Text me the data: Generating Ground Pressure Sequence from Textual Descriptions for HAR
CoRR(2024)
摘要
In human activity recognition (HAR), the availability of substantial ground
truth is necessary for training efficient models. However, acquiring ground
pressure data through physical sensors itself can be cost-prohibitive,
time-consuming. To address this critical need, we introduce Text-to-Pressure
(T2P), a framework designed to generate extensive ground pressure sequences
from textual descriptions of human activities using deep learning techniques.
We show that the combination of vector quantization of sensor data along with
simple text conditioned auto regressive strategy allows us to obtain
high-quality generated pressure sequences from textual descriptions with the
help of discrete latent correlation between text and pressure maps. We achieved
comparable performance on the consistency between text and generated motion
with an R squared value of 0.722, Masked R squared value of 0.892, and FID
score of 1.83. Additionally, we trained a HAR model with the the synthesized
data and evaluated it on pressure dynamics collected by a real pressure sensor
which is on par with a model trained on only real data. Combining both real and
synthesized training data increases the overall macro F1 score by 5.9 percent.
更多查看译文
关键词
HAR,Generative model,GPT,Pressure Sensor,Synthetic dataset
AI 理解论文
溯源树
样例
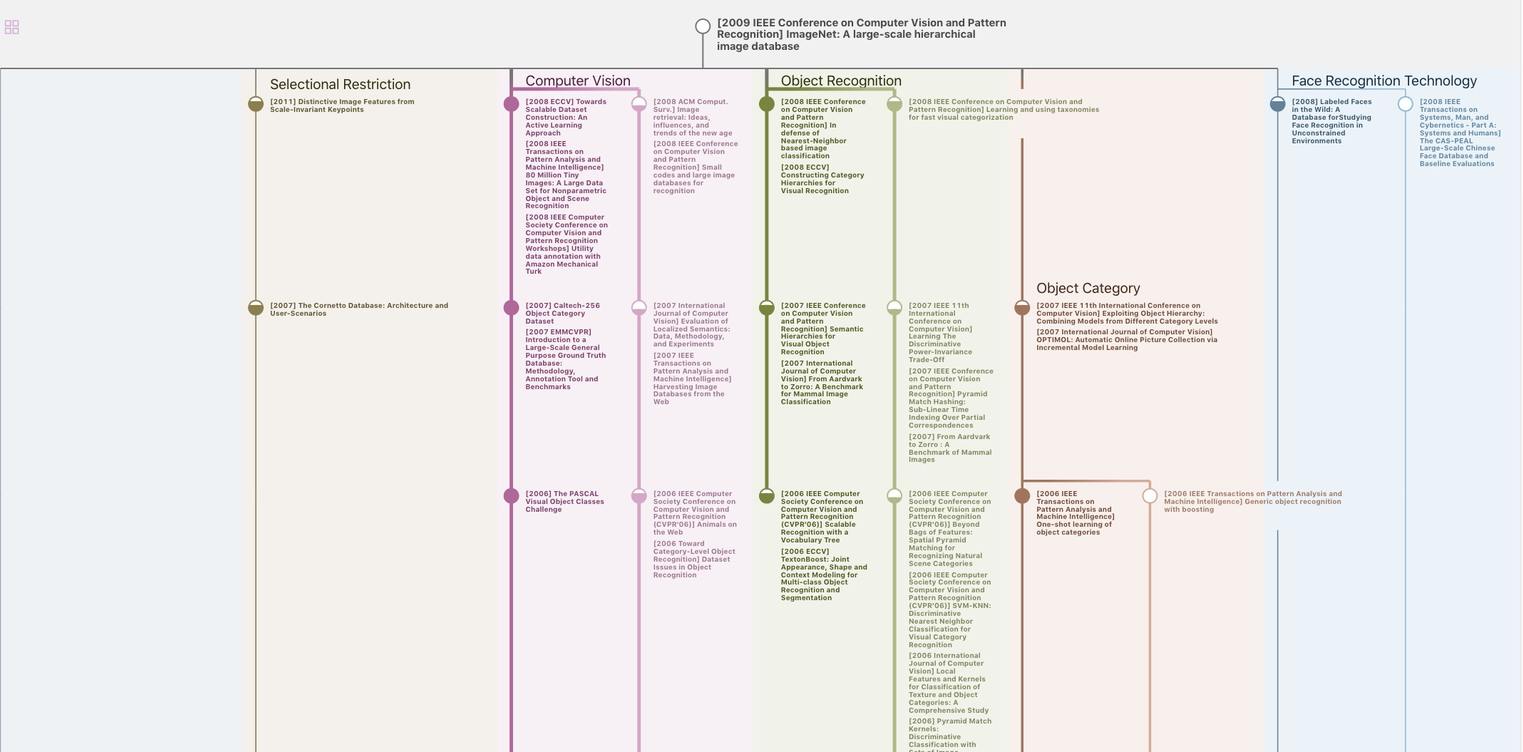
生成溯源树,研究论文发展脉络
Chat Paper
正在生成论文摘要