Transformer-based Spatial-Temporal Graph Attention Network for Traffic Flow Prediction.
2023 International Conference on Cyber-Enabled Distributed Computing and Knowledge Discovery (CyberC)(2023)
摘要
Traffic flow prediction, which plays an important role in intelligent traffic systems, has become a pressing problem to be addressed with the continuous development of smart cities. Currently, the fundamental obstacle lies in effectively modelling the complex spatial-temporal dependencies present in traffic flow data. Deep learning models such as Graph Neural Network based models and Transformer based models have shown promising results in this field. However, methods founded on a single model or framework have one significant limitation: Such methods cannot adequately represent the spatial and temporal features of traffic flow data, restricting the model’s ability to learn the dynamics of urban transportation. In this paper, we propose a transformer-based spatial-temporal graph attention network model called TSTGAT for traffic flow prediction, which integrates Transformer and Graph Attention Network. Experiments on two real-world traffic datasets from the Caltrans Performance Measurement System (PeMS) demonstrate that the proposed TSTGAT model outperforms well-known baselines.
更多查看译文
关键词
Traffic flow prediction,transformer,graph neural network,deep learning
AI 理解论文
溯源树
样例
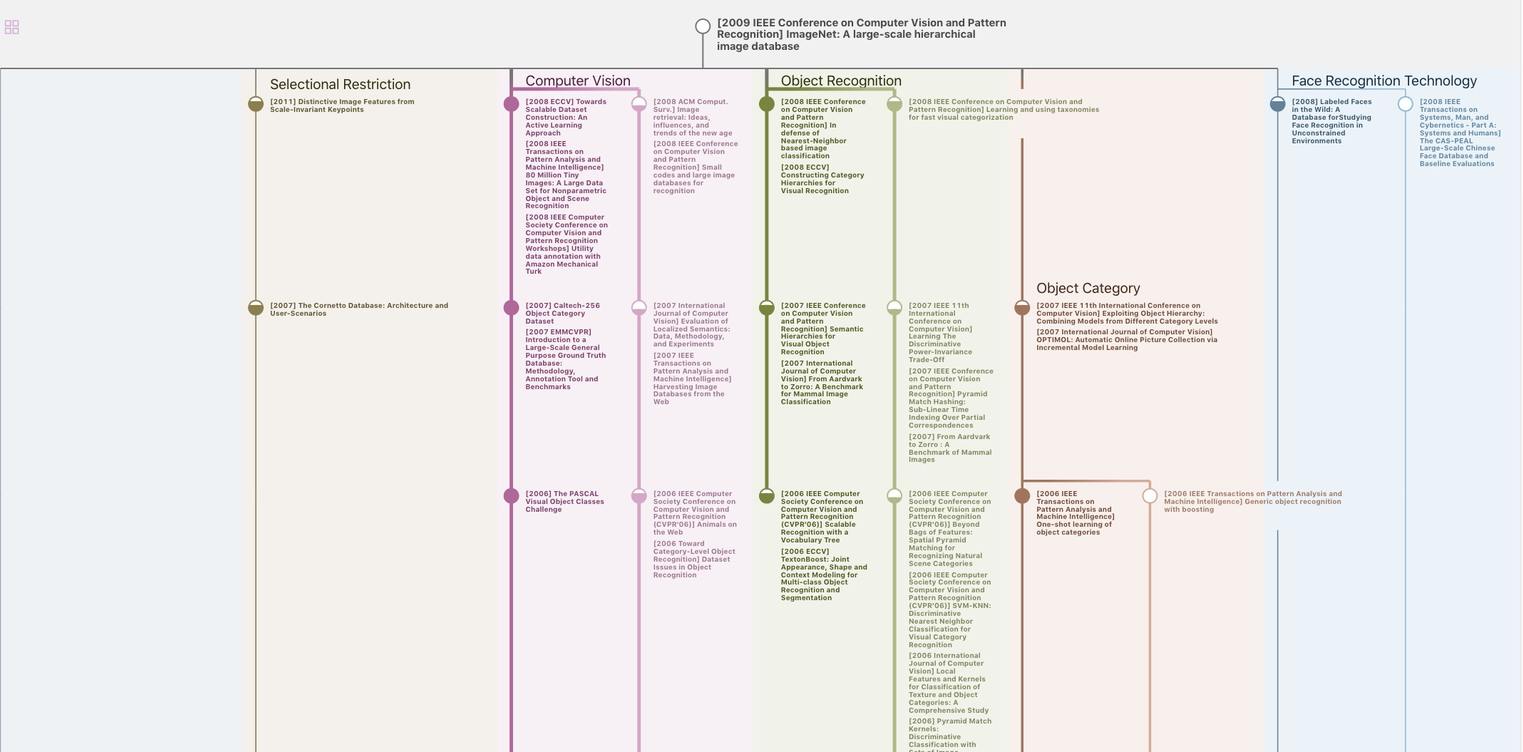
生成溯源树,研究论文发展脉络
Chat Paper
正在生成论文摘要