Improved Syndrome-based Neural Decoder for Linear Block Codes
GLOBECOM 2023 - 2023 IEEE Global Communications Conference(2024)
摘要
In this work, we investigate the problem of neural-based error correction
decoding, and more specifically, the new so-called syndrome-based decoding
technique introduced to tackle scalability in the training phase for larger
code sizes. We improve on previous works in terms of allowing full decoding of
the message rather than codewords, allowing thus the application to
non-systematic codes, and proving that the single-message training property is
still viable. The suggested system is implemented and tested on polar codes of
sizes (64,32) and (128,64), and a BCH of size (63,51), leading to a significant
improvement in both Bit Error Rate (BER) and Frame Error Rate (FER), with gains
between 0.3dB and 1dB for the implemented codes in the high Signal-to-Noise
Ratio (SNR) regime.
更多查看译文
关键词
Linear Code,Neural Decoding,Linear Block Codes,Signal-to-noise,Bit Error Rate,Bit Error,Systematic Coding,Code Size,Polar Codes,Neural Network,Machine Learning,Random Variables,High-dimensional,Dimensional Space,White Noise,Additive Noise,Random Noise,Recurrent Neural Network,Generator Matrix,Information Bits,Decoder Output,Parity-check,Gated Recurrent Unit,Forward Error Correction,Maximum A Posteriori,Original Message,Machine Learning Solutions
AI 理解论文
溯源树
样例
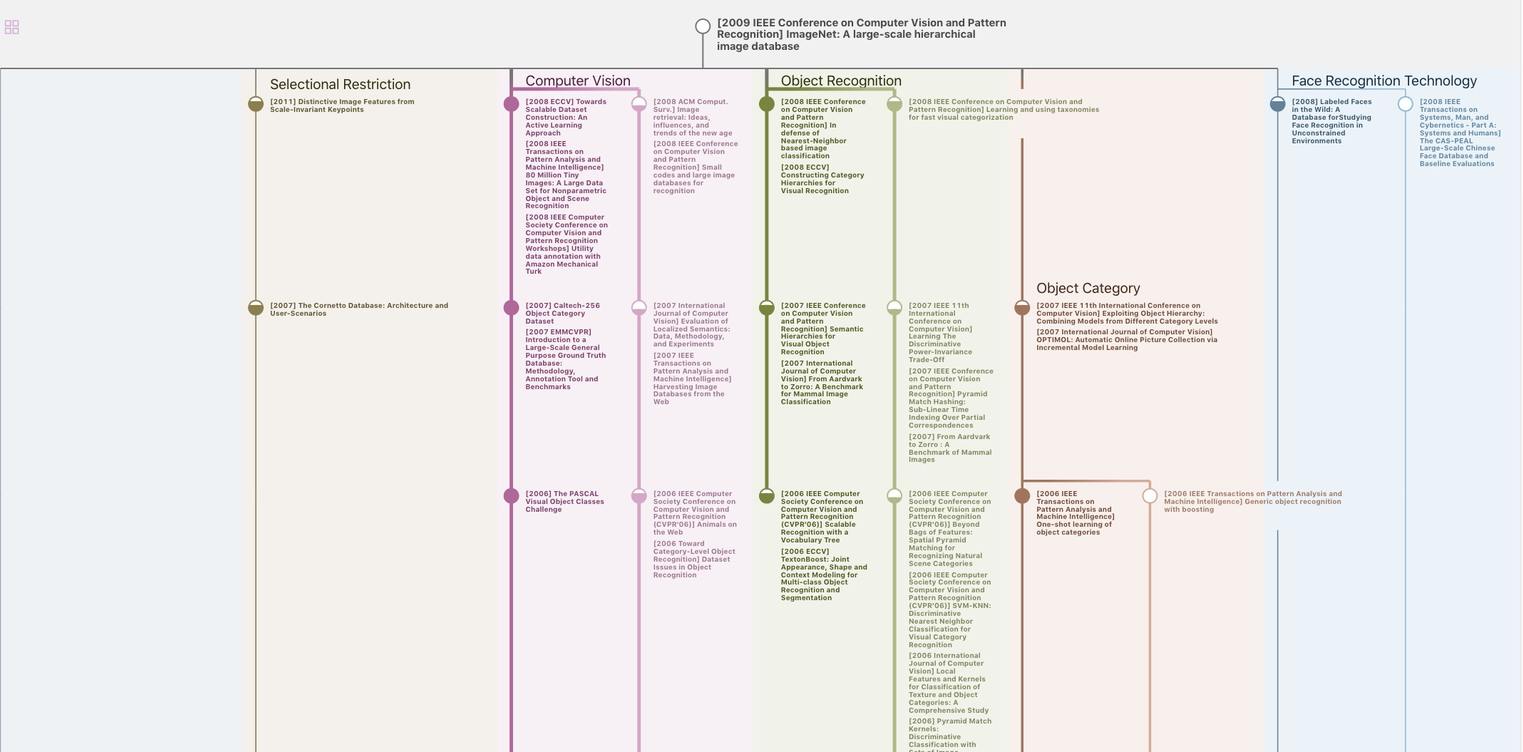
生成溯源树,研究论文发展脉络
Chat Paper
正在生成论文摘要