A feature optimization study based on a diabetes risk questionnaire
FRONTIERS IN PUBLIC HEALTH(2024)
摘要
Introduction The prevalence of diabetes, a common chronic disease, has shown a gradual increase, posing substantial burdens on both society and individuals. In order to enhance the effectiveness of diabetes risk prediction questionnaires, optimize the selection of characteristic variables, and raise awareness of diabetes risk among residents, this study utilizes survey data obtained from the risk factor monitoring system of the Centers for Disease Control and Prevention in the United States.Methods Following univariate analysis and meticulous screening, a more refined dataset was constructed. This dataset underwent preprocessing steps, including data distribution standardization, the application of the Synthetic Minority Oversampling Technique (SMOTE) in combination with the Round function for equilibration, and data standardization. Subsequently, machine learning (ML) techniques were employed, utilizing enumerated feature variables to evaluate the strength of the correlation among diabetes risk factors.Results The research findings effectively delineated the ranking of characteristic variables that significantly influence the risk of diabetes. Obesity emerges as the most impactful factor, overshadowing other risk factors. Additionally, psychological factors, advanced age, high cholesterol, high blood pressure, alcohol abuse, coronary heart disease or myocardial infarction, mobility difficulties, and low family income exhibit correlations with diabetes risk to varying degrees.Discussion The experimental data in this study illustrate that, while maintaining comparable accuracy, optimization of questionnaire variables and the number of questions can significantly enhance efficiency for subsequent follow-up and precise diabetes prevention. Moreover, the research methods employed in this study offer valuable insights into studying the risk correlation of other diseases, while the research results contribute to heightened societal awareness of populations at elevated risk of diabetes.
更多查看译文
关键词
diabetes,risk prediction,machine learning,feature enumeration,diabetes risk questionnaire,public health
AI 理解论文
溯源树
样例
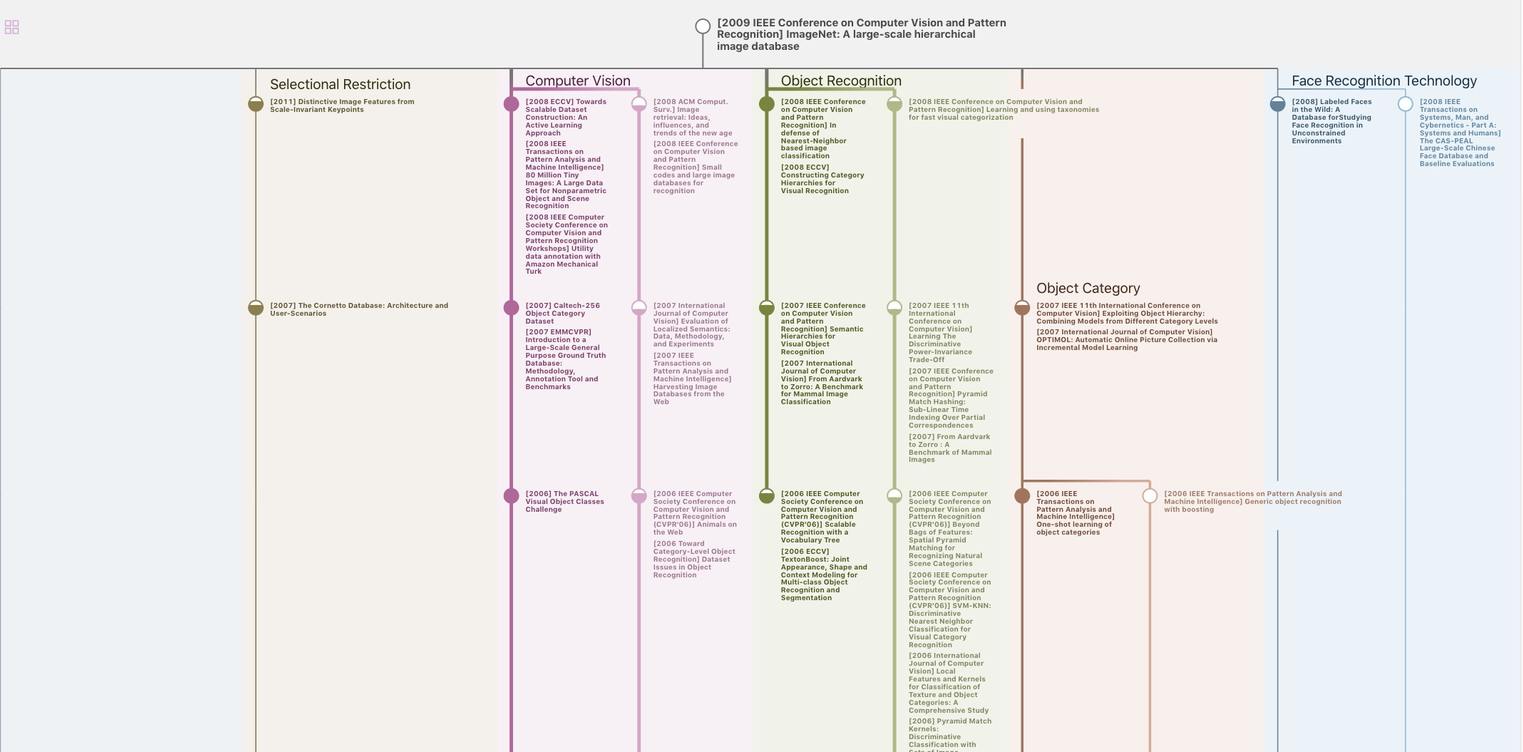
生成溯源树,研究论文发展脉络
Chat Paper
正在生成论文摘要