Combining unsupervised and supervised learning in microscopy enables defect analysis of a full 4H-SiC wafer
MRS Communications(2024)
摘要
Detecting and analyzing various defect types in semiconductor materials is an important prerequisite for understanding the underlying mechanisms and tailoring the production processes. Analysis of microscopy images that reveal defects typically requires image analysis tasks such as segmentation and object detection. With the permanently increasing amount of data from experiments, handling these tasks manually becomes more and more impossible. In this work, we combine various image analysis and data mining techniques to create a robust and accurate, automated image analysis pipeline for extracting the type and position of all defects in a microscopy image of a KOH-etched 4H-SiC wafer.
更多查看译文
关键词
Machine learning,Dislocations
AI 理解论文
溯源树
样例
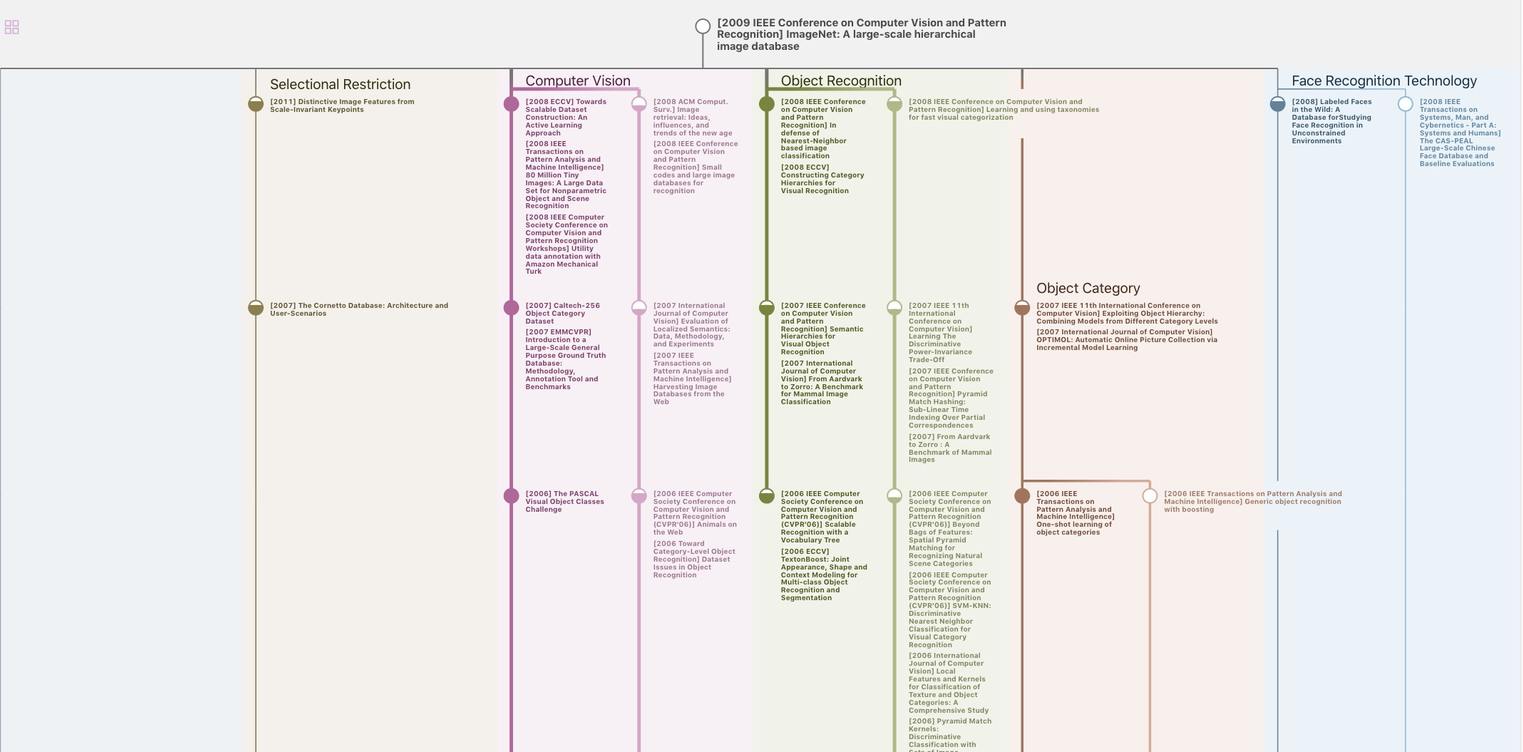
生成溯源树,研究论文发展脉络
Chat Paper
正在生成论文摘要