Statistical Analyses of Solar Prominences and Active Region Features in 304 ? Filtergrams Detected via Deep Learning
ASTROPHYSICAL JOURNAL SUPPLEMENT SERIES(2024)
摘要
Solar active regions (ARs) are areas on the Sun with very strong magnetic fields where various activities take place. Prominences are one of the typical solar features in the solar atmosphere, whose eruptions often lead to solar flares and coronal mass ejections. Therefore, studying their morphological features and their relationship with solar activity is useful in predicting eruptive events and in understanding the long-term evolution of solar activities. A huge amount of data have been collected from various ground-based telescopes and satellites. The massive amounts of data make human inspection difficult. For this purpose, we developed an automated detection method for prominences and ARs above the solar limb based on deep-learning techniques. We applied it to process the 304 & Aring; data obtained by SDO/AIA from 2010 May 13 to 2020 December 31. Besides the butterfly diagrams and latitudinal migrations of the prominences and ARs during solar cycle 24, the variations of their morphological features (such as the locations, areas, heights, and widths) with the calendar years and the latitude bands were analyzed. Most of these statistical results based on our new method are in agreement with previous studies, which also guarantees the validity of our method. The N-S asymmetry indices of the prominences and ARs show that the northern hemisphere is the dominant hemisphere in solar cycle 24, except for 2012-2015, and 2020 for ARs. The high-latitude prominences show much stronger N-S asymmetry, where the northern hemisphere is dominant in similar to 2011 and similar to 2015, and the southern hemisphere is dominant from 2016-2019.
更多查看译文
关键词
Solar prominences,Solar active regions,Convolutional neural networks,Solar cycle
AI 理解论文
溯源树
样例
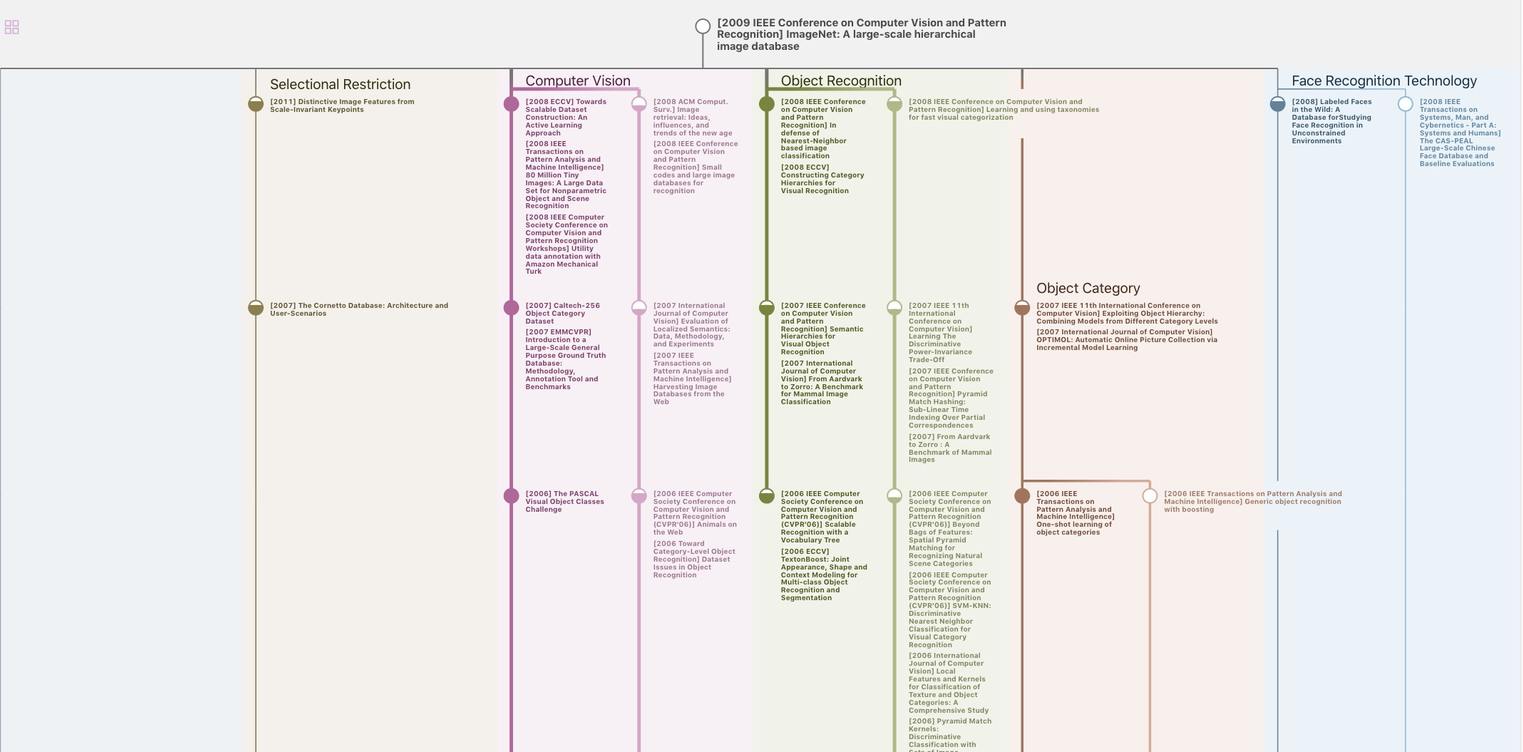
生成溯源树,研究论文发展脉络
Chat Paper
正在生成论文摘要