End-to-end pseudo relevance feedback based vertical web search queries recommendation
Multimedia Tools and Applications(2024)
摘要
Nowadays, the web has emerged as an enormous multimedia data resource. Social media platforms are becoming the mass producers of user-generated multimedia content. Web search engines usually organize media-specific information, such as text, images, video, etc., in specialized data repositories (verticals), providing easy access to multimedia content. Web search engines have become efficient in retrieving data across various distinct verticals. However, users still need help formulating queries to retrieve the relevant multimedia results from verticals. Novice users often issue short-length ambiguous queries due to a lack of domain knowledge or query formulation experience, resulting in the retrieval of irrelevant results. The query formulation itself is a time-consuming process for the users. We presented an end-to-end deep-learning automatic query recommendation approach to address the associated issues. The proposed method autonomously extracts the domain knowledge using pseudo-relevance feedback, transforms it into a unified text-to-text summary, and assists users in generating non-ambiguous and well-balanced query recommendations. The proposed system employs Google’s real-time dataset and is compared to the Google search engine. The evaluation consists of empirical and usability perspectives. The empirical evaluation of shorter query formulation and reformulation time obtained 89
更多查看译文
关键词
Deep learning,Query recommendation,Multimedia information retrieval,Natural language processing,Vertical web search,Pseudo relevance feedback
AI 理解论文
溯源树
样例
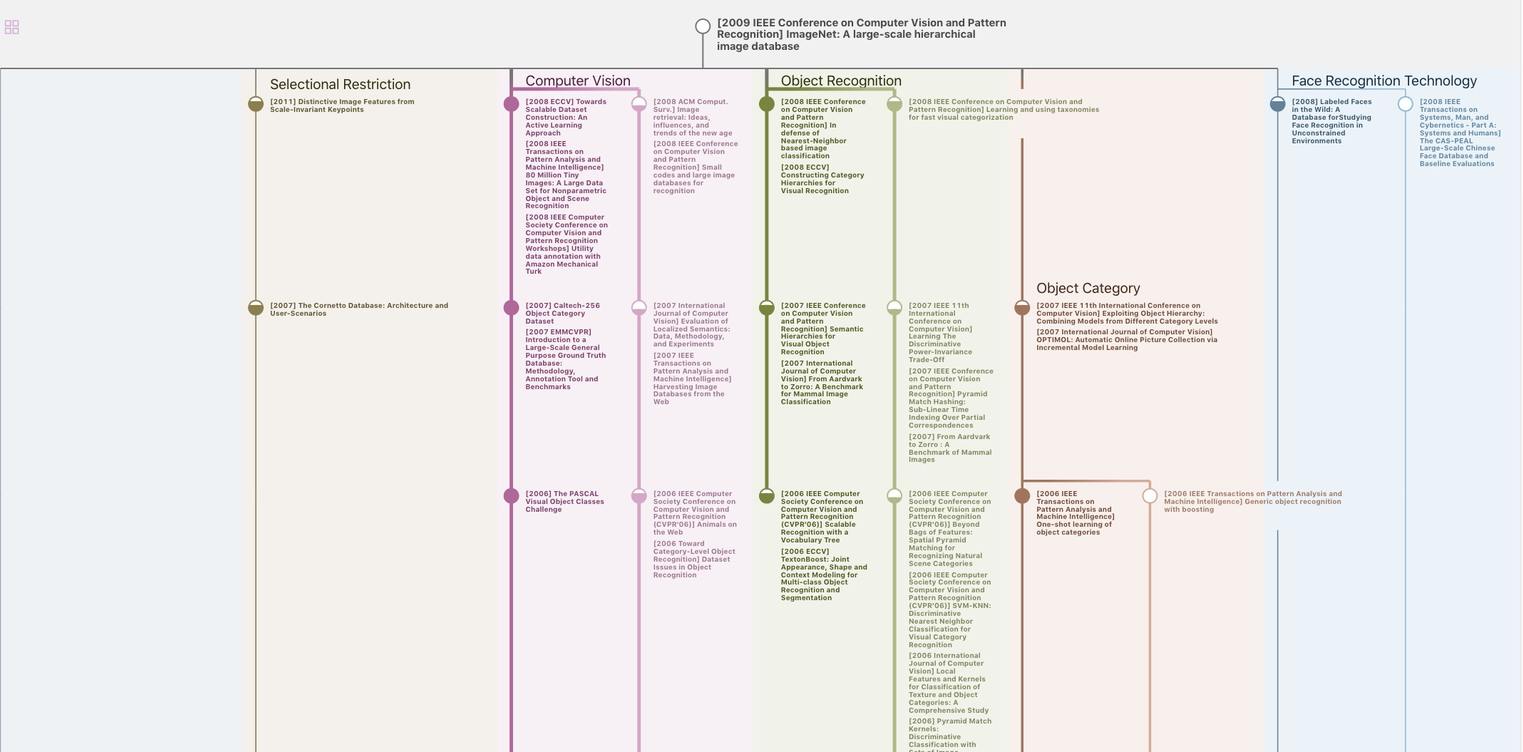
生成溯源树,研究论文发展脉络
Chat Paper
正在生成论文摘要