Learning to Retrieve for Job Matching
CoRR(2024)
摘要
Web-scale search systems typically tackle the scalability challenge with a
two-step paradigm: retrieval and ranking. The retrieval step, also known as
candidate selection, often involves extracting standardized entities, creating
an inverted index, and performing term matching for retrieval. Such traditional
methods require manual and time-consuming development of query models. In this
paper, we discuss applying learning-to-retrieve technology to enhance LinkedIns
job search and recommendation systems. In the realm of promoted jobs, the key
objective is to improve the quality of applicants, thereby delivering value to
recruiter customers. To achieve this, we leverage confirmed hire data to
construct a graph that evaluates a seeker's qualification for a job, and
utilize learned links for retrieval. Our learned model is easy to explain,
debug, and adjust. On the other hand, the focus for organic jobs is to optimize
seeker engagement. We accomplished this by training embeddings for personalized
retrieval, fortified by a set of rules derived from the categorization of
member feedback. In addition to a solution based on a conventional inverted
index, we developed an on-GPU solution capable of supporting both KNN and term
matching efficiently.
更多查看译文
AI 理解论文
溯源树
样例
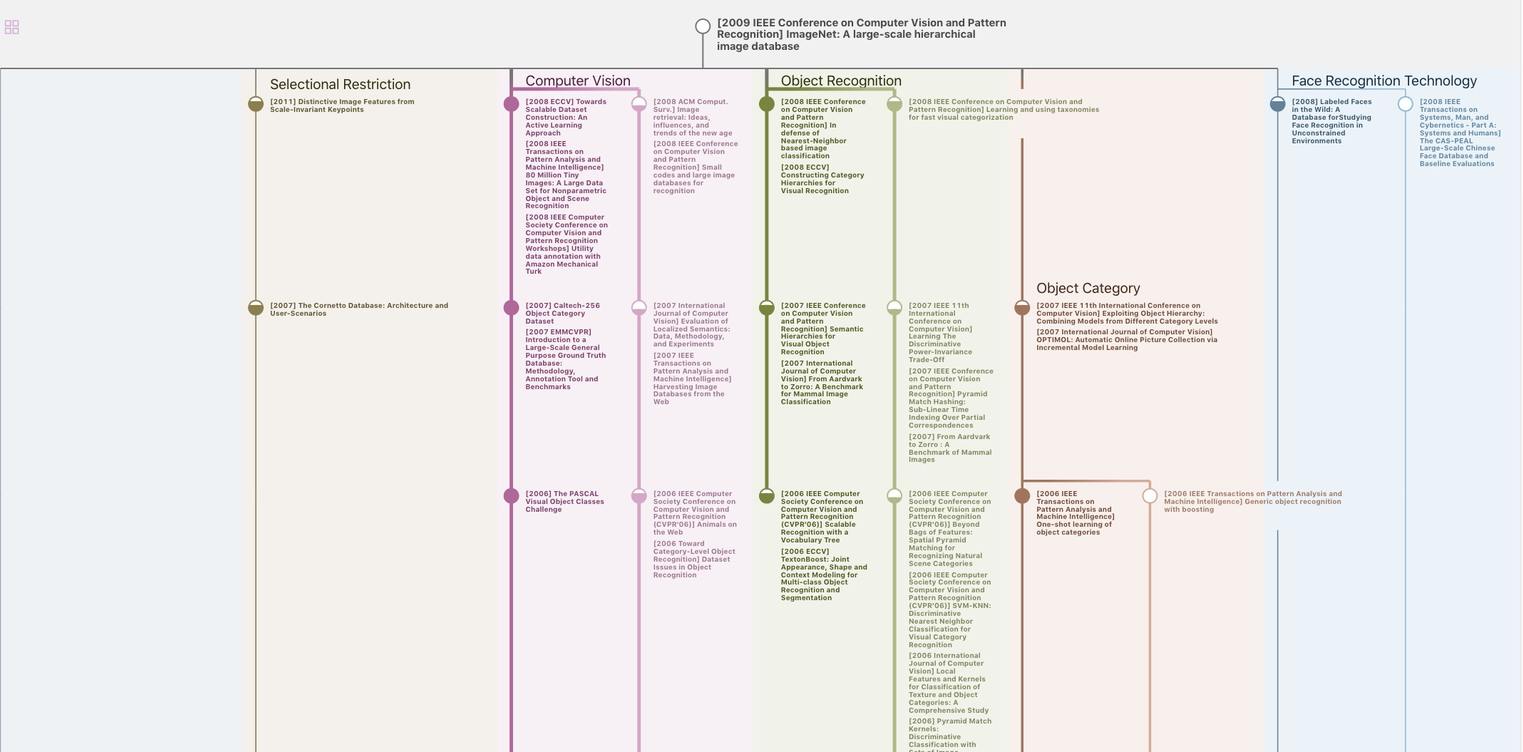
生成溯源树,研究论文发展脉络
Chat Paper
正在生成论文摘要