A Quantum-inspired Approach to Pattern Recognition and Machine Learning. Part I
International Journal of Theoretical Physics(2024)
摘要
How are abstract concepts formed and recognized on the basis of a previous experience? It is interesting to compare the behavior of human minds and of artificial intelligences with respect to this problem. Generally, a human mind that abstracts a concept (say, table ), from a given set of known examples creates a table-Gestalt : a kind of vague and out of focus image that does not fully correspond to a particular table with well determined features. Can the construction of a gestaltic pattern (that is so natural for human minds) be taught to an intelligent machine? This problem can be successfully discussed in the framework of a quantum-inspired approach to pattern recognition and to machine learning. The basic idea is replacing classical datasets with quantum datasets where objects are described by special quantum states, involving the characteristic uncertainty and ambiguity of the quantum theoretic formalism. In this framework, the intuitive concept of Gestalt can be simulated by the mathematical concept of positive centroid of a given quantum dataset . Accordingly, the crucial problem “how can we classify a new object on the basis of a previous experience?” can be dealt with in terms of some special quantum similarity-relations that may hold between the new object’s state and the positive centroid of the quantum dataset under consideration. This allows us to define a particular quantum classifier, called fidelity-classifier , that admits the possibility of uncertain answers . Although recognition procedures are different for human and for artificial intelligences, there is a common method of “facing the problems” that seems to work in both cases.
更多查看译文
关键词
Recognitions of concepts,Gestalt,Fidelity-classifiers
AI 理解论文
溯源树
样例
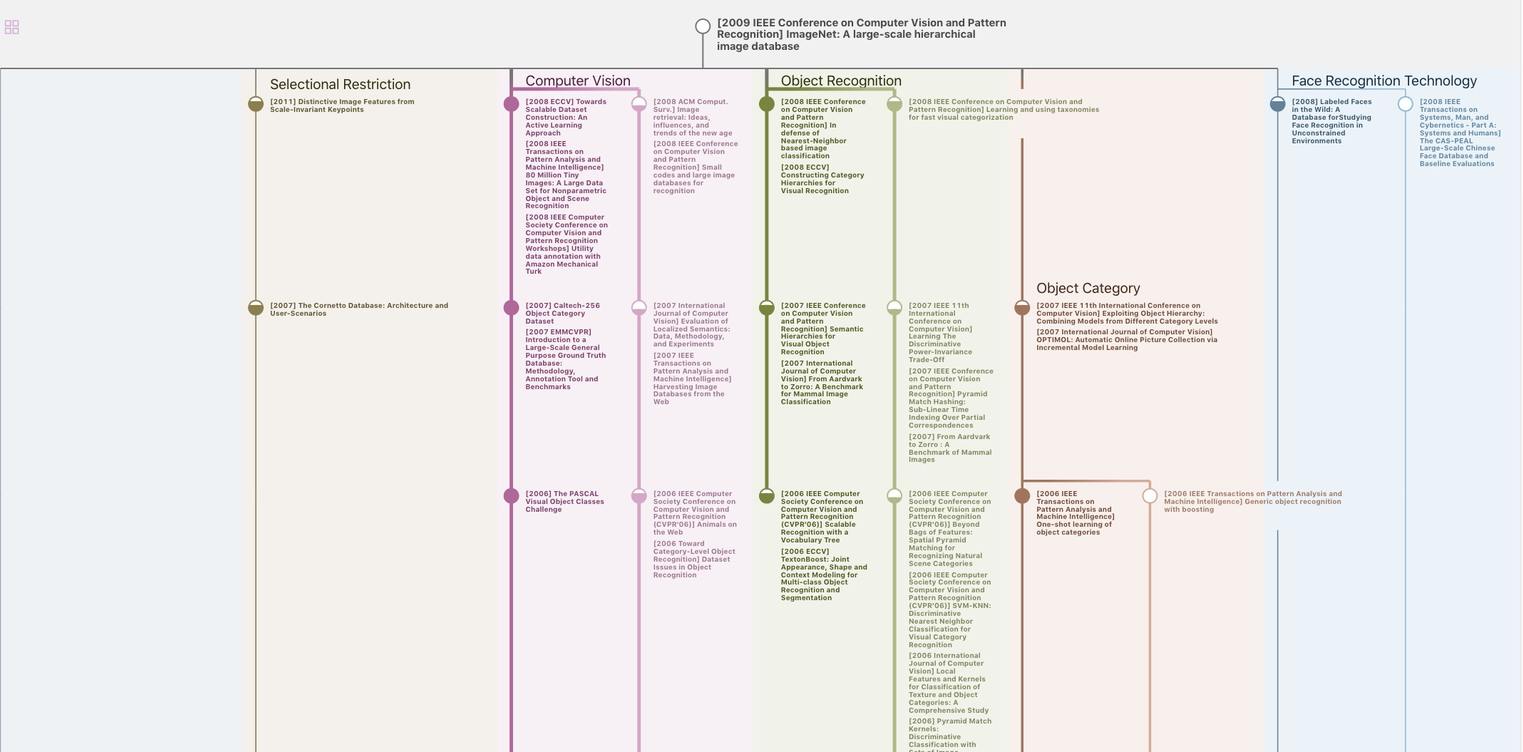
生成溯源树,研究论文发展脉络
Chat Paper
正在生成论文摘要