Unsupervised Detection of Seizure-Related Dynamic Alterations with Autoencoder-Derived Deep Features.
IEEE International Conference on Bioinformatics and Bioengineering(2023)
摘要
The detection of brain functional alterations related to Epilepsy is crucial in tackling this condition and administering effective patient care. In the last years, several methods have been proposed for accurate non-invasive temporal detection and characterization of seizures in epilepsy using electroencephalography (EEG) data as input. These methods usually follow machine or deep learning frameworks with the majority of the proposed solutions being supervised. In this study, we propose an unsupervised approach for seizure detection using deep learning-based autoencoder-derived features from EEG data. Our method employs deep learning techniques and Markov Chain modeling to automatically identify subtle alterations in high-frequency EEG temporal dynamics associated with seizures. The openly available CHB-MIT database was used for our evaluations. From the results, we demonstrate the potential of the proposed approach in detecting abnormal dynamic behaviors potentially associated with ictal events, achieving ROC-AUC scores in the range of 84-100
%
, without depending on extensive labeling efforts. The proposed pipeline potentially contributes towards the automation of detecting seizure onset in epilepsy.
更多查看译文
关键词
Epilepsy,Anomaly Detection,Deep Learning Unsupervised Learning,Autoencoders
AI 理解论文
溯源树
样例
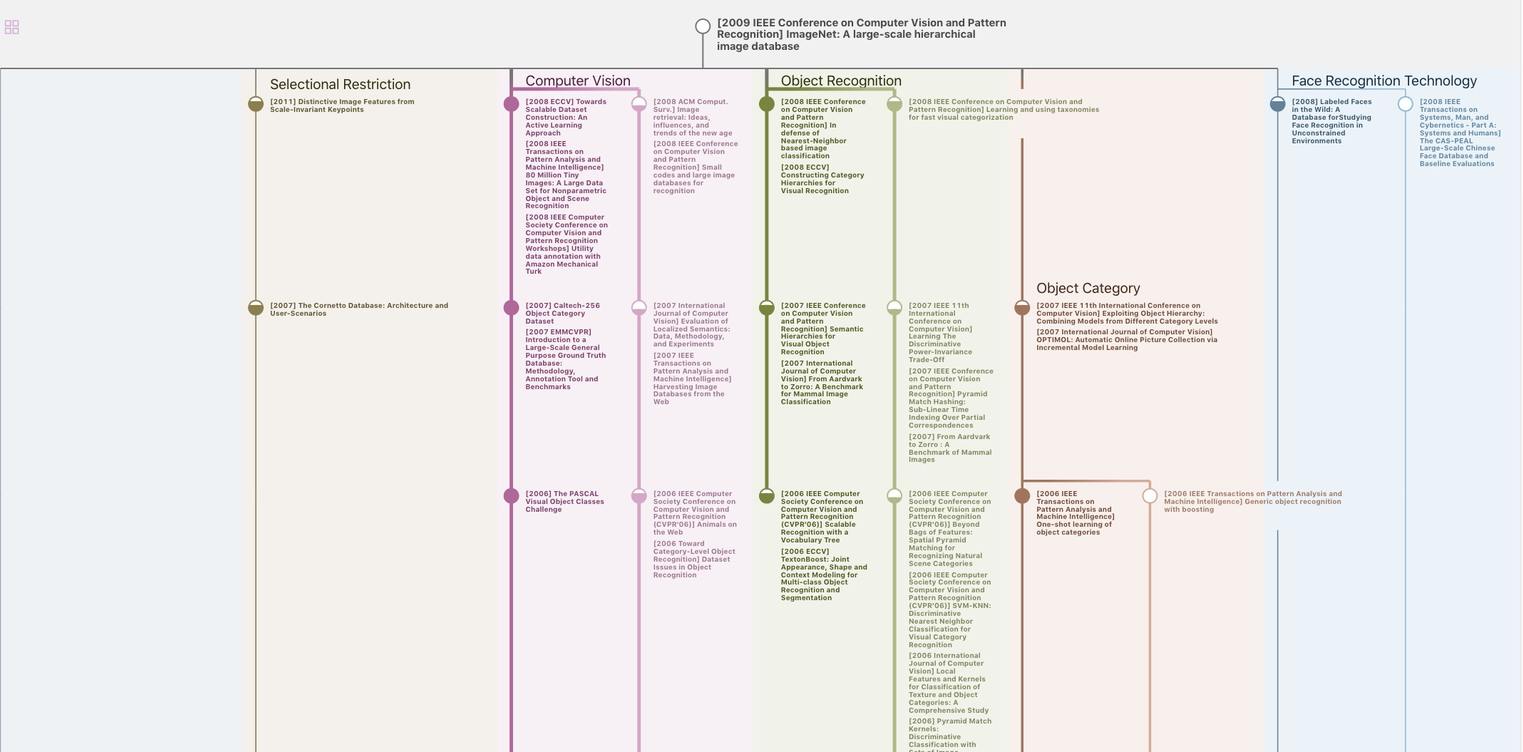
生成溯源树,研究论文发展脉络
Chat Paper
正在生成论文摘要