High-Speed Detector for Low-Powered Devices in Aerial Grasping
IEEE ROBOTICS AND AUTOMATION LETTERS(2024)
摘要
Autonomous aerial harvesting is a highly complex problem because it requires numerous interdisciplinary algorithms to be executed on mini low-powered computing devices. Object detection is one such algorithm that is compute-hungry. In this context, we make the following contributions: (i) Fast Fruit Detector (FFD), a resource-efficient, single-stage, and postprocessing-free object detector based on our novel latent object representation (LOR) module, query assignment, and prediction strategy. FFD achieves 100 FPS @ FP 32 precision on the latest 10 W NVIDIA Jetson-NX embedded device while co-existing with other time-critical sub-systems such as control, grasping, SLAM, a major achievement of this work, (ii) a method to generate vast amounts of training data without exhaustive manual labelling of fruit images since they consist of a large number of instances, which increases the labelling cost and time, and (iii) an open-source fruit detection dataset having plenty of very small-sized instances that are difficult to detect. Our exhaustive evaluations on our and MinneApple dataset show that FFD, being only a single-scale detector, is more accurate than many representative detectors, e.g. FFD is better than single-scale Faster-RCNN by 10.7 AP, multi-scale Faster-RCNN by 2.3 AP, and better than latest single-scale YOLO-v 8 by 8 AP and multi-scale YOLO-v 8 by 0.3 while being considerably faster.
更多查看译文
关键词
Aerial systems: applications,agricultural automation,deep learning for visual perception
AI 理解论文
溯源树
样例
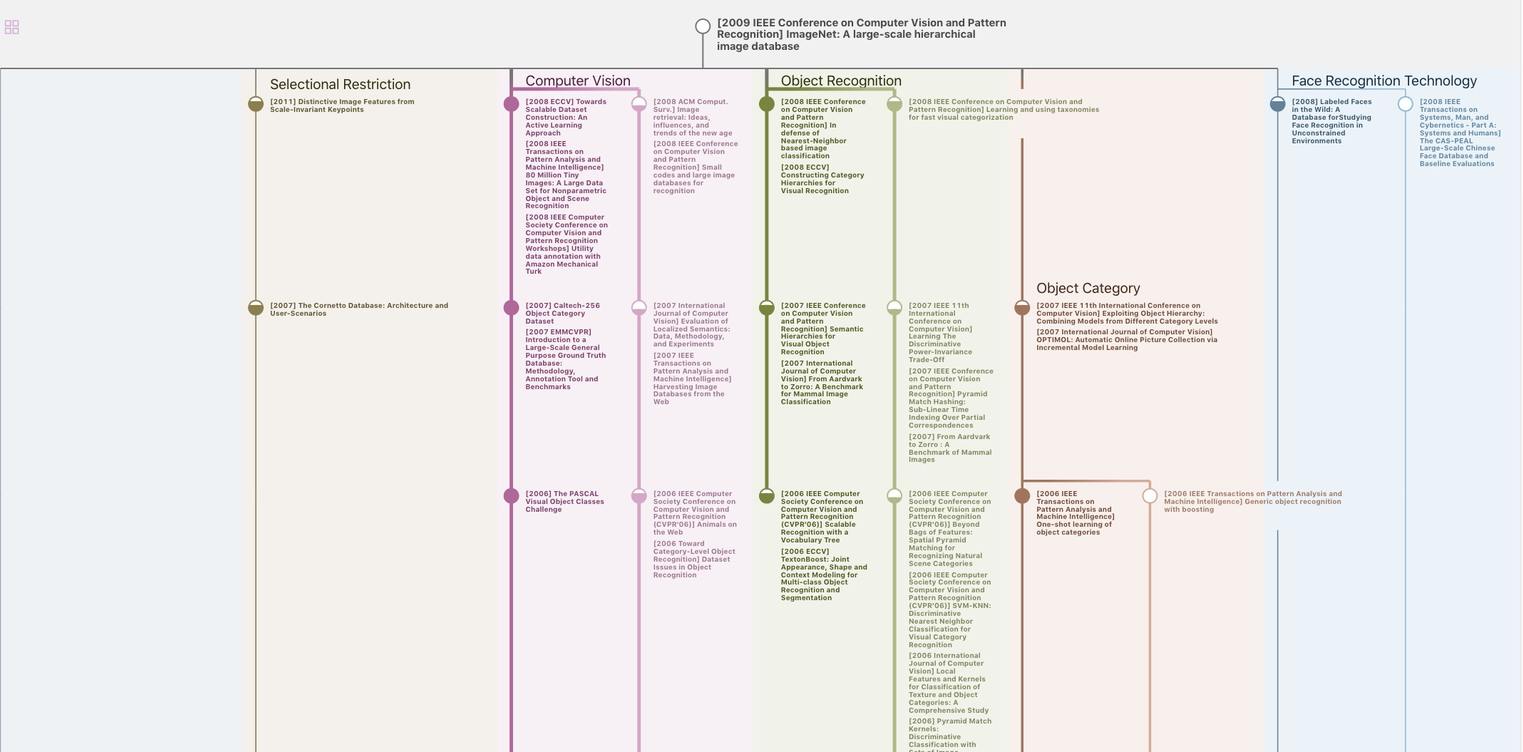
生成溯源树,研究论文发展脉络
Chat Paper
正在生成论文摘要