GHCL: Gaussian heuristic curriculum learning for Brain CT report generation
Multimedia Systems(2024)
摘要
Brain computed tomography (CT) report generation, which aims at generating accurate and descriptive reports for Brain CT imaging, has gained growing attention from researchers. Existing works mainly train a language-generation model with complex image-text pairs for supervision, which still struggled with the following challenges: 1) the serious long-tail distribution of textual supervise signals led by imbalanced text length distribution, and 2) the insufficient medical data caused by expensive expert intervention. In this paper, we propose a novel Gaussian heuristic curriculum learning (GHCL) model to effectively tackle the long-tail data distribution and optimally utilize the limited training data. Specifically, our training process mimics the learning process of physicians in a step-wise paradigm. Firstly, we evaluate the scores of training difficulty for each sample through two elaborately designed Gaussian heuristic metrics. Then, during the training of the language-generation model, we iteratively select the most suitable batch of training samples, which is comprehensively considered by the calculated scores of training difficulty. In this way, GHCL can effectively guide the progressive learning of the report generation model and boost the quality of generated Brain CT reports. We comprehensively compare the method with previous state-of-the-art models on the Brain CT report generation dataset BCT-CHR. Experimental results demonstrate that our method surpasses previous state-of-the-art approaches and GHCL is flexible to combine with existing approaches to further improve the performance.
更多查看译文
关键词
Brain CT report generation,Curriculum learning,Gaussian distribution
AI 理解论文
溯源树
样例
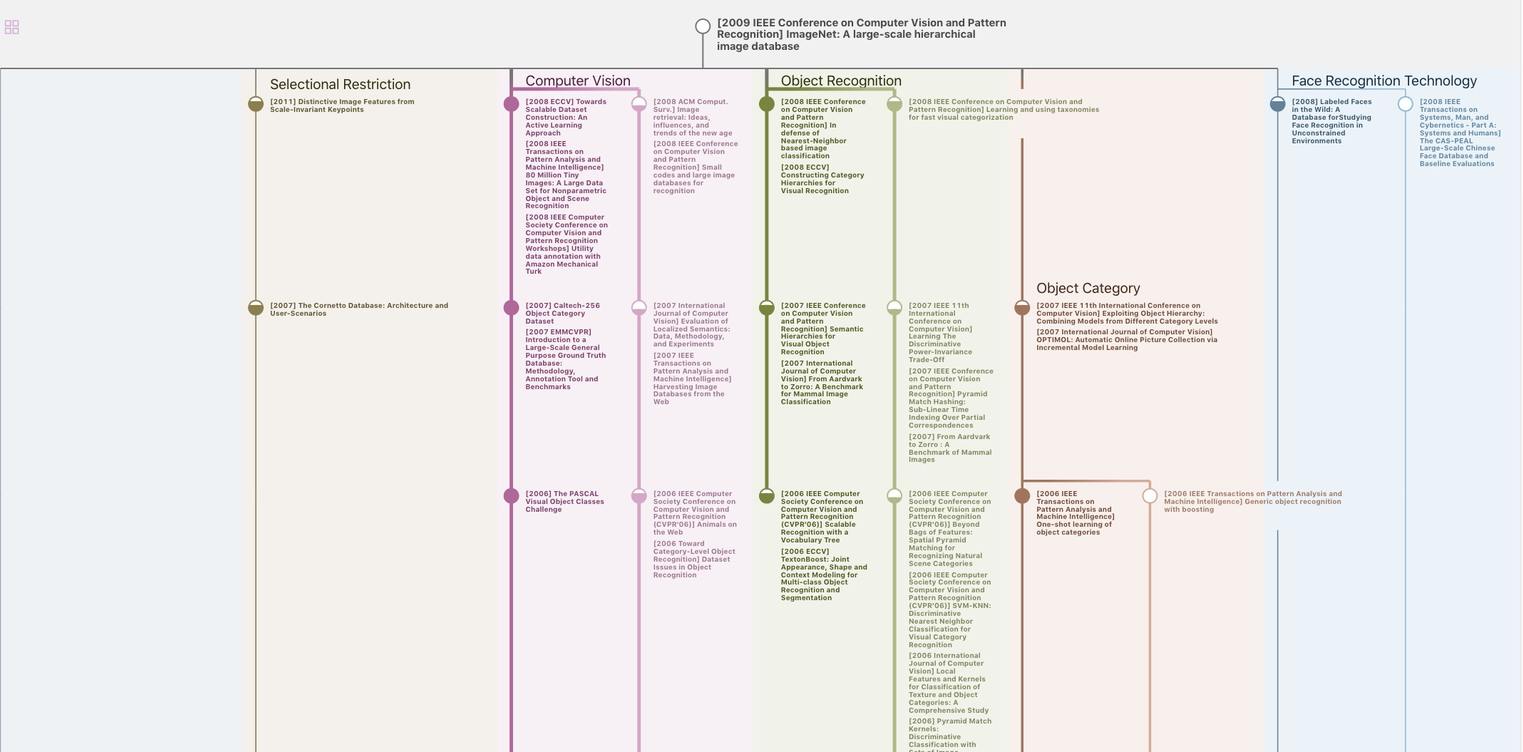
生成溯源树,研究论文发展脉络
Chat Paper
正在生成论文摘要