Recommender for Its Purpose: Repeat and Exploration in Food Delivery Recommendations
CoRR(2024)
摘要
Recommender systems have been widely used for various scenarios, such as
e-commerce, news, and music, providing online contents to help and enrich
users' daily life. Different scenarios hold distinct and unique
characteristics, calling for domain-specific investigations and corresponding
designed recommender systems. Therefore, in this paper, we focus on food
delivery recommendations to unveil unique features in this domain, where users
order food online and enjoy their meals shortly after delivery. We first
conduct an in-depth analysis on food delivery datasets. The analysis shows that
repeat orders are prevalent for both users and stores, and situations'
differently influence repeat and exploration consumption in the food delivery
recommender systems. Moreover, we revisit the ability of existing
situation-aware methods for repeat and exploration recommendations
respectively, and find them unable to effectively solve both tasks
simultaneously. Based on the analysis and experiments, we have designed two
separate recommendation models – ReRec for repeat orders and ExpRec for
exploration orders; both are simple in their design and computation. We conduct
experiments on three real-world food delivery datasets, and our proposed models
outperform various types of baselines on repeat, exploration, and combined
recommendation tasks. This paper emphasizes the importance of dedicated
analyses and methods for domain-specific characteristics for the recommender
system studies.
更多查看译文
AI 理解论文
溯源树
样例
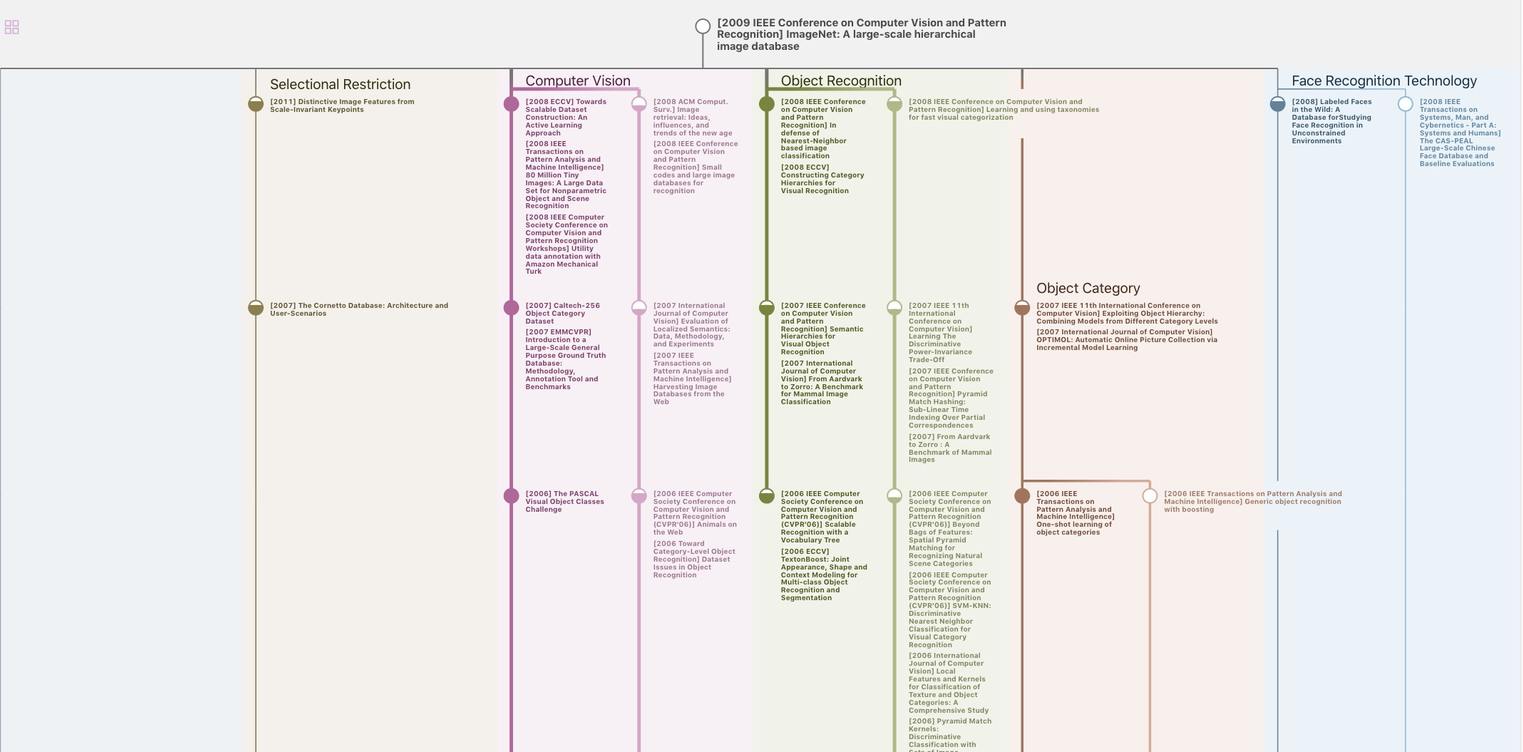
生成溯源树,研究论文发展脉络
Chat Paper
正在生成论文摘要