Seam Removal for Patch-Based Ultra-High-Resolution Stain Normalization.
IEEE International Conference on Bioinformatics and Bioengineering(2023)
摘要
Stain normalization is a key computational method in pathology that transforms histological stain images of one style to another. Modern methods are mostly based on neural image-to-image translation techniques. For very large image inputs, which are common in practice (e.g., whole slide images (WSIs)), the inferences are forced to run multiple times, each on a different subset of the image, due to GPU memory constraints. To minimize the differences between different outputs, several modifications [1], [2] of the standard instance-normalization (IN) layers have been proposed. Despite the reduced color variances, visible seams remain even with these approaches, which are disruptive to histologists when they closely examine the stitched results. These seams are also detrimental to the performance of some downstream tasks such as tumor classification. Hence, we propose a novel approach to effectively remove the seams by utilizing a Pix2Pix [3]-based neural network and an alpha blending-based post-processing step. Tested on real-world medical and natural image datasets, we found that our method performed much better than traditional Poisson image editing-based seam removal approaches. Our approaches qualitatively (in terms of the visibility of the seams) and quantitatively improved the results by prior stain normalization methods by large margins.
更多查看译文
关键词
Stain normalization,neural network,image-to-image translation
AI 理解论文
溯源树
样例
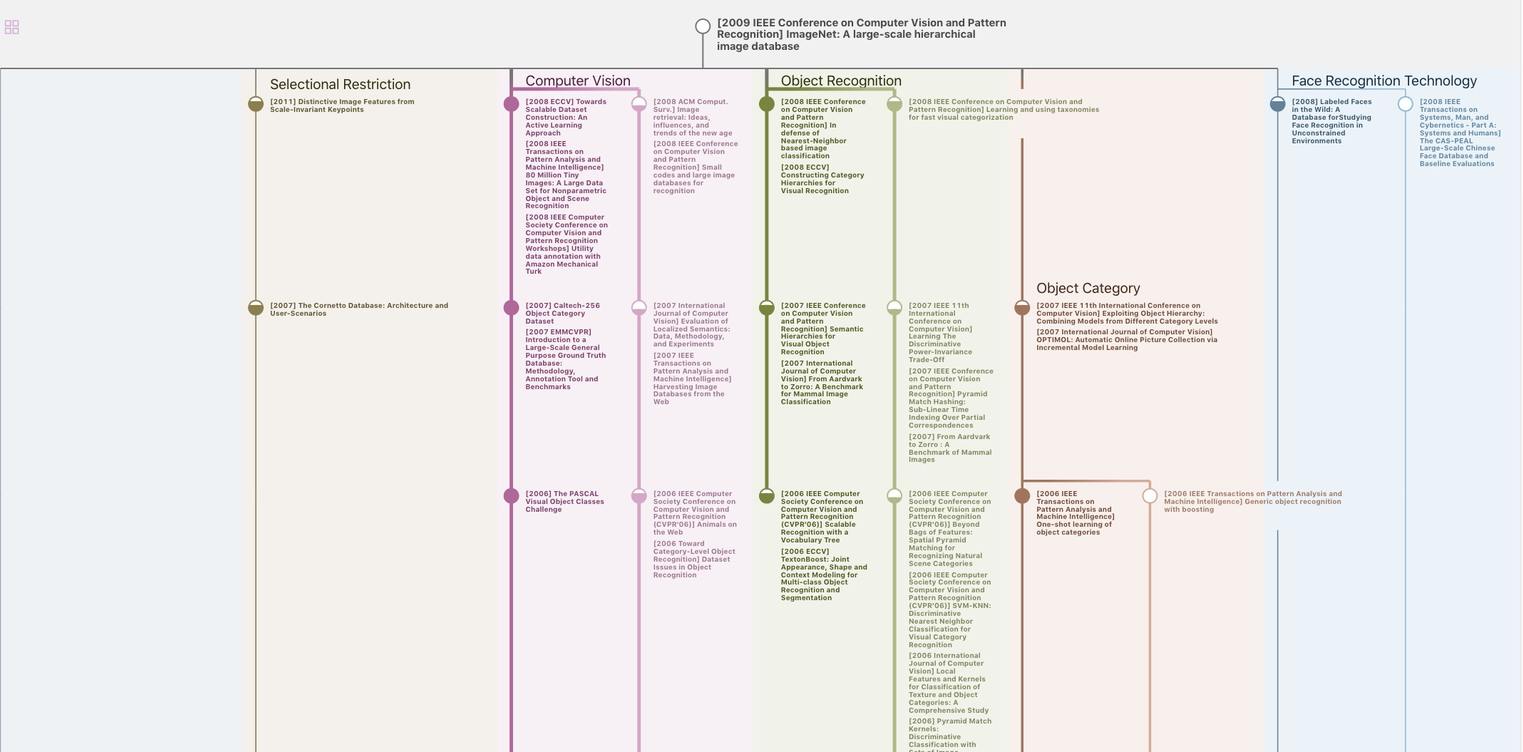
生成溯源树,研究论文发展脉络
Chat Paper
正在生成论文摘要