Ultra-low dose chest CT with silver filter and deep learning reconstruction significantly reduces radiation dose and retains quantitative information in the investigation and monitoring of lymphangioleiomyomatosis (LAM)
European Radiology(2024)
摘要
Frequent CT scans to quantify lung involvement in cystic lung disease increases radiation exposure. Beam shaping energy filters can optimize imaging properties at lower radiation dosages. The aim of this study is to investigate whether use of SilverBeam filter and deep learning reconstruction algorithm allows for reduced radiation dose chest CT scanning in patients with lymphangioleiomyomatosis (LAM). In a single-center prospective study, 60 consecutive patients with LAM underwent chest CT at standard and ultra-low radiation doses. Standard dose scan was performed with standard copper filter and ultra-low dose scan was performed with SilverBeam filter. Scans were reconstructed using a soft tissue kernel with deep learning reconstruction (AiCE) technique and using a soft tissue kernel with hybrid iterative reconstruction (AIDR3D). Cyst scores were quantified by semi-automated software. Signal-to-noise ratio (SNR) was calculated for each reconstruction. Data were analyzed by linear correlation, paired t-test, and Bland–Altman plots. Patients averaged 49.4 years and 100
更多查看译文
关键词
Deep learning,Radiation dosage,Tomography (X-ray computed),Lung diseases
AI 理解论文
溯源树
样例
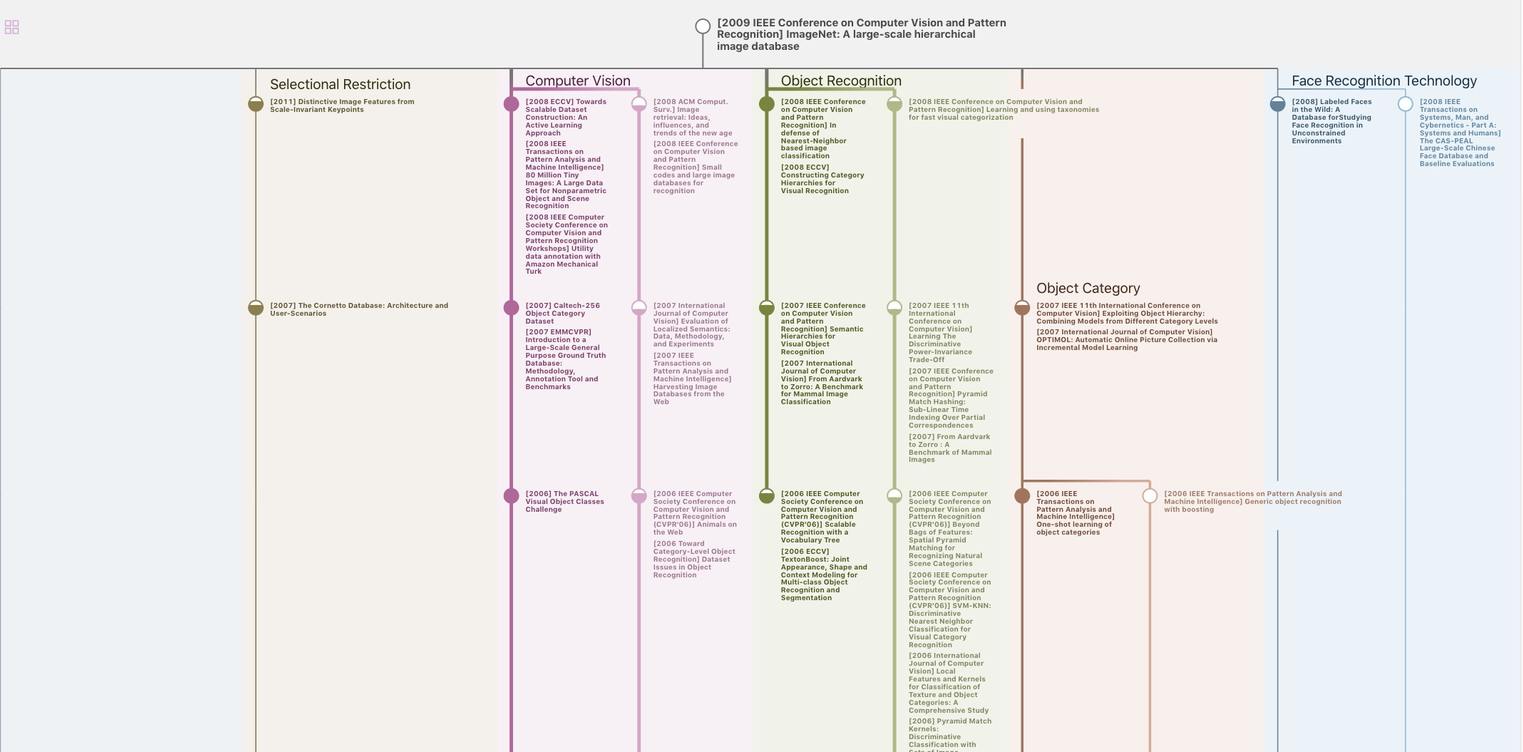
生成溯源树,研究论文发展脉络
Chat Paper
正在生成论文摘要