SCSNet: Sharpened Cosine Similarity-Based Neural Network for Hyperspectral Image Classification
IEEE GEOSCIENCE AND REMOTE SENSING LETTERS(2024)
摘要
Hyperspectral image classification (HSIC) faces challenges in preserving high-frequency features during down-sampling and hierarchical filtering in the CNN architecture. To overcome this, we propose sharpened cosine similarity (SCS) as an alternative to convolutions within a neural network for HSIC. SCSNet emphasizes parameter efficiency by bypassing nonlinear activation layers, normalization steps, and dropout post the SCS layer. Additionally, MaxAbsPool is implemented instead of MaxPool for superior performance. Experimental results on public HSI datasets demonstrate SCS's comparable accuracy, achieving 99% for both Indian Pines and Salinas datasets.
更多查看译文
关键词
Cosine similarity,hyperspectral image classification (HSIC),neural network (NN)
AI 理解论文
溯源树
样例
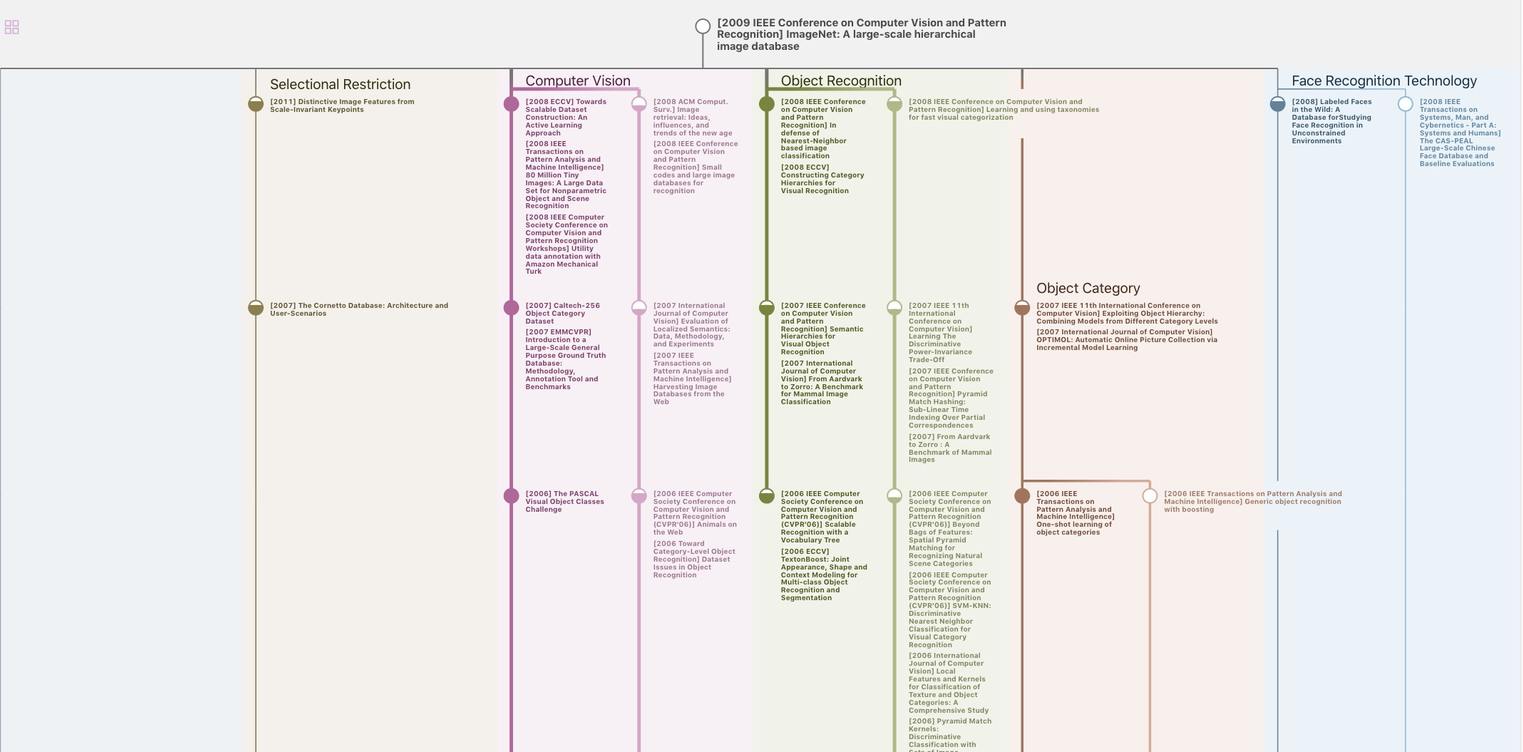
生成溯源树,研究论文发展脉络
Chat Paper
正在生成论文摘要