Indoor tracking using auxiliary particle filter and deep learning in wireless sensor networks
AD HOC NETWORKS(2024)
摘要
The low-cost received signal strength indicators (RSSIs) are commonly employed in indoor tracking using various state estimation methods, including particle filters (PFs). In the context of target tracking, PFs often employ the constant velocity (CV) and the constant acceleration (CA) models as transition models. This paper proposes an alternative model that replaces the CV with a stacked long short-term memory (LSTM) model. The trained stacked LSTM model is the transition function in the two-step auxiliary sampling importance resampling (TS-ASIR) filter. TS-ASIR filter introduces a two-time step transition model derived from sequential importance sampling (SIS). This filter is characterized by two auxiliary variables representing indices from the previous steps. Besides, the generalized regression neural network (GRNN) is employed for position estimation, utilizing RSSI measurements in an environment with high noise levels. Furthermore, map -aiding (MA) is integrated with the TS-ASIR to reduce localization and tracking errors on a 2-D plane. The MA improves the accuracy by updating particle weights according to a digital map, independently of infrastructure. The MA method updates particle weights twice for the TS-ASIR filter. Notably, when assessing the root mean square error (RMSE), the GRNN+TS-ASIR-LSTM-MA approach demonstrates superior accuracy compared to the filtered estimated GRNN position by the unscented Kalman filter (UKF) that was implemented using the CV model, with improvements of 38.20% and 57.12% in the two considered scenarios.
更多查看译文
关键词
Received signal strength indicator (RSSI),Wireless sensors network (WSN),Indoor tracking,Long short-time memory (LSTM),Auxiliary particle filter (APF),Map-aiding (MA)
AI 理解论文
溯源树
样例
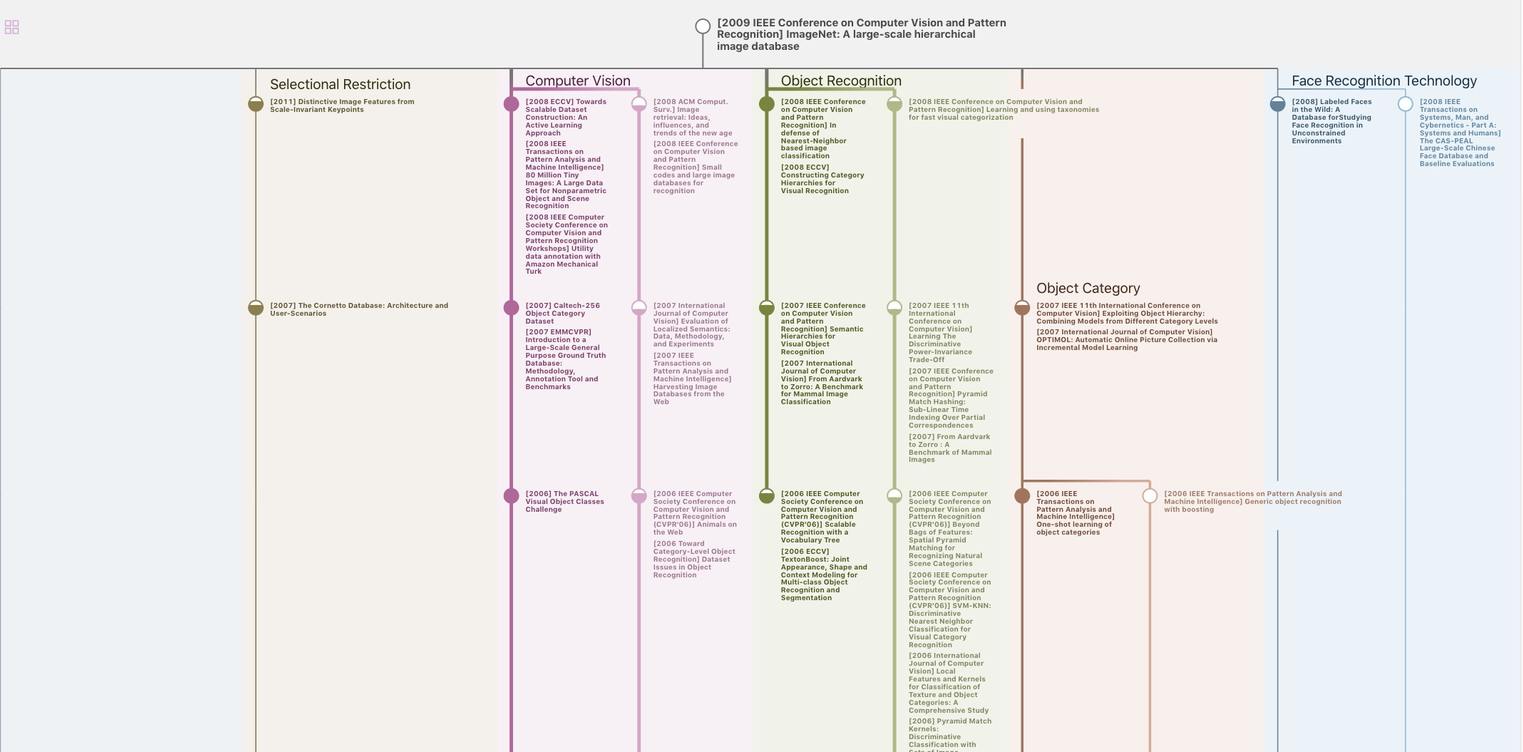
生成溯源树,研究论文发展脉络
Chat Paper
正在生成论文摘要