Controlled Variable Selection from Summary Statistics Only? A Solution via GhostKnockoffs and Penalized Regression.
ArXiv(2024)
摘要
Identifying which variables do influence a response while controlling false positives pervades statistics and data science. In this paper, we consider a scenario in which we only have access to summary statistics, such as the values of marginal empirical correlations between each dependent variable of potential interest and the response. This situation may arise due to privacy concerns, e.g., to avoid the release of sensitive genetic information. We extend GhostKnockoffs (He et al. [2022]) and introduce variable selection methods based on penalized regression achieving false discovery rate (FDR) control. We report empirical results in extensive simulation studies, demonstrating enhanced performance over previous work. We also apply our methods to genome-wide association studies of Alzheimer's disease, and evidence a significant improvement in power.
更多查看译文
AI 理解论文
溯源树
样例
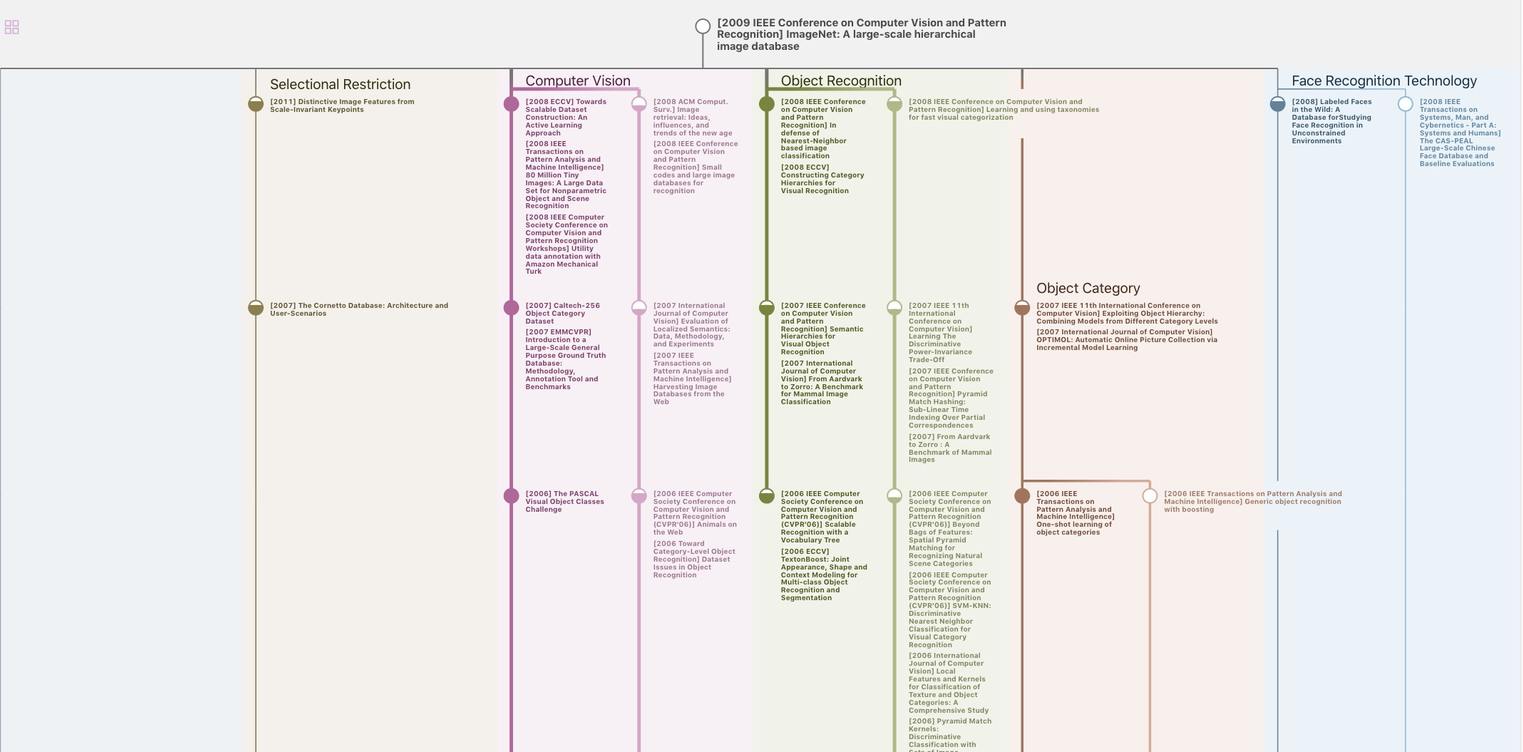
生成溯源树,研究论文发展脉络
Chat Paper
正在生成论文摘要