Assessing Wheat Yield and Grain Protein Content with Machine Learning and Satellite Hyperspectral Data: Multi-Year Explorative Analysis.
Workshop on Hyperspectral Image and Signal Processing(2023)
摘要
The estimation of yield and grain protein content (GPC) is crucial for agricultural management and decision-making processes. Traditional methods of measuring yield and GPC have limitations for large-scale monitoring. In recent years, remote sensing and machine learning (ML) techniques have emerged as powerful tools for yield and GPC estimation. This work aims to explore the potential of hyperspectral satellite remote sensing and ML techniques in estimating yield, GPC and their product (YGPC) for winter wheat. Several PRISMA hyperspectral images acquired during 2020, 2021 and 2022 were used as input of two different ML regression algorithms: Partial Least Square Regression and Gaussian Process Regression. Generally, good performances were obtained for yield and YGPC with nRMSE ranging from 13% to 17% whereas GPC assessment proved to be more challenging with nRMSE ranging from 22% to 24%. The best phenological phase for the estimation resulted in the period after the peak of production.
更多查看译文
关键词
Grain Protein Content,Yield,Hyperspectral,Machine Learning,Remote Sensing
AI 理解论文
溯源树
样例
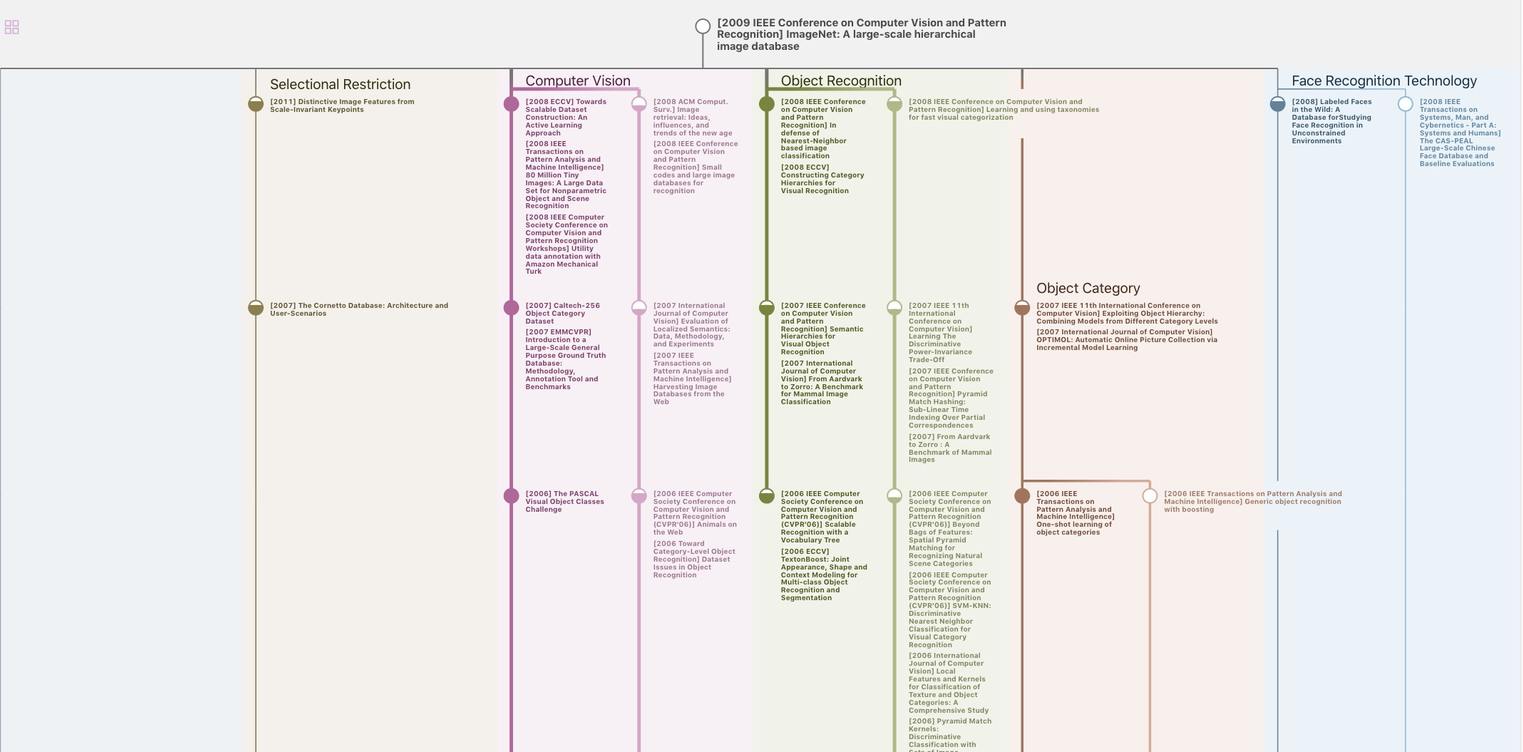
生成溯源树,研究论文发展脉络
Chat Paper
正在生成论文摘要