An innovative heterogeneous transfer learning framework to enhance the scalability of deep reinforcement learning controllers in buildings with integrated energy systems
Building Simulation(2024)
摘要
Deep Reinforcement Learning (DRL)-based control shows enhanced performance in the management of integrated energy systems when compared with Rule-Based Controllers (RBCs), but it still lacks scalability and generalisation due to the necessity of using tailored models for the training process. Transfer Learning (TL) is a potential solution to address this limitation. However, existing TL applications in building control have been mostly tested among buildings with similar features, not addressing the need to scale up advanced control in real-world scenarios with diverse energy systems. This paper assesses the performance of an online heterogeneous TL strategy, comparing it with RBC and offline and online DRL controllers in a simulation setup using EnergyPlus and Python. The study tests the transfer in both transductive and inductive settings of a DRL policy designed to manage a chiller coupled with a Thermal Energy Storage (TES). The control policy is pre-trained on a source building and transferred to various target buildings characterised by an integrated energy system including photovoltaic and battery energy storage systems, different building envelope features, occupancy schedule and boundary conditions (e.g., weather and price signal). The TL approach incorporates model slicing, imitation learning and fine-tuning to handle diverse state spaces and reward functions between source and target buildings. Results show that the proposed methodology leads to a reduction of 10
更多查看译文
关键词
transfer learning,reinforcement learning,building control,building energy management
AI 理解论文
溯源树
样例
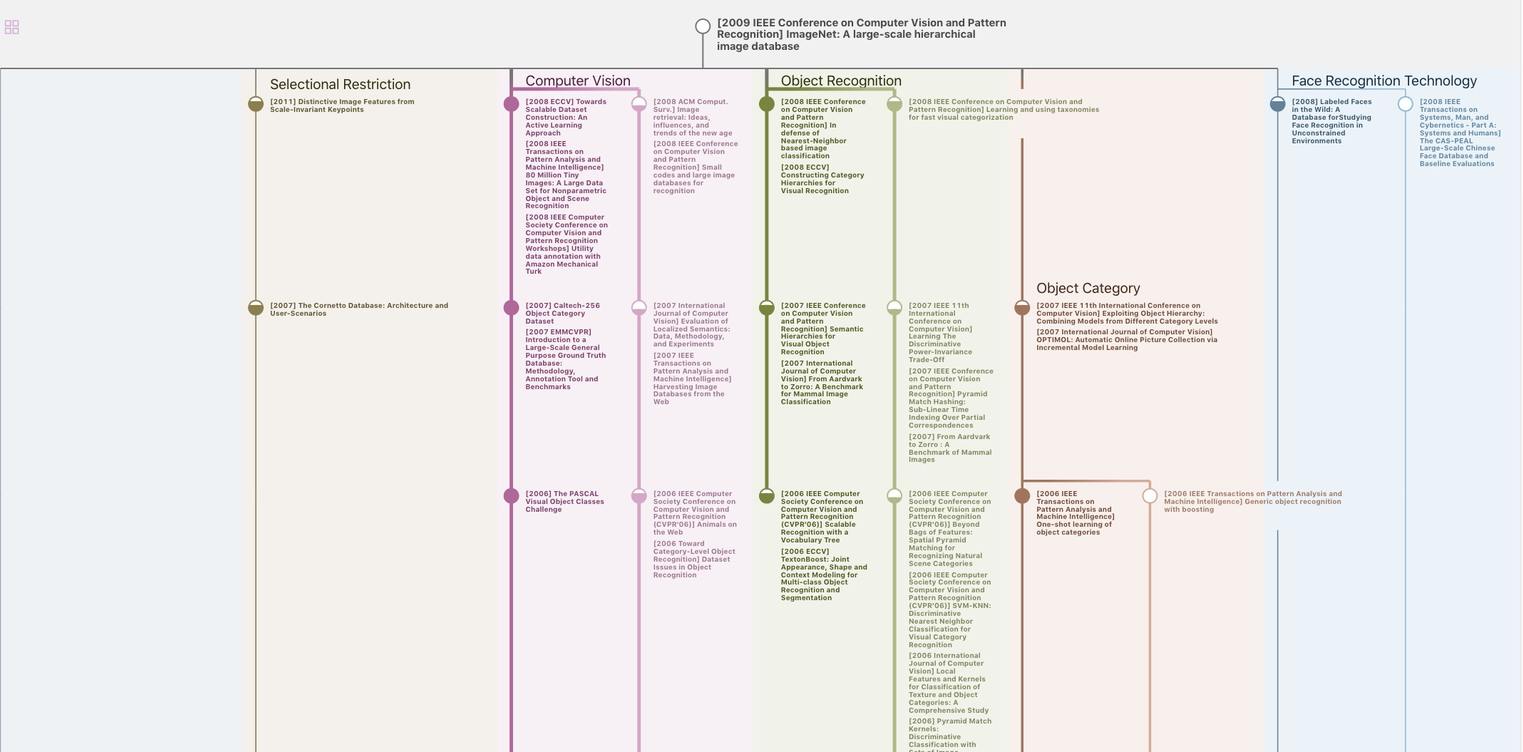
生成溯源树,研究论文发展脉络
Chat Paper
正在生成论文摘要