Identification of Idiopathic Pulmonary Fibrosis and Prediction of Disease Severity via Machine Learning Analysis of Comprehensive Metabolic Panel and Complete Blood Count Data
Lung(2024)
摘要
Diagnosis of idiopathic pulmonary fibrosis (IPF) typically relies on high-resolution computed tomography imaging (HRCT) or histopathology, while monitoring disease severity is done via frequent pulmonary function testing (PFT). More reliable and convenient methods of diagnosing fibrotic interstitial lung disease (ILD) type and monitoring severity would allow for early identification and enhance current therapeutic interventions. This study tested the hypothesis that a machine learning (ML) ensemble analysis of comprehensive metabolic panel (CMP) and complete blood count (CBC) data can accurately distinguish IPF from connective tissue disease ILD (CTD-ILD) and predict disease severity as seen with PFT. Outpatient data with diagnosis of IPF or CTD-ILD (n = 103 visits by 53 patients) were analyzed via ML methodology to evaluate (1) IPF vs CTD-ILD diagnosis; (2)
更多查看译文
关键词
Interstitial lung disease,Idiopathic pulmonary fibrosis,Connective tissue disease,Machine learning,Pulmonary function testing
AI 理解论文
溯源树
样例
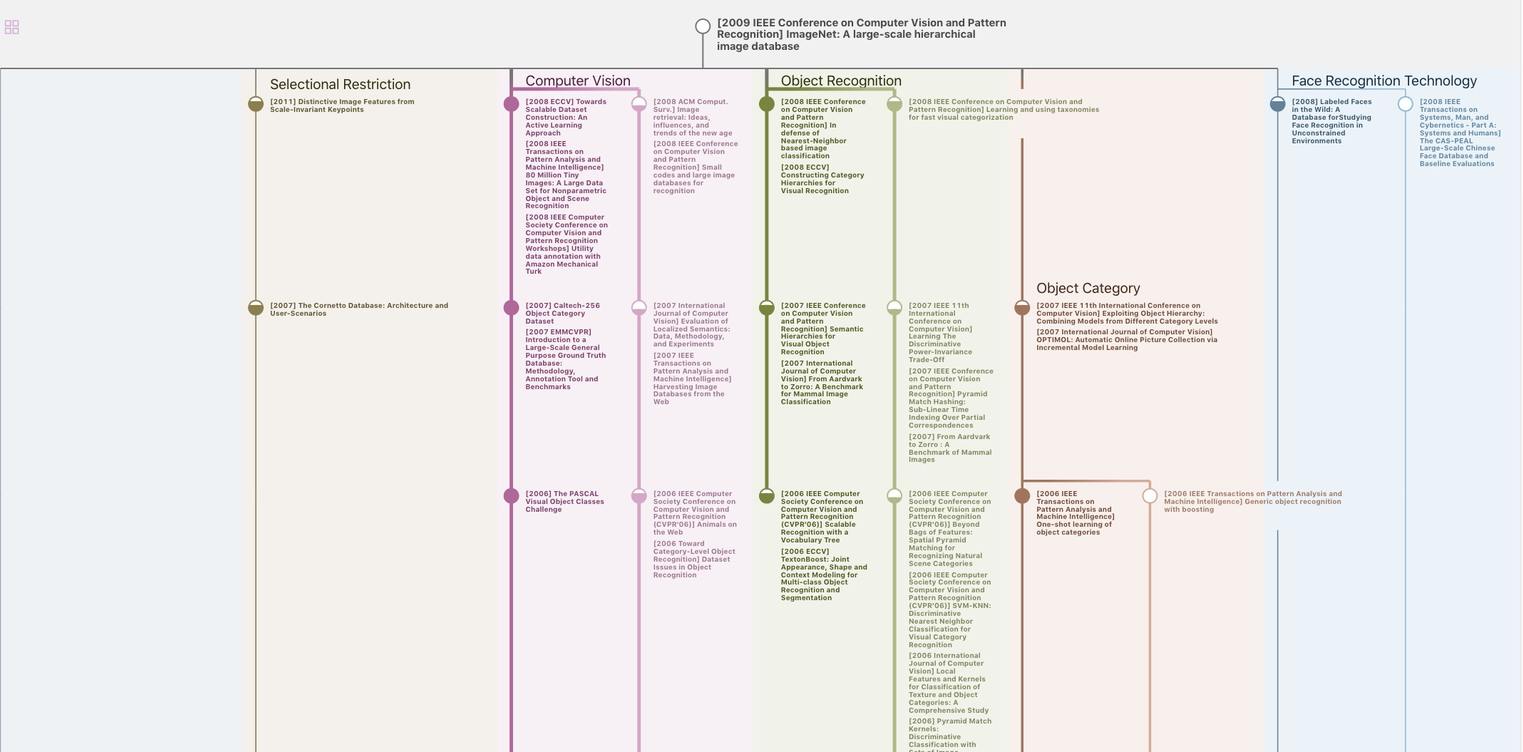
生成溯源树,研究论文发展脉络
Chat Paper
正在生成论文摘要