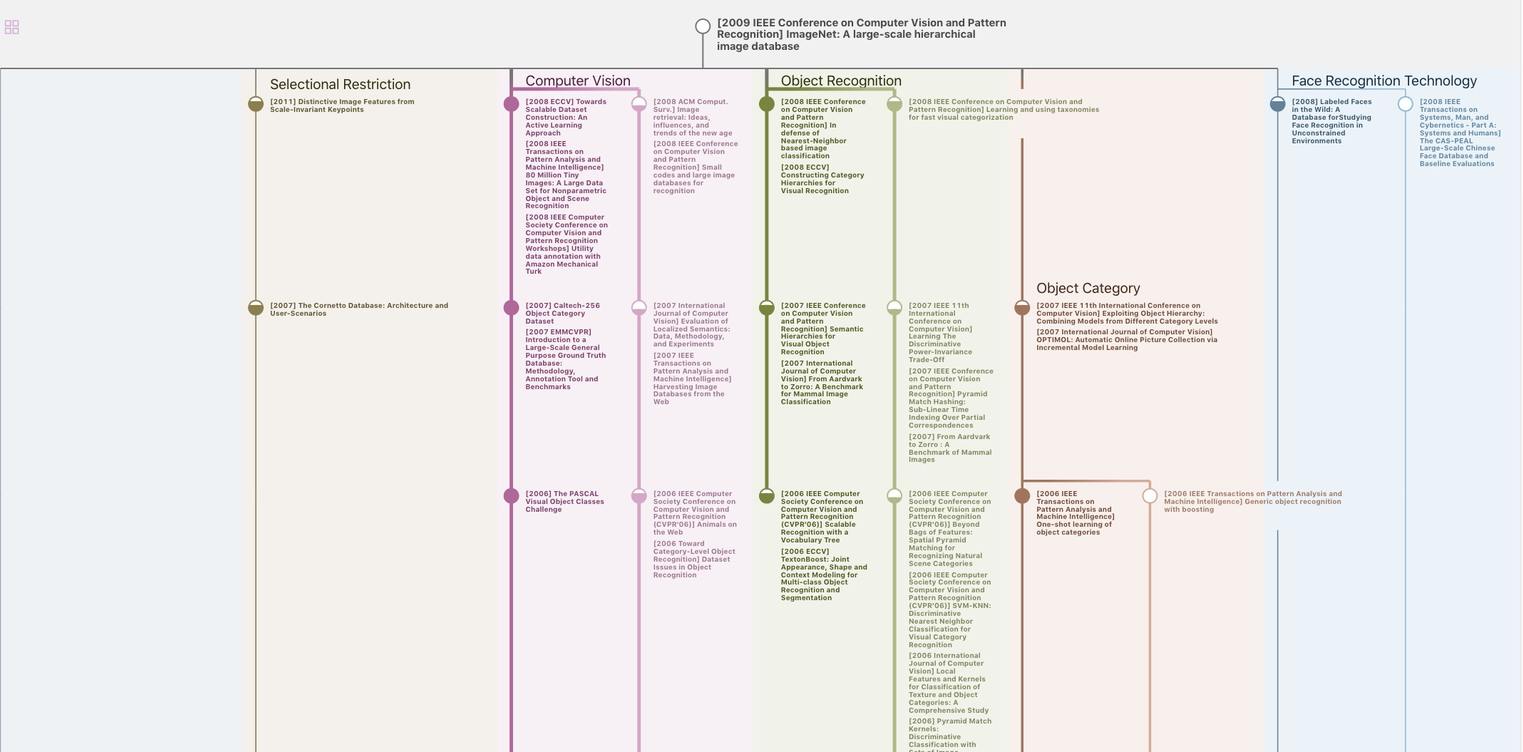
生成溯源树,研究论文发展脉络
Chat Paper
正在生成论文摘要