Global and Local Dual Fusion Network for Large-Ratio Cloud Occlusion Missing Information Reconstruction of a High-Resolution Remote Sensing Image
IEEE GEOSCIENCE AND REMOTE SENSING LETTERS(2024)
摘要
Large-ratio cloud occlusion significantly hampers the utilization of high-resolution remote sensing imagery. The existing reconstruction methods 1) overlook the problem of reconstructed and composite images sharing high- and low-level semantic and visual attributes in nonreconstructed regions, exacerbating the pronounced boundary effects; 2) neglect appearance discrepancies between reconstructed and nonreconstructed regions, leading to spectral degradation, and texture loss; and 3) overlook the problem of reconstructing large-ratio missing information. To address these issues, a global and local dual fusion network (GLDF-RecNet) is proposed in this study for large-ratio cloud occlusion removal in high-resolution remote sensing images. The global foreground-background aware attention module tackles shared high-level semantic features, whereas the local visual feature enhancement (LVRE) module addresses appearance differences. The GLDF-RecNet combines the Sobel and reconstruction loss functions for effective reconstruction by employing a two-stage fusion strategy. Compared to the classical recurrent feature reasoning network, spatiotemporal generator network (STGAN), spatial-temporal-spectral convolutional neural network (STS-CNN), and bishift network (BSN), the proposed model demonstrates superior quantitative and visual reconstruction outcomes for the 40%, 50%, and 70% missing ratios of Gaofen-1 (2 m).
更多查看译文
关键词
Image reconstruction,Feature extraction,Visualization,Semantics,Remote sensing,Kernel,Convolutional neural networks,Cloud occlusion,cloud removal,high resolution,missing information reconstruction
AI 理解论文
溯源树
样例
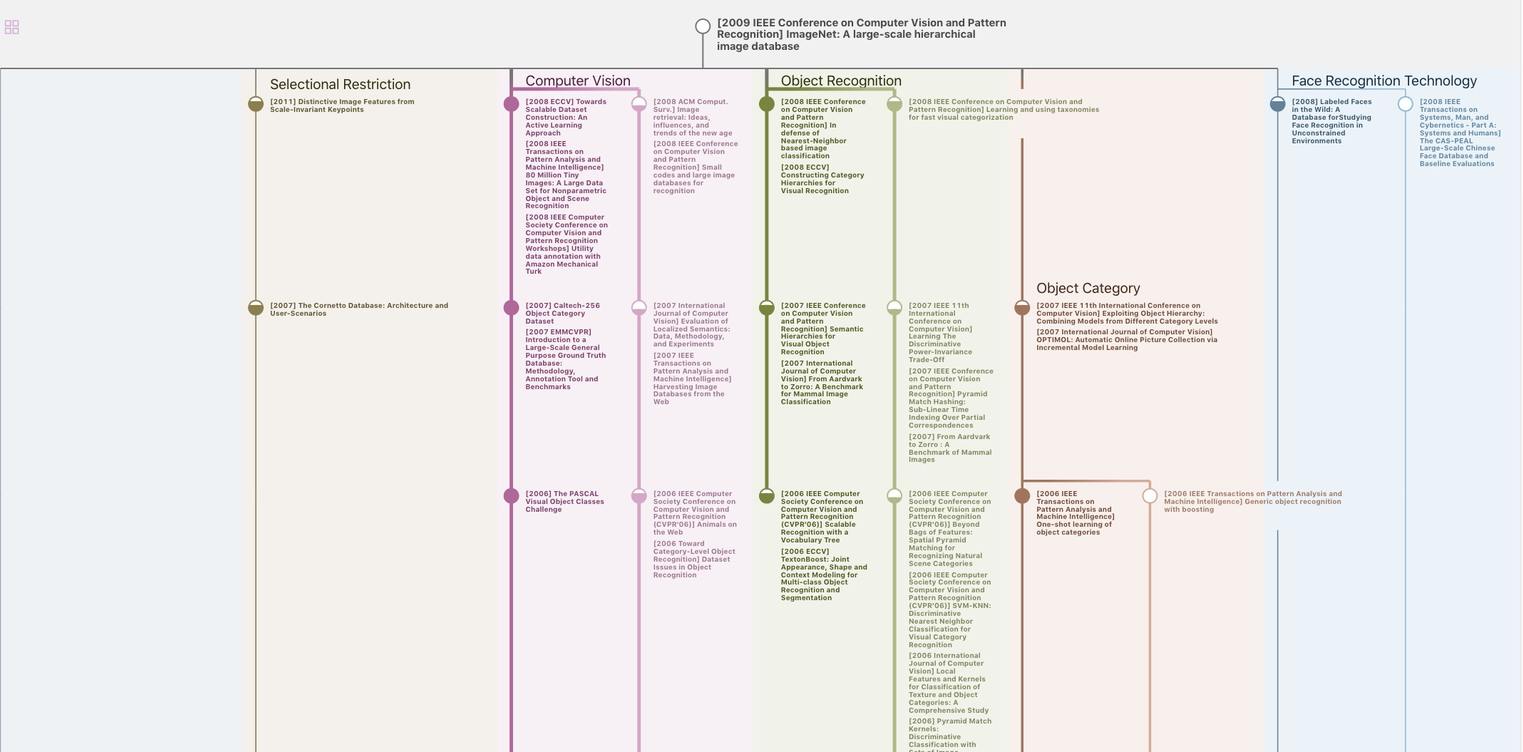
生成溯源树,研究论文发展脉络
Chat Paper
正在生成论文摘要