Complemented subspace-based weighted collaborative representation model for imbalanced learning
Applied Soft Computing(2024)
摘要
Collaborative representation-based classifiers (CRCs) have demonstrated remarkable classification performance in various pattern recognition fields. However, their success heavily relies on a balanced class distribution. More radically, significantly skewed class distributions may severely affect the effectiveness of the CRCs. To address this limitation, in this study, a complemented subspace-based regularization technique is integrated into the CRC framework for tackling imbalanced classification tasks. To enhance recognition accuracy for minority classes, a class weight learning algorithm is presented, in which the weight of each class is adaptively obtained according to the prior distribution information of the original data. Furthermore, the proposed model provides an efficient closed-form solution, ensuring computational efficiency comparable to CRCs. Extensive experiments on diverse imbalanced datasets are carried out to substantiate the superiority of our method over numerous advanced imbalanced learning algorithms.
更多查看译文
关键词
Imbalanced learning,Collaborative representation,Complemented subspace,Class weight,Regularization
AI 理解论文
溯源树
样例
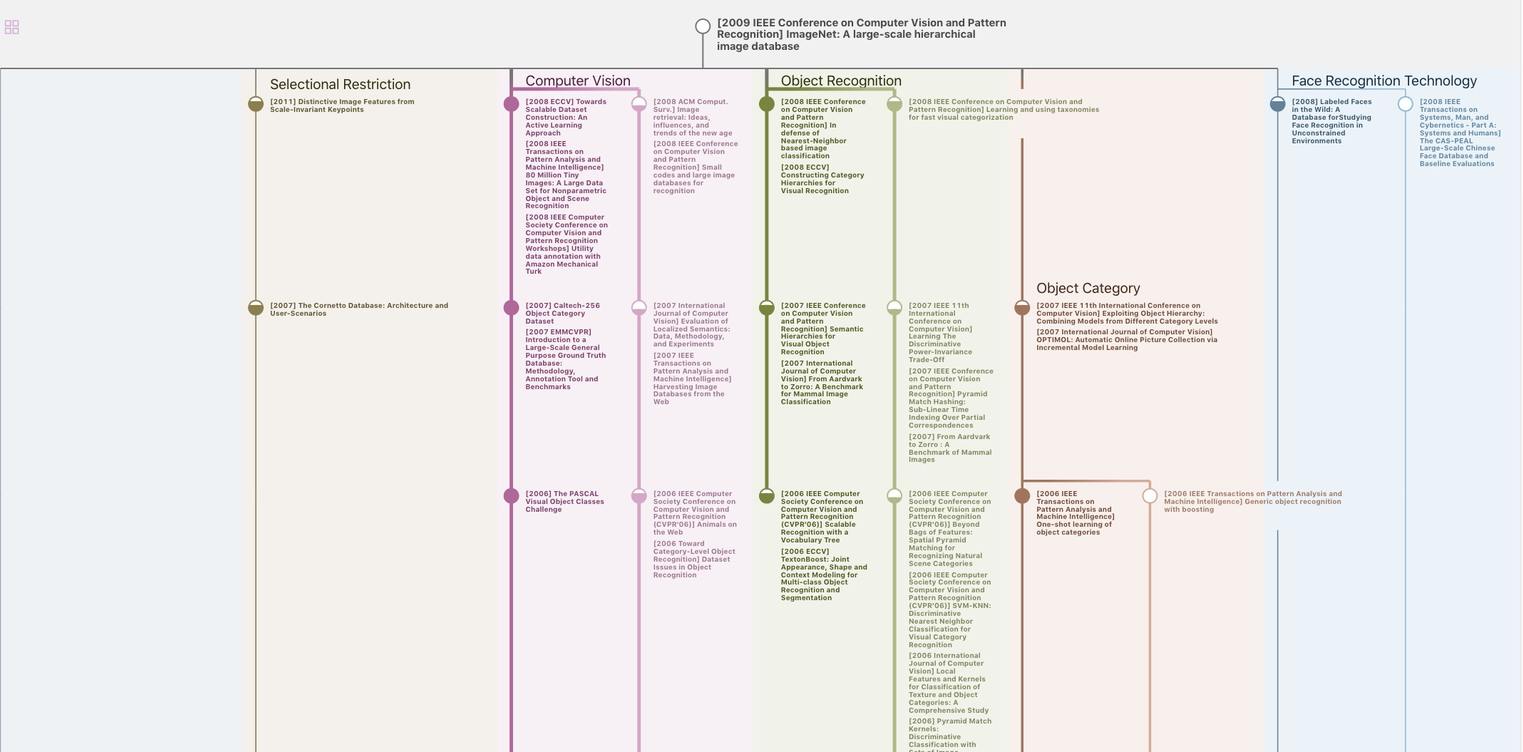
生成溯源树,研究论文发展脉络
Chat Paper
正在生成论文摘要