Ego Group Partition: A Novel Framework for Improving Ego Experiments in Social Networks
CoRR(2024)
摘要
Estimating the average treatment effect in social networks is challenging due
to individuals influencing each other. One approach to address interference is
ego cluster experiments, where each cluster consists of a central individual
(ego) and its peers (alters). Clusters are randomized, and only the effects on
egos are measured. In this work, we propose an improved framework for ego
cluster experiments called ego group partition (EGP), which directly generates
two groups and an ego sub-population instead of ego clusters. Under specific
model assumptions, we propose two ego group partition algorithms. Compared to
the original ego clustering algorithm, our algorithms produce more egos, yield
smaller biases, and support parallel computation. The performance of our
algorithms is validated through simulation and real-world case studies.
更多查看译文
AI 理解论文
溯源树
样例
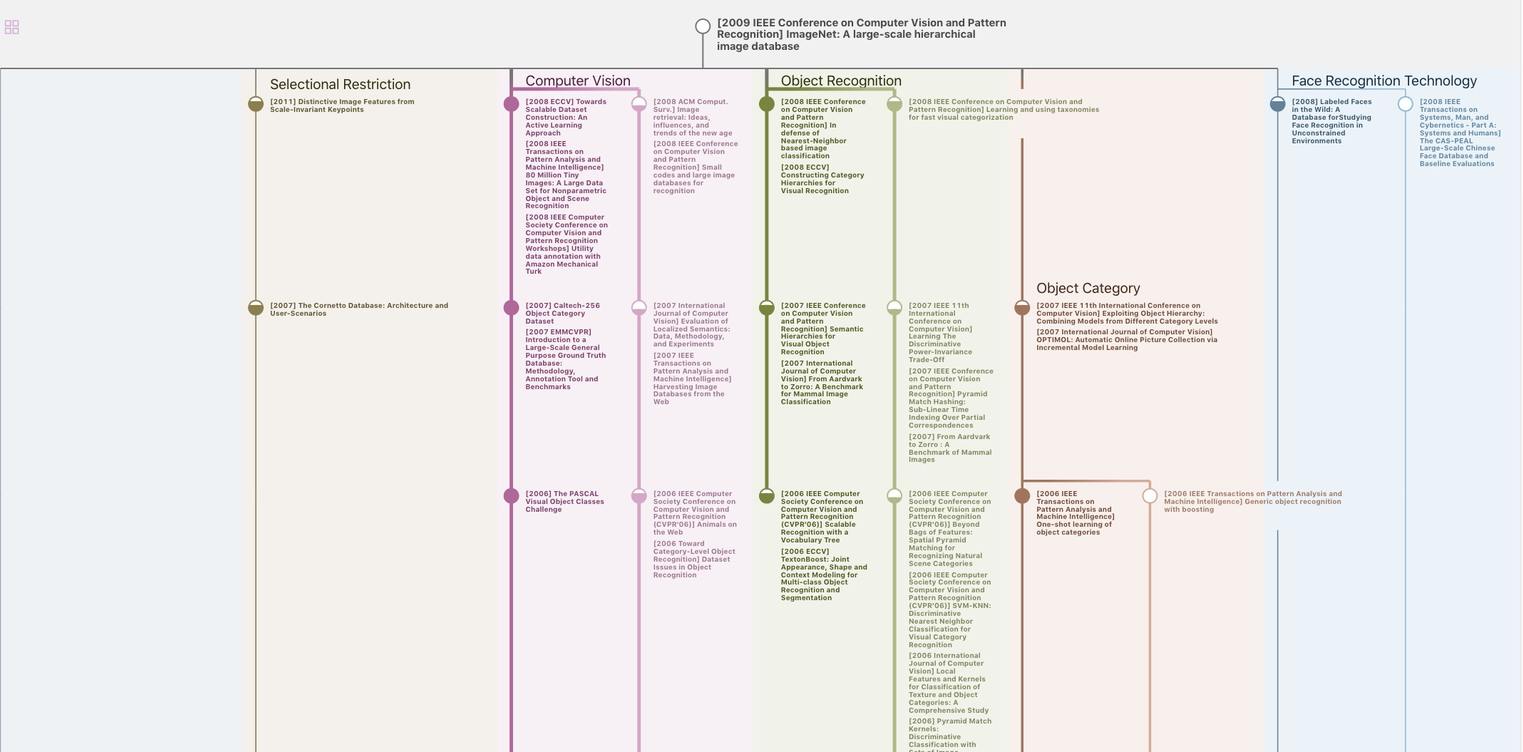
生成溯源树,研究论文发展脉络
Chat Paper
正在生成论文摘要