A Novel Data Augmentation Approach Using Mask Encoding for Deep Learning-Based Asynchronous SSVEP-BCI
IEEE TRANSACTIONS ON NEURAL SYSTEMS AND REHABILITATION ENGINEERING(2024)
摘要
Deep learning (DL)-based methods have been successfully employed as asynchronous classification algorithms in the steady-state visual evoked potential (SSVEP)-based brain-computer interface (BCI) system. However, these methods often suffer from the limited amount of electroencephalography (EEG) data, leading to overfitting. This study proposes an effective data augmentation approach called EEG mask encoding (EEG-ME) to mitigate overfitting. EEG-ME forces models to learn more robust features by masking partial EEG data, leading to enhanced generalization capabilities of models. Three different network architectures, including an architecture integrating convolutional neural networks (CNN) with Transformer (CNN-Former), time domain-based CNN (tCNN), and a lightweight architecture (EEGNet) are utilized to validate the effectiveness of EEG-ME on publicly available benchmark and BETA datasets. The results demonstrate that EEG-ME significantly enhances the average classification accuracy of various DL-based methods with different data lengths of time windows on two public datasets. Specifically, CNN-Former, tCNN, and EEGNet achieve respective improvements of 3.18%, 1.42%, and 3.06% on the benchmark dataset as well as 11.09%, 3.12%, and 2.81% on the BETA dataset, with the 1-second time window as an example. The enhanced performance of SSVEP classification with EEG-ME promotes the implementation of the asynchronous SSVEP-BCI system, leading to improved robustness and flexibility in human-machine interaction.
更多查看译文
关键词
Asynchronous brain-computer interface,data augmentation,deep learning,electroencephalography mask encoding,steady-state visual evoked potential
AI 理解论文
溯源树
样例
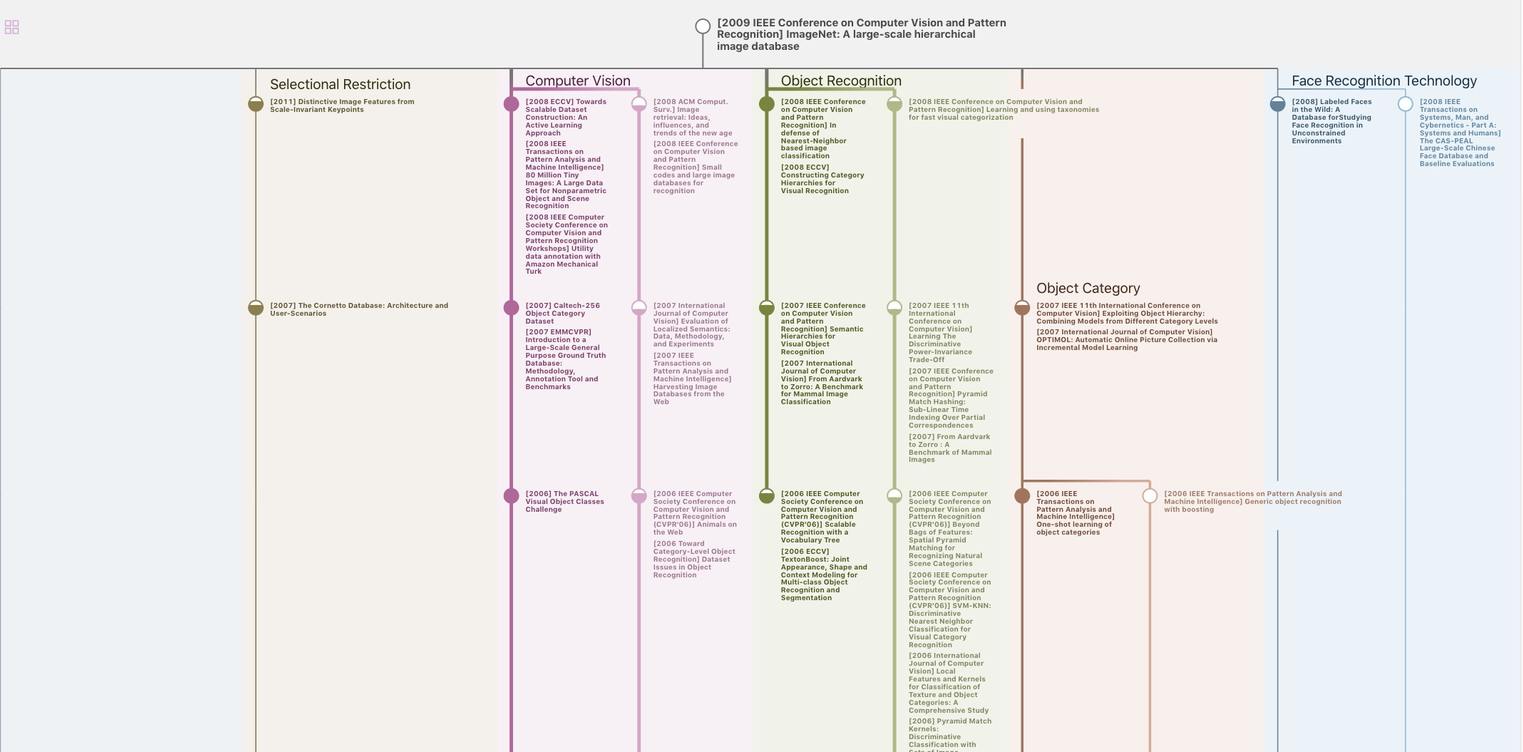
生成溯源树,研究论文发展脉络
Chat Paper
正在生成论文摘要